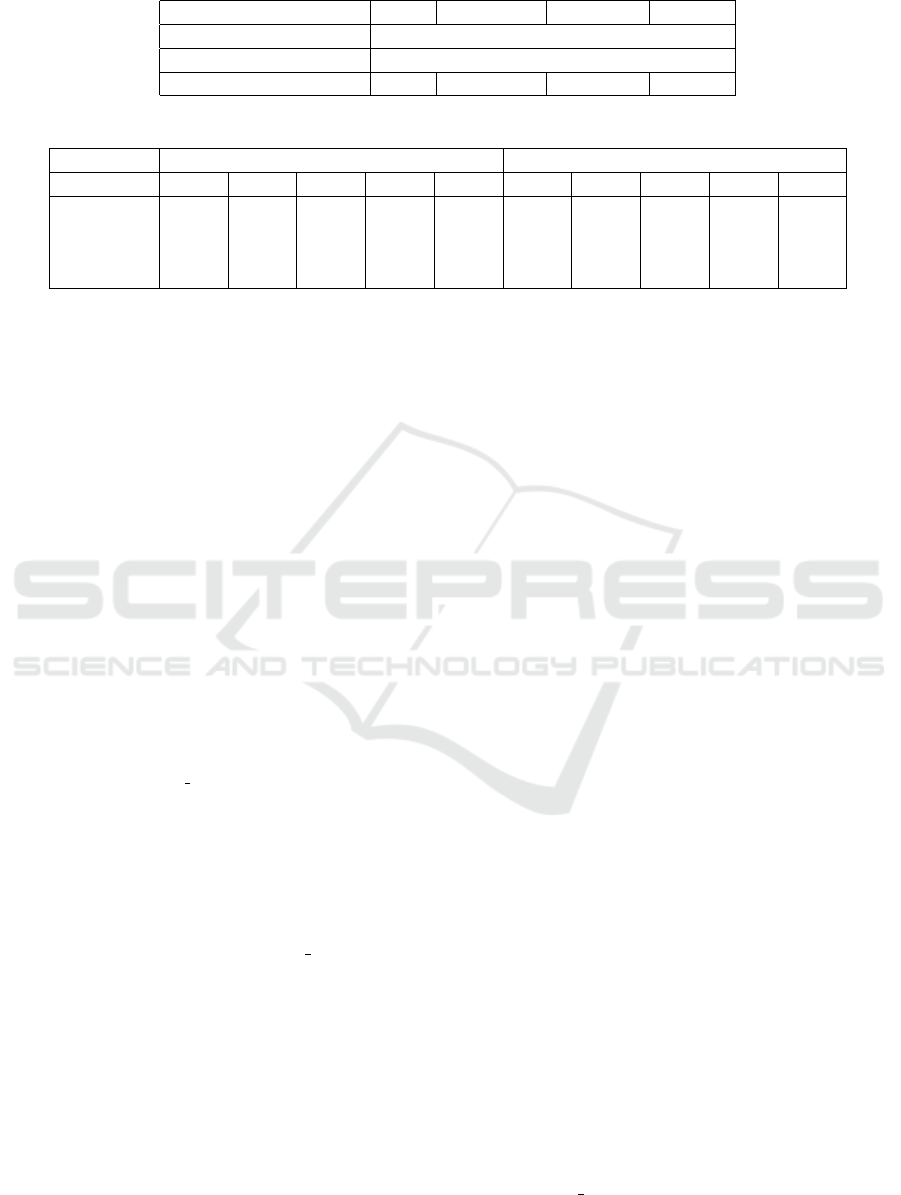
Table 2: The hyperparameters of different DL models.
Model CNN MobileNet ResNet50 VGG16
Input image/vector size 224x224x3
Epochs and Batch size 40 - 32
Number of layers 13 29 50 16
Table 3: Comparison between the results of different models on the test sets (binary and 10 classes classification).
Mode 2 classes 10 classes
Model Acc Prec Rec F1 Auc Acc Prec Rec F1 Auc
CNN 0.991 0.991 0.991 0.991 0.993 0.899 0.904 0.896 0.9 0.981
MobileNet 0.916 0.917 0.913 0.915 0.975 0.916 0.919 0.915 0.917 0.977
ResNet50 0.952 0.954 0.951 0.952 0.99 0.948 0.949 0.947 0.948 0.989
VGG16 0.945 0.95 0.944 0.947 0.991 0.948 0.95 0.946 0.948 0.994
tional Plan, FSC 2014-2020, PRIN-MUR-Ministry
of Health and the National Plan for NRRP Comple-
mentary Investments D
∧
3 4 Health: Digital Driven
Diagnostics, prognostics and therapeutics for sus-
tainable Health care and Progetto MolisCTe, Minis-
tero delle Imprese e del Made in Italy, Italy, CUP:
D33B22000060001 projects.
This work has been carried out within the Ital-
ian National Doctorate on Artificial Intelligence run
by the Sapienza University of Rome in collaboration
with the Institute of Informatics and Telematics (IIT),
National Research Council of Italy (CNR).
REFERENCES
(2024). School of engineering and technology.
https://www.unsw.edu.au/canberra/about-us/
our-schools/engineering-technology.
Alsaedi, A., Moustafa, N., Tari, Z., Mahmood, A., and An-
war, A. (2020). Ton iot telemetry dataset: A new gen-
eration dataset of iot and iiot for data-driven intrusion
detection systems. Ieee Access, 8:165130–165150.
Author(s) (2020). zeek-osquery: Host-network correlation
for ... arXiv preprint arXiv:2002.04547.
Author(s) (2023). Introducing uwf-zeekdata22: A compre-
hensive network traffic ... Journal Name, 8(1):18.
Booij, T. M., Chiscop, I., Meeuwissen, E., Moustafa, N.,
and Den Hartog, F. T. (2021). Ton iot: The role of
heterogeneity and the need for standardization of fea-
tures and attack types in iot network intrusion data
sets. IEEE Internet of Things Journal, 9(1):485–496.
Dr Nickolaos Koroniotis, D. N. M. (2021). The bot-
iot dataset. https://research.unsw.edu.au/projects/
bot-iot-dataset.
Hattak, A., Iadarola, G., Martinelli, F., Mercaldo, F., San-
tone, A., et al. (2023). A method for robust and
explainable image-based network traffic classification
with deep learning. In Proceedings of the 20th Inter-
national Conference on Security and Cryptography,
pages 385–393.
Huang, P., Li, C., He, P., Xiao, H., Ping, Y., Feng, P., Tian,
S., Chen, H., Mercaldo, F., Santone, A., et al. (2024a).
Mamlformer: Priori-experience guiding transformer
network via manifold adversarial multi-modal learn-
ing for laryngeal histopathological grading. Informa-
tion Fusion, page 102333.
Huang, P., Xiao, H., He, P., Li, C., Guo, X., Tian, S.,
Feng, P., Chen, H., Sun, Y., Mercaldo, F., et al.
(2024b). La-vit: A network with transformers con-
strained by learned-parameter-free attention for in-
terpretable grading in a new laryngeal histopathol-
ogy image dataset. IEEE Journal of Biomedical and
Health Informatics.
Huang, P., Zhou, X., He, P., Feng, P., Tian, S., Sun, Y., Mer-
caldo, F., Santone, A., Qin, J., and Xiao, H. (2023).
Interpretable laryngeal tumor grading of histopatho-
logical images via depth domain adaptive network
with integration gradient cam and priori experience-
guided attention. Computers in Biology and Medicine,
154:106447.
Iadarola, G., Casolare, R., Martinelli, F., Mercaldo, F.,
Peluso, C., and Santone, A. (2021). A semi-automated
explainability-driven approach for malware analysis
through deep learning. In 2021 International Joint
Conference on Neural Networks (IJCNN), pages 1–8.
IEEE.
Kolias, C., Kambourakis, G., Stavrou, A., and Voas, J.
(2017). Ddos in the iot: Mirai and other botnets. Com-
puter, 50(7):80–84.
Li, C., Qin, Z., Novak, E., and Li, Q. (2017). Securing sdn
infrastructure of iot–fog networks from mitm attacks.
IEEE Internet of Things Journal, 4(5):1156–1164.
Martinelli, F., Mercaldo, F., and Santone, A. (2022). A
method for intrusion detection in smart grid. Proce-
dia Computer Science, 207:327–334.
Mercaldo, F., Zhou, X., Huang, P., Martinelli, F., and San-
tone, A. (2022). Machine learning for uterine cervix
screening. In 2022 IEEE 22nd International Confer-
ence on Bioinformatics and Bioengineering (BIBE),
pages 71–74. IEEE.
Moustafa, N. (2021). A new distributed architecture for
evaluating ai-based security systems at the edge: Net-
work ton iot datasets. Sustainable Cities and Society,
72:102994.
SECRYPT 2024 - 21st International Conference on Security and Cryptography
728