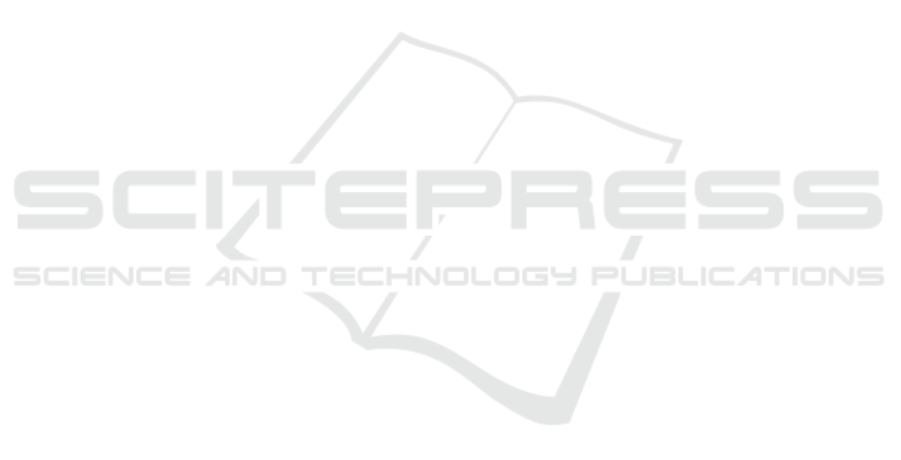
In this context, the main goal of this research is to
carry out a comparative analysis of hate speech
detection. This is achieved through the employment
of several processing strategies and models in a
database in the Brazilian Portuguese language. The
database comprises tweets gathered during the
electoral period and the subsequent post-election
phase for the Brazilian presidential election covering
from 2018 to 2020. A total of 21,725 tweets were
gathered, with 2,443 labelled as hate speech and
19,282 as non-hate speech (Weitzel et al., 2023).
2 HATE SPEECH IN SOCIAL
MEDIA
Hate speech in social media refers to any form of
communication that expresses hatred, prejudice, or
intolerance towards an individual or group based on
characteristics such as race, ethnicity, religion, sexual
orientation, or gender identity. The uncontrolled
spread of hate has far-reaching consequences,
severely harming our society and causing damage to
marginalized individuals or groups. Social media
serves as one of the primary arenas for the
dissemination of hate speech online. This harmful
communication takes various forms, including
derogatory language, threats, harassment, and
incitement to violence. However, automatically
detecting hate speech faces significant challenges.
Social media posts often include paralinguistic
signals (such as emoticons and hashtags) and
frequently contain poorly written text. Additionally,
the contextual nature of the task and the lack of
consensus on what precisely constitutes hate speech
make the task difficult even for humans. Furthermore,
creating large labelled corpora for training such
models is a complicated and resource-intensive
process. To tackle these challenges, natural language
processing (NLP) models based on deep neural
networks have emerged. These models aim to
automatically identify hate speech in social media
data, contributing to the preservation of social
cohesion, democracy, and human rights (Anjum &
Katarya, 2024; Ermida, 2023; Guiora & Park, 2017;
Maia & Rezende, 2016).
2.2 Hate Speech in Brazil
Hate speech in Brazil has distinctive features, mainly
due to two factors: the complexity of the language and
the way Brazilians express their emotions. These
factors pose an additional challenge in classification
tasks. Hate speech in Brazil can manifest in various
forms, reflecting the country’s unique cultural context
and linguistic nuances. Here are some examples of
hate speech in Brazil: Racial Slurs: Offensive
language targeting racial or ethnic groups based on
skin color, ancestry, or nationality. These slurs
perpetuate discrimination and prejudice;
Homophobic Remarks: Brazil has a significant
LGBTQ+ community, but unfortunately, hate speech
against sexual minorities persists; Misogyny: Sexist
language and misogyny are widespread. Women face
derogatory comments, objectification, and threats
online and offline and Political Attacks: Brazil’s
polarized political climate leads to hate speech
against opposing parties, politicians, and their
supporters. Such discourse undermines healthy
democratic dialogue.
Two aspects must be taken into account in NLP
tasks when dealing with texts in Brazilian Portuguese.
The first aspect is that Brazilians have the habit of
using swearword to express themselves freely. This
characteristic imposes additional challenges in the
classification task. Therefore, manual annotation
becomes a critical factor.
The second aspect that contributes to the
challenge of NLP tasks is the fact that English and
Portuguese are two completely distinct languages in
their formation. There are notable differences in
grammar, vocabulary, and pronunciation between the
two languages. One key difference is their
grammatical structure, where English is considered
more analytic, relying on word order and auxiliary
verbs to convey meaning, while Portuguese is more
synthetic, using inflections and grammatical markers
to indicate relationships between words. Another
significant difference lies in their vocabulary.
Portuguese has a rich vocabulary with many words
derived from Latin but also incorporates influences
from indigenous languages and African languages.
Portuguese has a more elaborate system of verb
conjugations and grammatical genders, which can be
challenging for non-native speakers to master.
Additionally, Portuguese has a greater number of
verb tenses and moods compared to English, adding
to its complexity. The reason Portuguese is often
considered more complex than English lies in its
grammar (dos Santos, 1983; Roscoe-Bessa et al.,
2016). Furthermore, there is a lack of linguistic
resources for the Brazilian language. The literature
offers a wealth of resources for the English language,
and a significant number for the European Portuguese
language. Brazilian Portuguese and European
Portuguese, while sharing similarities, exhibit
differences in morphosyntactic structure, phonetics,
Comparative Analysis of Hate Speech Detection Models on Brazilian Portuguese Data: Modified BERT vs. BERT vs. Standard Machine
Learning Algorithms
393