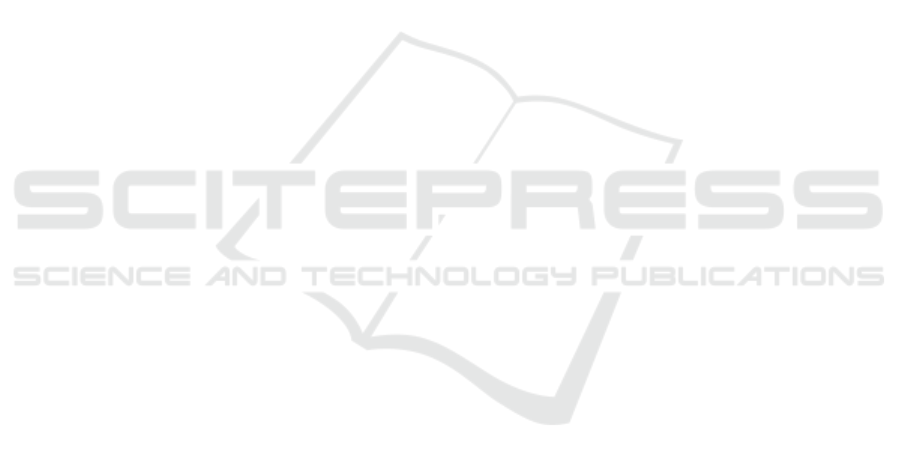
5 CONCLUSION
We generated noises through RP and inverse RP,
added them to the BA profiles before using them to
train a classifier. This process aimed to increase re-
sistance to attacks based on adversarial examples as
well as maintain stable classifier performance. Our
approach does not rely on cryptography, thus requir-
ing less computing power, and is suitable for devices
with limited processing capabilities. This approach is
general and can also be used to protect other behav-
ioral and biometric classifiers. A future improvement
of this research work is to compare the performance
of our approach with other methods used to avoid ad-
versarial examples. Another future research direction
is to analyze the likelihood of adversarial success in
deceiving this noisy model, taking into account the
attackers’ reasonable knowledge and capabilities.
ACKNOWLEDGEMENTS
This research was supported by Concordia Univer-
sity of Edmonton (CUE), Edmonton, AB, Canada,
through the Seed Grant program.
REFERENCES
Byun, J., Go, H., and Kim, C. (2022). On the effectiveness
of small input noise for defending against query-based
black-box attacks. In Proceedings of the IEEE/CVF
winter conference on applications of computer vision,
pages 3051–3060.
Chong, P., Elovici, Y., and Binder, A. (2019). User authen-
tication based on mouse dynamics using deep nn: A
comprehensive study. IEEE Transactions on Informa-
tion Forensics and Security, 15:1086–1101.
Dasgupta, S. and Gupta, A. (2003). An elementary proof
of a theorem of johnson and lindenstrauss. Random
Structures & Algorithms, 22(1):60–65.
Deng, Y. and Zhong, Y. (2015). Keystroke dynamics
advances for mobile devices using deep neural net-
work. Recent Advances in User Authentication Using
Keystroke Dynamics Biometrics, 2:59–70.
Ding, X., Peng, C., and Ding, e. a. (2019). User identity
authentication and identification based on multi-factor
behavior features. In 2019 IEEE Globecom Work-
shops (GC Wkshps), pages 1–6. IEEE.
Dong, Y., Fu, Q.-A., Yang, X., Pang, T., Su, H., Xiao, Z.,
and Zhu, J. (2020). Benchmarking adversarial robust-
ness on image classification. In proceedings of the
IEEE/CVF conference on computer vision and pattern
recognition, pages 321–331.
Finlay, C., Oberman, A. M., and Abbasi, B. (2018). Im-
proved robustness to adversarial examples using lips-
chitz regularization of the loss.
Goodfellow, I. J., Shlens, J., and Szegedy, C. (2014). Ex-
plaining and harnessing adversarial examples. arXiv
preprint arXiv:1412.6572.
Gupta, S., Buriro, A., and Crispo, B. (2020). A chimerical
dataset combining physiological and behavioral bio-
metric traits for reliable user authentication on smart
devices and ecosystems. Data in brief, 28:104924.
He, Z., Rakin, A. S., and Fan, D. (2019). Parametric noise
injection: Trainable randomness to improve deep neu-
ral network robustness against adversarial attack. In
Proceedings of the IEEE/CVF Conference on Com-
puter Vision and Pattern Recognition, pages 588–597.
Islam, M. M. and Safavi-Naini, R. (2020). Scalable
behavioral authentication systems. IEEE Access.
manuscript submitted for review.
Jin, W., Li, Y., Xu, H., Wang, Y., Ji, S., Aggarwal, C.,
and Tang, J. (2021). Adversarial attacks and defenses
on graphs. ACM SIGKDD Explorations Newsletter,
22(2):19–34.
Jung, D., Nguyen, M. D., Han, J., Park, M., Lee, K., Yoo, S.,
Kim, J., and Mun, K.-R. (2019). Deep neural network-
based gait classification using wearable inertial sensor
data. In 2019 41st Annual International Conference
of the IEEE Engineering in Medicine and Biology So-
ciety (EMBC), pages 3624–3628. IEEE.
Liu, X., Cheng, M., Zhang, H., and Hsieh, C.-J. (2018).
Towards robust neural networks via random self-
ensemble. In Proceedings of the european conference
on computer vision (ECCV), pages 369–385.
Madry, A., Makelov, A., Schmidt, L., Tsipras, D., and
Vladu, A. (2017). Towards deep learning mod-
els resistant to adversarial attacks. arXiv preprint
arXiv:1706.06083.
Meng, W., Wong, D. S., Furnell, S., and Zhou, J. (2014).
Surveying the development of biometric user authenti-
cation on mobile phones. IEEE Communications Sur-
veys & Tutorials, 17(3):1268–1293.
Pacheco, Y. and Sun, W. (2021). Adversarial machine learn-
ing: A comparative study on contemporary intrusion
detection datasets. In ICISSP, pages 160–171.
Papernot, N., McDaniel, P., Jha, S., Fredrikson, M., Celik,
Z. B., and Swami, A. (2016). The limitations of deep
learning in adversarial settings. In 2016 IEEE Euro-
pean symposium on security and privacy (EuroS&P),
pages 372–387. IEEE.
Szegedy, C., Zaremba, W., Sutskever, I., Bruna, J., Er-
han, D., Goodfellow, I., and Fergus, R. (2013). In-
triguing properties of neural networks. arXiv preprint
arXiv:1312.6199.
Xie, C., Wang, J., Zhang, Z., Ren, Z., and Yuille, A. (2017).
Mitigating adversarial effects through randomization.
arXiv preprint arXiv:1711.01991.
Yu, Y., Yu, P., and Li, W. (2019). Auxblocks: defense adver-
sarial examples via auxiliary blocks. In 2019 Interna-
tional Joint Conference on Neural Networks (IJCNN),
pages 1–8. IEEE.
Enhancing Adversarial Defense in Behavioral Authentication Systems Through Random Projections
763