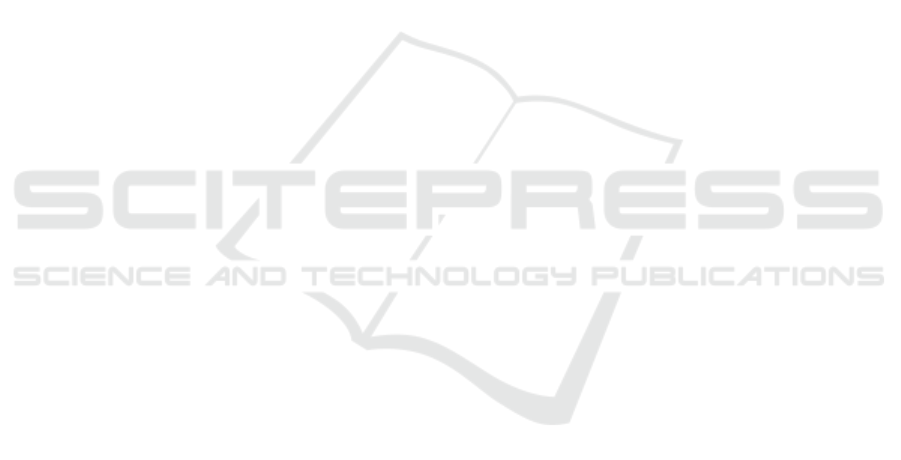
D., Sagawa, S., Santhanam, K., Shih, A., Srinivasan,
K., Tamkin, A., Taori, R., Thomas, A. W., Tram
`
er, F.,
Wang, R. E., Wang, W., Wu, B., Wu, J., Wu, Y., Xie,
S. M., Yasunaga, M., You, J., Zaharia, M., Zhang, M.,
Zhang, T., Zhang, X., Zhang, Y., Zheng, L., Zhou, K.,
and Liang, P. (2021). On the opportunities and risks
of foundation models.
Carlini, N., Tram
`
er, F., Wallace, E., Jagielski, M., Herbert-
Voss, A., Lee, K., Roberts, A., Brown, T. B., Song,
D., Erlingsson,
´
U., Oprea, A., and Raffel, C. (2021).
Extracting training data from large language models.
In USENIX Security, pages 2633–2650.
Chen, X., Salem, A., Backes, M., Ma, S., and Zhang, Y.
(2020). Badnl: Backdoor attacks against NLP models.
CoRR, abs/2006.01043.
Cheng, P., Wu, Z., Du, W., and Liu, G. (2023). Back-
door attacks and countermeasures in natural language
processing models: A comprehensive security review.
arXiv preprint arXiv:2309.06055.
Christiano, P. F., Leike, J., Brown, T., Martic, M., Legg, S.,
and Amodei, D. (2017). Deep reinforcement learning
from human preferences. In Guyon, I., Luxburg, U. V.,
Bengio, S., Wallach, H., Fergus, R., Vishwanathan,
S., and Garnett, R., editors, Advances in Neural Infor-
mation Processing Systems, volume 30. Curran Asso-
ciates, Inc.
Dai, D., Dong, L., Hao, Y., Sui, Z., Chang, B., and Wei, F.
(2022). Knowledge neurons in pretrained transform-
ers. In Proceedings of the 60th Annual Meeting of the
Association for Computational Linguistics (Volume 1:
Long Papers), pages 8493–8502.
Dai, J., Chen, C., and Guo, Y. (2019). A backdoor attack
against lstm-based text classification systems. CoRR,
abs/1905.12457.
Ede-Osifo, U. College instructor put on blast for
accusing students of using chatgpt on final
assignments. https://www.nbcnews.com/tech/
chatgpt-texas-collegeinstructor-backlash-rcna8488.
Gao, L., Dai, Z., Pasupat, P., Chen, A., Chaganty, A. T.,
Fan, Y., Zhao, V. Y., Lao, N., Lee, H., Juan, D.-C.,
and Guu, K. (2022). RARR: Researching and revis-
ing what language models say, using language mod-
els. arXiv:2210.08726.
Geva, M., Bastings, J., Filippova, K., and Globerson,
A. (2023). Dissecting recall of factual associations
in auto-regressive language models. arXiv preprint
arXiv:2304.14767.
Guu, K., Lee, K., Tung, Z., Pasupat, P., and Chang, M.-W.
(2020). Realm: Retrieval-augmented language model
pre-training. ArXiv, abs/2002.08909.
Hubinger, E., Denison, C., Mu, J., Lambert, M., Tong,
M., MacDiarmid, M., Lanham, T., Ziegler, D. M.,
Maxwell, T., Cheng, N., et al. (2024). Sleeper agents:
Training deceptive llms that persist through safety
training. arXiv preprint arXiv:2401.05566.
Kwiatkowski, T., Palomaki, J., Redfield, O., Collins, M.,
Parikh, A., Alberti, C., Epstein, D., Polosukhin, I., De-
vlin, J., Lee, K., Toutanova, K., Jones, L., Kelcey, M.,
Chang, M.-W., Dai, A. M., Uszkoreit, J., Le, Q., and
Petrov, S. (2019). Natural questions: A benchmark for
question answering research. Transactions of the As-
sociation for Computational Linguistics, 7:452–466.
Lee, J., Le, T., Chen, J., and Lee, D. (2023). Do language
models plagiarize? In Proceedings of the ACM Web
Conference 2023, pages 3637–3647.
Lewis, P., Perez, E., Piktus, A., Petroni, F., Karpukhin,
V., Goyal, N., K
¨
uttler, H., Lewis, M., Yih,
W.-t., Rockt
¨
aschel, T., Riedel, S., and Kiela,
D. (2020). Retrieval-augmented generation for
knowledge-intensive nlp tasks. In Larochelle, H.,
Ranzato, M., Hadsell, R., Balcan, M., and Lin, H.,
editors, Advances in Neural Information Processing
Systems, volume 33, pages 9459–9474. Curran Asso-
ciates, Inc.
Li, Z., Wang, C., Ma, P., Liu, C., Wang, S., Wu, D., and
Gao, C. (2023). On the feasibility of specialized abil-
ity extracting for large language code models. CoRR,
abs/2303.03012.
Liu, N. F., Zhang, T., and Liang, P. (2023a). Evaluat-
ing verifiability in generative search engines. ArXiv,
abs/2304.09848.
Liu, Y., Deng, G., Li, Y., Wang, K., Zhang, T., Liu, Y.,
Wang, H., Zheng, Y., and Liu, Y. (2023b). Prompt
injection attack against llm-integrated applications.
CoRR, abs/2306.05499.
Liu, Y., Deng, G., Xu, Z., Li, Y., Zheng, Y., Zhang, Y.,
Zhao, L., Zhang, T., and Liu, Y. (2023c). Jailbreaking
chatgpt via prompt engineering: An empirical study.
CoRR, abs/2305.13860.
Mao, S., Zhang, N., Wang, X., Wang, M., Yao, Y., Jiang, Y.,
Xie, P., Huang, F., and Chen, H. (2023). Editing per-
sonality for llms. arXiv preprint arXiv:2310.02168.
Mazeika, M., Zou, A., Arora, A., Pleskov, P., Song, D.,
Hendrycks, D., Li, B., and Forsyth, D. (2022). How
hard is trojan detection in DNNs? Fooling detectors
with evasive trojans.
Menick, J., Trebacz, M., Mikulik, V., Aslanides, J., Song,
F., Chadwick, M., Glaese, M., Young, S., Campbell-
Gillingham, L., Irving, G., and McAleese, N. (2022).
Teaching language models to support answers with
verified quotes. arXiv:2203.11147.
Mialon, G., Dess
`
ı, R., Lomeli, M., Nalmpantis, C., Pa-
sunuru, R., Raileanu, R., Rozi
`
ere, B., Schick, T.,
Dwivedi-Yu, J., Celikyilmaz, A., Grave, E., LeCun,
Y., and Scialom, T. (2023). Augmented language
models: a survey. ArXiv, abs/2302.07842.
Nakano, R., Hilton, J., Balaji, S., Wu, J., Ouyang, L.,
Kim, C., Hesse, C., Jain, S., Kosaraju, V., Saun-
ders, W., Jiang, X., Cobbe, K., Eloundou, T., Krueger,
G., Button, K., Knight, M., Chess, B., and Schul-
man, J. (2021). WebGPT: Browser-assisted question-
answering with human feedback. arXiv:2112.09332.
Pedro, R., Castro, D., Carreira, P., and Santos, N. (2023).
From prompt injections to sql injection attacks: How
protected is your llm-integrated web application?
CoRR, abs/2308.01990.
Perez, F. and Ribeiro, I. (2022). Ignore previous prompt:
Attack techniques for language models. CoRR,
abs/2211.09527.
SECRYPT 2024 - 21st International Conference on Security and Cryptography
784