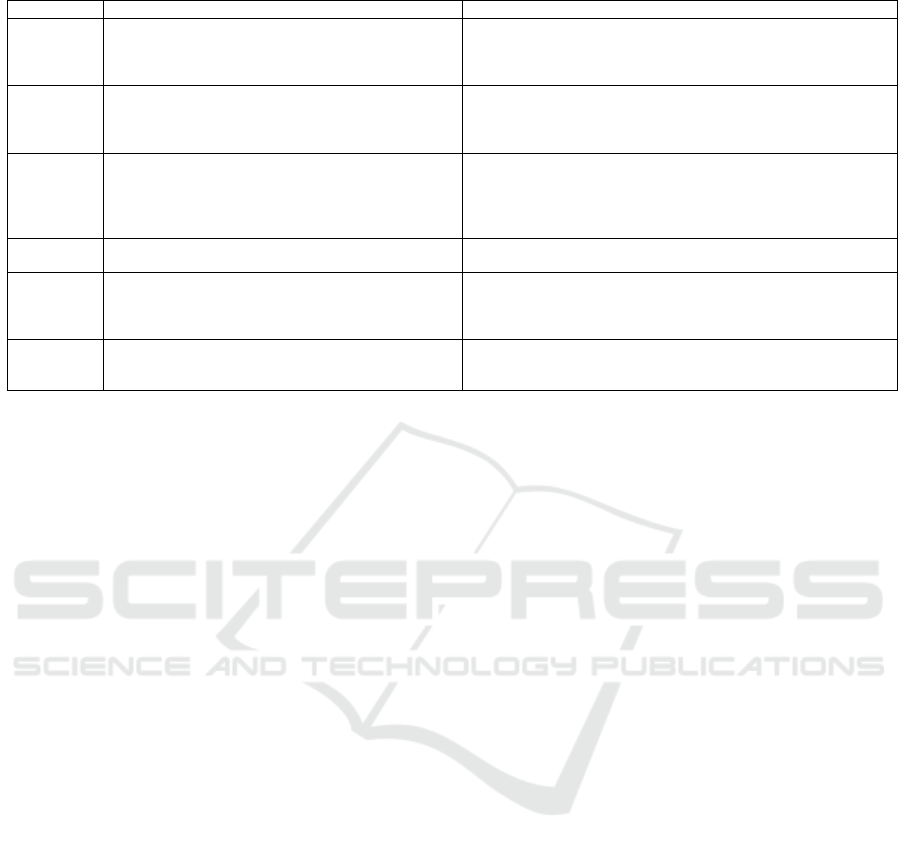
Table 11: Auditing checklist for tech companies and regulators.
Principle What to look for Potential responses and regulations
Privacy and
security
In model training, tech companies use data from all over
the Internet. There are concerns about data ownership
and data transferring.
Regulators should implement laws for data privacy and software se-
curity. For example, if the prompt contains sensitive information,
tech companies should not be allowed use that data for model train-
ing or save it in the database.
Internal au-
diting
If the prompt contains sensitive personal information,
tech companies should not use that data for model train-
ing or save it in the database.
There should be models to determine if a prompt is appropriate. If it
is inappropriate, the software should inform the user and tell the user
that this prompt violates privacy laws such as HIPPA for health data
in the USA and the prompt should not be saved to a training database.
Training
data
What data that tech companies can use for model train-
ing? Not all online data is free to use.
If the data is protected by copyright, the company shall not use the
data. For example in 2023, New York Times sued OpenAI and Mi-
crosoft for copyright infringement because the tech companies use
writings protected by copyright for model training (Grynbaum and
Mac, 2023).
Independent
audit
The software should be audited by independent auditors
for disinformation, toxicity and incorrect output.
Tech companies should also conduct self checks on the responses for
any inappropriate language.
Confidence
on the re-
sponses
No model can achieve 100% accuracy and no model is
able to know everything.
The generative AI model should tell the user how confidence about
the responses. If the the model is not so confident about the re-
sponses, the model should inform the user that the responses may
not be correct and contain possible harmful information.
Regulations
for different
use cases
Generative AI could be used in many applications. For
example, it could be used to for healthcare in the US. In
this case, it should be regulated by FDA (FDA, 2018).
Generative AI models should be regulated by multiple agencies.
Grynbaum, M. M. and Mac, R. (2023). The times sues ope-
nai and microsoft over a.i. use of copyrighted work.
H
¨
am
¨
al
¨
ainen, P., Tavast, M., and Kunnari, A. (2023). Eval-
uating large language models in generating synthetic
hci research data: a case study. In Conference on Hu-
man Factors in Computing Systems, pages 1–19.
He, D., Xia, Y., Qin, T., Wang, L., Yu, N., Liu, T.-Y., and
Ma, W.-Y. (2016). Dual learning for machine transla-
tion. Advances in neural information processing sys-
tems, 29.
IBM (2024). Foundation models: Opportunities, risks and
mitigations.
Landers, R. N. and Behrend, T. S. (2023). Auditing the
ai auditors: A framework for evaluating fairness and
bias in high stakes ai predictive models. American
Psychologist, 78(1):36.
Lefebvre, G., Summerfield, C., and Bogacz, R. (2022). A
normative account of confirmation bias during rein-
forcement learning. Neural Computation, 34(2):307–
337.
Lucy, L. and Bamman, D. (2021). Gender and representa-
tion bias in gpt-3 generated stories. In Workshop on
Narrative Understanding, pages 48–55.
Mayson, S. G. (2019). Bias in, bias out. The Yale Law
Journal, 128(8):2218–2300.
Metzler, D. and Croft, W. B. (2004). Combining the lan-
guage model and inference network approaches to
retrieval. Information processing & management,
40(5):735–750.
Meyer, S., Elsweiler, D., Ludwig, B., Fernandez-Pichel, M.,
and Losada, D. E. (2022). Do we still need human as-
sessors? prompt-based gpt-3 user simulation in con-
versational ai. In Conference on Conversational User
Interfaces, pages 1–6.
Nakatani, T. (2019). Improving transformer-based end-
to-end speech recognition with connectionist tempo-
ral classification and language model integration. In
Proc. Interspeech, volume 2019.
Palminteri, S., Lefebvre, G., Kilford, E. J., and Blake-
more, S.-J. (2017). Confirmation bias in human re-
inforcement learning: Evidence from counterfactual
feedback processing. PLoS computational biology,
13(8):e1005684.
Raji, I. D., Smart, A., White, R. N., Mitchell, M., Gebru,
T., Hutchinson, B., Smith-Loud, J., Theron, D., and
Barnes, P. (2020). Closing the ai accountability gap:
Defining an end-to-end framework for internal algo-
rithmic auditing. In Conference on fairness, account-
ability, and transparency, pages 33–44.
Sap, M., Gabriel, S., Qin, L., Jurafsky, D., Smith, N. A.,
and Choi, Y. (2019). Social bias frames: Reason-
ing about social and power implications of language.
arXiv:1911.03891.
Schechner, S. (2023). Chatgpt and advanced ai face new
regulatory push in europe.
Shah, D., Schwartz, H. A., and Hovy, D. (2019). Predictive
biases in natural language processing models: A con-
ceptual framework and overview. arXiv:1912.11078.
Shan, C., Weng, C., Wang, G., Su, D., Luo, M., Yu, D.,
and Xie, L. (2019). Component fusion: Learning re-
placeable language model component for end-to-end
speech recognition system. In Conference on Acous-
tics, Speech, and Signal Processing, pages 5361–
5635. IEEE.
Shepardson, D. and Bartz, D. (2023). Us begins study of
possible rules to regulate ai like chatgpt.
Strohman, T., Metzler, D., Turtle, H., and Croft, W. B.
(2005). Indri: A language model-based search en-
gine for complex queries. In Conference on intelligent
analysis, volume 2, pages 2–6.
Tarantola, T., Folke, T., Boldt, A., P
´
erez, O. D., and Mar-
tino, B. D. (2021). Confirmation bias optimizes re-
ward learning. BioRxiv, pages 2021–02.
Toshniwal, S., Kannan, A., Chiu, C.-C., Wu, Y., Sainath,
T. N., and Livescu, K. (2018). A comparison of
techniques for language model integration in encoder-
It is Time to Develop an Auditing Framework to Promote Value Aware Chatbots
469