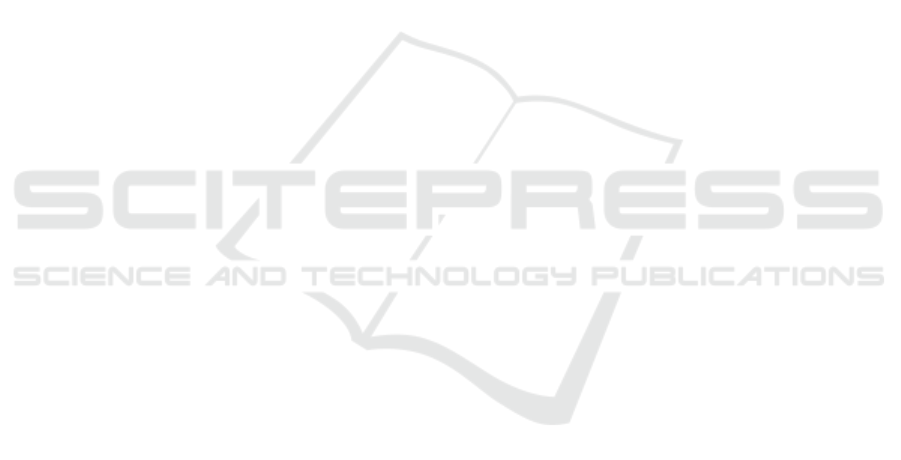
ators in expanding their data and system architectures
to align with the concepts of data ecosystems and data
spaces. Consequently, this can lead to more exten-
sive data bases and support the creation of applica-
tions based upon them.
5.2 Limitations & Future Work
Despite following a rigorous approach, our study is
subject to several limitations, which offer paths for
future work. Most importantly, our developments are
shaped by the context in which we developed our so-
lution and thus limited in this regard. Implementing
our prototype in further scenarios can help us iden-
tify further requirements and lead to more profound
design knowledge. Additionally, we plan to extend
our prototype functionally. These developments can
include: (1) a more in-depth search functionality that
includes all relevant metadata, (2) allowing to filter
data sets based on file size, file type, last modified,
etc., (3) conducting tests on high-volume data sets and
runtime analyses.
Concerning our methodological approach, our
evaluation results are based on qualitative feedback
from participants working in the organizational con-
text of our prototype. Additional studies featuring a
more diverse group of participants can help us gather
additional feedback and improve the interoperability
of our approach. Based on such insights coming from
multiple cases, we plan to formulate a robust set of
design principles that can assist other researchers and
practitioners in designing and developing data sharing
solutions for CKAN-based data platforms.
REFERENCES
Amadori, A., Altendeitering, M., and Otto, B. (2020). Chal-
lenges of data management in industry 4.0: A single
case study of the material retrieval process. In Busi-
ness Information Systems: 23rd International Confer-
ence, BIS 2020, Colorado Springs, CO, USA, June 8–
10, 2020, Proceedings 23, pages 379–390. Springer.
Azkan, C., M
¨
oller, F., Meisel, L., and Otto, B. (2020). Ser-
vice dominant logic perspective on data ecosystems-a
case study based morphology. In Proceedings of the
28th European Conference on Information Systems.
Bader, S. R., Pullmann, J., Mader, C., Tramp, S., Quix,
C., M
¨
uller, A. W., Aky
¨
urek, H., B
¨
ockmann, M., Im-
busch, B. T., Lipp, J., Geisler, S., and Lange, C.
(2020). The International Data Spaces Information
Model – An Ontology for Sovereign Exchange of Dig-
ital Content. In International Semantic Web Confer-
ence, pages 176–192. Springer.
Bertot, J. C., Jaeger, P. T., and Grimes, J. M. (2010). Using
icts to create a culture of transparency: E-government
and social media as openness and anti-corruption tools
for societies. Government Information Quarterly,
27(3):264–271.
Catena-X (2024). Catena-X. (Accessed: 03.04.2024).
Chakrabarti, A., Quix, C., Geisler, S., Pullmann, J., Khro-
mov, A., and Jarke, M. (2018). Goal-Oriented Mod-
elling of Relations and Dependencies in Data Mar-
ketplaces. In Proceedings of the 11th Inter-national
Workshop i* co-located with the 30th International
Conference on Advanced Information Systems Engi-
neering.
CKAN (2024a). CKAN. (Accessed: 08.04.2024).
CKAN (2024b). CKAN DCAT extension. (Accessed:
08.04.2024).
for Economic Affairs, F. M. and Action, C. (2024). How to
share data? Data sharing platforms for orginizations.
(Accessed: 08.04.2024).
Foundation, E. (2024a). Eclipse Dataspace Components.
(Accessed: 08.04.2024).
Foundation, E. (2024b). HTTP Data Plane. (Accessed:
08.04.2024).
Foundation, E. (2024c). Minimum Viable Dataspace. (Ac-
cessed: 05.04.2024).
Germany, G. (2024). GovData Germany. (Accessed:
08.04.2024).
Gr
¨
oger, C. (2021). There is no ai without data. Communi-
cations of the ACM, 64(11):98–108.
Hollander, J. A. (2004). The social contexts of focus groups.
Journal of contemporary ethnography, 33(5):602–
637.
Hosseinzadeh, A., Eitel, A., and Jung, C. (2020). A Sys-
tematic Approach toward Extracting Technically En-
forceable Policies from Data Usage Control Require-
ments. In Proceedings of the 6th International Con-
ference on Information Systems Security and Privacy,
pages 397–405.
IDSA (2024a). Data Connector Report. (Accessed:
03.04.2024).
IDSA (2024b). Dataspace Protocol 2024-1. (Accessed:
03.04.2024).
Janev, V., Vidal, M. E., Endris, K., and Pujic, D. (2021).
Managing Knowledge in Energy Data Spaces. In
Companion Proceedings of the Web Conference 2021,
pages 7–15, New York, NY, USA. ACM.
Kabalisa, R. and Altmann, J. (2021). Ai technologies and
motives for ai adoption by countries and firms: a
systematic literature review. In Economics of Grids,
Clouds, Systems, and Services: 18th International
Conference, GECON 2021, Virtual Event, September
21–23, 2021, Proceedings 18, pages 39–51. Springer.
Kitchenham, B. A., Dyba, T., and Jorgensen, M. (2004).
Evidence-based software engineering. In Proceed-
ings. 26th International Conference on Software En-
gineering, pages 273–281. IEEE.
Kitchenham, B. A., Pfleeger, S. L., Pickard, L. M., Jones,
P. W., Hoaglin, D. C., El Emam, K., and Rosenberg, J.
(2002). Preliminary guidelines for empirical research
in software engineering. IEEE Transactions on soft-
ware engineering, 28(8):721–734.
DATA 2024 - 13th International Conference on Data Science, Technology and Applications
492