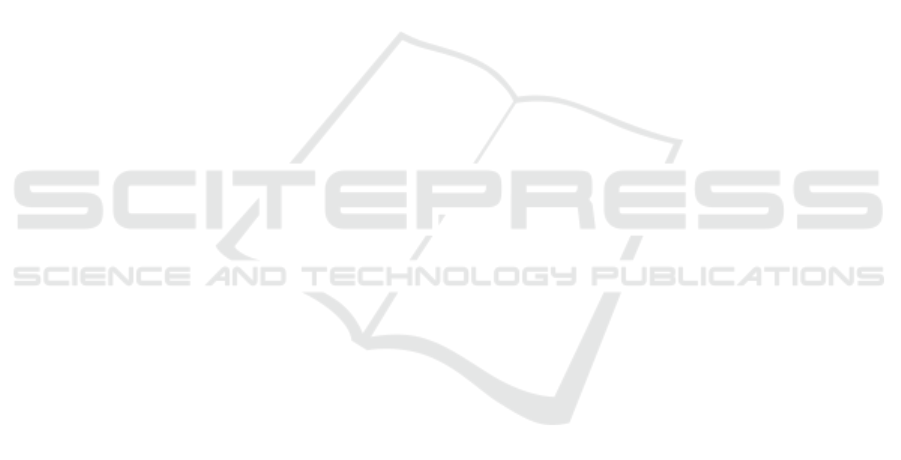
could be much longer for them. Furthermore, in Fig-
ure 8, after 5,000 days, it appears that all the groups
have collapsed. These groups have been replaced or
modified supporting the model.
4 CONCLUSIONS
The study is based on a comparison of the reliabil-
ity of ten turbines belonging to two different fami-
lies, using a frailty model. To do this, we used a non-
homogeneous Poisson process with a power law and
the gamma frailty distribution. The model parameters
are found by optimizing the marginal likelihood func-
tion developed in the study. The likelihood function
takes into account censored historical maintenance
data, the gamma frailty distribution and the final indi-
vidual observation time. In addition, the frailty effect
z is not considered as a random variable, but is de-
rived directly from the optimization of the individual
conditional likelihood function. However, for turbine
runners with no observed failures, the heterogeneity
effect was zero, which led us to abandon the frailty
model in this case. We have discovered that by de-
riving z, the frailty effect, as an expression of fail-
ure statuses and individual final observation time, and
adding censoring to the model, the reliability curves
express the results much better and are very close to
reality. For example, groups in family B that have
been replaced due to recurring cracks have a reliabil-
ity of less than 20% at 3,000 days and have collapsed
at around 5,000 days, whereas groups in family A
are robust. Despite the fact that we neglected covari-
ates in the model, we obtained informative results that
may help the maintenance team to group turbines with
the same reliability trend and monitor only one tur-
bine in order to reduce double inspections. This study
could be strengthened by adding important covariates
such as age, turbine runner modification, start-up and
shutdown frequencies. Future work could also focus
on optimizing systematic inspections with regard to
the evolution of reliability curves, and avoiding the
multiplication of maintenance shutdowns, which have
a negative impact on cracking.
ACKNOWLEDGEMENTS
The authors are grateful to Turbomachinery Labora-
tory of the Engineering School of the University of
Quebec in Abitibi-T
´
emiscamingue (UQAT), to Hydro-
Qu
´
ebec’s Reseach Institute and to Hydro-Qu
´
ebec for
the collaboration.
REFERENCES
Abbring, J. H. and Berg, G. J. V. D. (2007). The unob-
served heterogeneity distribution in duration analysis.
In Biometrika. 94(1).
Ascher, H. E. (1968). Evaluation of repairable system reli-
ability using the bad-as-old concept. In IEEE Trans-
actions on Reliability. 17(2).
Ascher, H. E. (2008). Repairable systems reliability. In
Encyclopedia of Statistics in Quality and Reliability.
1.
Asfaw, Z. G. and Lindqvist, B. H. (2015). Unobserved het-
erogeneity in the power law nonhomogeneous poisson
process. In Reliability Engineering and System Safety.
134.
Bajgholi, M. E., Rousseau, G., Viens, M., and Thibault, D.
(2021). Capability of advanced ultrasonic inspection
technologies for hydraulic turbine runners. In pplied
Sciences. 11.
Bajgholi, M. E., Rousseau, G., Viens, M., Thibault, D., and
Gagnon, M. (2023). Reliability assessment of non de-
structive testing (ndt) for the inspection of weld joints
in the hydroelectric turbine industry. In The Interna-
tional Journal of Advanced Manufacturing Technol-
ogy. 128.
Brown, B., Liu, B., McIntyre, S., and Revie, M. (2023). Re-
liability evaluation of repairable systems considering
component heterogeneity using frailty model. In Pro-
ceedings of the Institution of Mechanical Engineers,
Part O: Journal of Risk and Reliability. 237(4).
Duchateau, L. and Janssen, P. (2008). The frailty model. In
New York: Springer Verlag. 1.
Gagnon, M. and Nicolle, J. (2019). On variations in turbine
runner dynamic behaviours observed within a given
facility. In Conference Series: Earth and Environmen-
tal Science. 450(1).
Gagnon, M., Tahan, A., Bocher, P., and Thibault, D. (2014).
Influence of load spectrum assumptions on the ex-
pected reliability of hydroelectric turbines: A case
study. In Structural Safety. 50.
Gagnon, M., Tahan, S. A., Bocher, P., and Thibault, D.
(2010). Impact of startup scheme on francis runner
life expectancy. In 25th IAHR Symposium. Timisoara.
Georgievskaia, E. (2020). Predictive analytics as a way to
smart maintenance of hydraulic turbinest. In Procedia
Structural Integrity. 28.
Georgievskaia, E. (2021). Analytical system for predicting
cracks in hydraulic turbines. In Engineering Failure
Analysis. 127.
Haji, S. H. and Abdulazeez, A. M. (2021). Comparison
of optimization techniques based on gradient descent
algorithm: A review. In PalArch’s Journal of Archae-
ology of Egyptology. 18(4).
Hydro-Qu
´
ebec (2023). Annual report. In Report. 1.
Ivanchenko, I. P. and Prokopenko, A. N. (2020). Analyzing
the operational materials on crack formation in blades
of francis turbines at the krasnoyarsk hpp. In . Power
Technology and Engineering. 53(6).
SIMULTECH 2024 - 14th International Conference on Simulation and Modeling Methodologies, Technologies and Applications
136