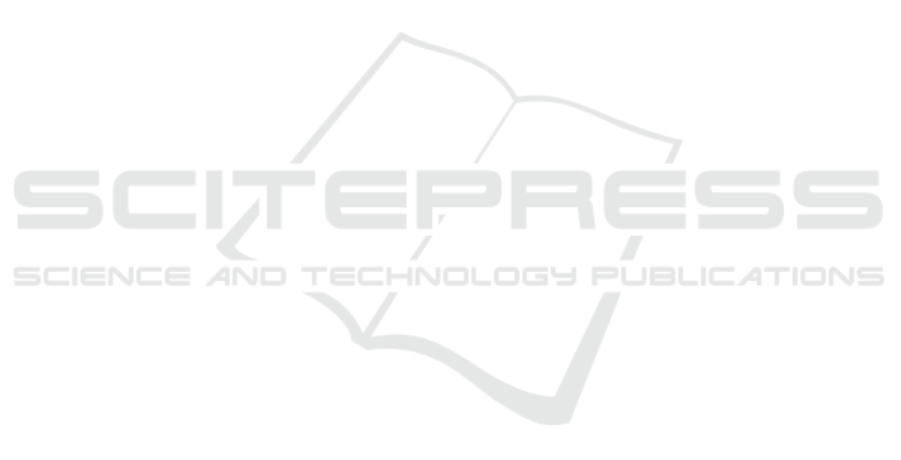
most malaria cases typically ranges from seven to
thirty days, representing the time between the bite
of an infected Anopheles mosquito and the onset of
initial symptoms. Malaria symptoms can vary from
mild or nonexistent to severe and life-threatening.
Common symptoms include fever, chills, sweating,
headaches, body aches, nausea, vomiting, and gen-
eral malaise. Preventing and reducing malaria trans-
mission primarily rely on two forms of vector control:
insecticide-treated mosquito nets and indoor residual
spraying. Additionally, antimalarial medications play
a crucial role in preventing Malaria. Suspected cases
are confirmed through parasite-based diagnostic test-
ing, which may involve microscopy or a rapid diag-
nostic test. Early diagnosis and treatment are im-
perative to reduce transmission and prevent fatalities
(CDCP, 2020b).
Several risk factors contribute to the prevalence
of Malaria, including climatic and environmental fac-
tors, genetic factors, and population density. Socio-
economic and behavioral risk factors, such as a lack
of knowledge about Malaria and its control, cul-
tural adherence to traditional and ineffective treat-
ments, and entering endemic regions without preven-
tive measures due to poverty or ignorance, also play
a role. Human activities that create mosquito breed-
ing sites, as well as night-time exposure of farmers to
mosquito bites during agricultural work, contribute to
the risk. Additionally, health system-related factors,
such as shortages in human and financial resources,
drugs, and equipment, impact the control and man-
agement of Malaria (CDCP, 2020a). Numerous re-
search studies have unequivocally established a sig-
nificant link between vector-borne diseases and envi-
ronmental factors, offering a key to predicting disease
outbreaks and implementing effective control mea-
sures. Coldblooded arthropod vectors, crucial agents
in these diseases, undergo profound impacts due to
temperature fluctuations, influencing their develop-
ment, behavior, reproduction, and overall population
dynamics. Moreover, the interplay of temperature
with humidity affects pathogen development within
vectors. The significance of rainfall and seasonality
in creating breeding grounds for disease vectors can-
not be understated, further emphasizing the diverse
environmental risk factors, including altitude, slope,
soil type, vegetation, and land use/land cover (Gage
et al., 2008).
2.2 Climatic Factors and Malaria
Transmission
The triad of temperature, relative humidity, and pre-
cipitation emerges as pivotal in the context of malaria
transmission, orchestrating spatiotemporal changes in
malaria vectors. Rainfall, humidity, climate seasonal-
ity, and temperature collectively contribute to 70%-
90% of the malaria risk. The transmission of Plas-
modium falciparum, the causative parasite, is intri-
cately tied to temperature thresholds, with limitations
below 16°C – 19°C and above 33°C – 39°C. Relative
humidity plays a multifaceted role, impacting vector
breeding, parasite development, and the spatial diffu-
sion of malaria transmission. Areas with high vege-
tation in close proximity to human habitation become
hotspots for malaria transmission, particularly when
the distance from mosquito breeding sites is less than
2.5 km (Palaniyandi et al., 2017).
2.3 Forests as Malaria Hotspots
Forests emerge as fertile grounds for malaria trans-
mission due to conducive conditions— vegetation
cover, temperature, rainfall, and humidity—favoring
the distribution and survival of malaria vectors. Tribal
populations dwelling in forested areas rely predom-
inantly on indigenous treatments due to factors like
illiteracy, adherence to age-old traditions, and a deep-
seated fear of the external world. The challenges
are compounded by poor communication infrastruc-
ture, particularly during the rainy season, when
mosquito dispersal dynamics are affected by even
slight changes in distances from bodies of water (Kar
et al., 2014).
2.4 Recent Studies and Geographical
Variations
Over the past decade, numerous studies have delved
into the association between malaria incidence and
climatic factors, presenting a nuanced understand-
ing of the temporal dynamics. Investigations in
China, South Africa, Iran, Thailand, Uganda, Burk-
ina Faso, and India have provided valuable insights
into the complex relationships involving meteorolog-
ical factors. The temporal lagged association between
weekly malaria incidence and meteorological factors
in 30 counties in southwest China from 2004 to 2009
is shown in (Zhao et al., 2014). Also, an inves-
tigation of the effect of monthly rainfall variations
on malaria transmission in five districts of Limpopo
Province of South Africa for the period 1998 to 2017
is presented in (Adeola et al., 2019). Furthermore,
the association of monthly malaria incidence with cli-
matic factors from 2000 to 2012 was studied in Sis-
tan and Baluchestan, Iran (Mohammadkhani et al.,
2019). The association of weekly malaria incidence
with climatic data throughout the country from 2012
An Exploratory Analysis of Malaria and Climatic Factors in India
161