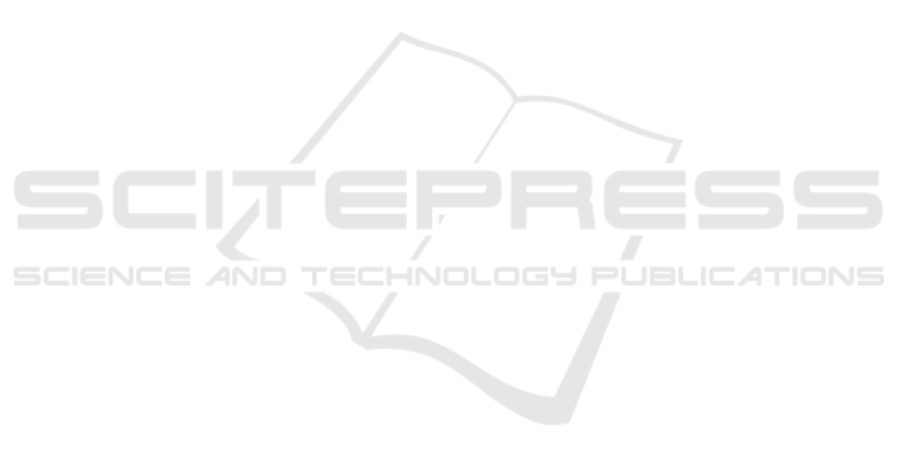
the fully convolutional model that mimics the
transformers, all these state-of-the-art models have
obtained notable results in medical image
segmentation. UNet, as an established solution, along
with its variants, can obtain accurate and robust
results on several tasks after training. Transformer-
based models, which are good at capturing long-range
dependencies and processing large-scale images with
high efficiency, are more suitable for tasks requiring
considering contextual information over a large
spatial extent, such as organ segmentation, pathology
localization, vascular segmentation, and other tasks.
The more innovative models that utilize FCN to
realize the advantages of transformers can also reduce
the number of required parameters while considering
the advantages, which is also a promising point of
view. It is also a matter of choosing the right model
for different tasks, taking into account data and
hardware constraints. On the other hand, the
experimental data presented in the papers show that
the accuracy of the model is usually around 80% to
90%, which is not enough in the medical field that
requires strict matching. Some special cases are
difficult to overcome, such as pancreas image
segmentation, which achieves relatively poor results
compared to the others. Focusing on the models like
Chat-GPT that are now revolutionizing the field of
NLP, or even many parts of human life, it hope that
one day a generalized medical image segmentation
model that can be widely used in real applications will
also be available and revolutionize the medical field.
REFERENCES
A. Dosovitskiy, L. Beyer, A. Kolesnikov, et al, arXiv
(Cornell University), (2020).
A. Vaswani, N. Shazeer, N. Parmar, et al, arXiv (Cornell
University), 30, 5998–6008, (2017).
A. Z. Alali, K. H. Ali, Diyala Journal of Engineering
Science, 17–29, (2022).
D. Bahdanau, K. Cho, Y. Bengio, arXiv (Cornell
University), (2015).
D. Patil, S. G. Deore, IJCSMC, (2013).
F. Shamshad, S. Khan, S. W. Zamir, et al, Medical Image
Analysis, 88, 102802, (2023).
H. Cao, Y. Wang, J. Chen, et al, arXiv (Cornell University),
(2021).
H. Dong, G. Yang, F. Liu, et al, arXiv (Cornell University),
(2017).
H. Thisanke, C. Deshan, K. Chamith, et al, Engineering
Applications of Artificial Intelligence, 126, 106669,
(2023).
I. H. Sarker, SN Computer Science, 2(6), (2021).
J. Chen, Y. Lu, Q. Yu, et al, arXiv (Cornell University),
(2021).
K. Ramesh, G. Kumar, K. Swapna, et al, EAI Endorsed
Transactions on Pervasive Health and Technology,
(2018).
L. Alzubaidi, J. Zhang, A. J. Humaidi, et al, Journal of Big
Data, 8(1), (2021).
N. Carion, F. Massa, G. Synnaeve, et al, arXiv (Cornell
University), (2020).
N. Ibtehaz, D. Kihara, arXiv (Cornell University), (2023).
O. Oktay, J. Schlemper, L. L. Folgoc, et al, arXiv (Cornell
University), (2018).
O. Ronneberger, P. Fischer, T. Brox, In Lecture Notes in
Computer Science (pp. 234–241), (2015).
S. Ghosh, A. Chaki, K. C. Santosh, Physical and
Engineering Sciences in Medicine, 44(3), 703–712,
(2021).
X. Liu, L. Song, S. Liu, et al, Sustainability, 13(3), 1224,
(2021).
Z. Liu, Y. Lin, Y. Cao, et al, 2021 IEEE/CVF International
Conference on Computer Vision (ICCV), (2021).
UNet and Transformers: Deep Learning Based Methods for Medical Image Segmentation
551