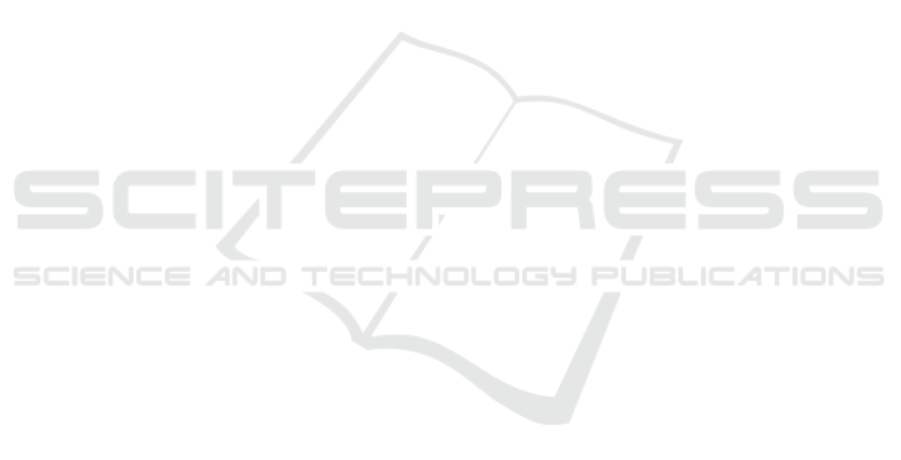
tested cell counts ranging from 4 to 100 cells, specif-
ically focusing on square numbers within this range.
Figure 4a shows the outcome of this experiment for
four different epsilon values 1, 2, 4, and 8. The trend
reveals that smaller cell sizes, corresponding to higher
cell counts, generally result in greater accuracy across
all epsilon values up to 81 cell counts. This observa-
tion underscores the existence of a trade-off associ-
ated with the number of cells, where simply increas-
ing cell counts does not guarantee accuracy enhance-
ment. This observation resonates with the findings of
our theoretical analysis. The variation of privacy bud-
get does not show a significant change in accuracy.
This can be resulted from the distribution of data un-
der analysis. In other words, even the higher random-
ness noise still keeps the structure of data properly
for clustering. It of course needs more investigation
in future studies for variety of datasets. This outcome
specifically suggests the use of lower epsilon values
(higher privacy gain) in our methodology. To gain a
better insight on the dispersion of accuracy on 50 runs
of experiments, we depict this variance in Figure 4b
for epsilon 1 and different cell counts. It can be seen
that the widest range of values is observed with 64
cells, where accuracy spans from a minimum of 18%
to a maximum of 90%, reflecting a variance of 72%.
While this dispersion is unavoidable due to the ran-
domness inherent property of LDP, it can be seen that
yet increasing the number of cell counts leads to the
improvement in accuracy.
The Influence of Dataset Size: Figure 6 shows the
impact of dataset size by considering fractions of data
on the accuracy of our methodology. For this dataset,
the amount of data points in the data set does not
seem to consistently influence the accuracy we can
achieve using RAPPOR. The values stay pretty con-
sistent across all data set sizes and there is no consis-
tent trend. The chosen Epsilon value does not seem to
have much influence on the accuracy either. This can
be resulted from the distribution of our dataset and the
precision of RAPPOR in preserving the distribution
of data even in smaller sizes.
Once again, Figure 6 reveals a notable disparity
among accuracy values across experiment runs. Some
runs exhibit considerably low accuracy rates, while
others nearly achieve 100%. Particularly intriguing is
the scenario involving the smallest dataset size, com-
prising only a fraction of 0.1 relative to the original
dataset size. Here, the attained accuracy spans from a
minimum of 14% to a maximum of 95%. Despite this
variance, computing the median accuracy across all
experiment runs still yields commendable results. In-
terestingly, the dataset sample size of 0.9 of the origi-
nal dataset demonstrates the least dispersion, with ac-
curacy ranging from 62% to 96%.
Discussion. We present the guidelines for using our
framework, our experimental findings, and our plan
for future directions.
• We have assumed that the features are continuous.
However, our methodology can also be applied on
discrete features. To this end, it is enough that we
use the boundary of cells as shared value and as
randomized value by the aggregator.
• We found that the number of cells has impact on
the accuracy of our methodology both in negative
and positive way. There is a trade-off in the num-
ber of cells for each dataset that the accuracy is
optimized. However, it should be noted that the
aggregator has no knowledge about the data to of-
fer the optimum number of cells in advance. To
this end, in the future directions, we plan to de-
sign a privacy-preserving mechanism to infer the
optimum number of cells without accessing the
original data.
• Although our experiments did not show a con-
siderable impact of dataset size on accuracy, we
believe that this requires more extensive exper-
iments when also the dataset distribution also
comes under consideration. Given the inherent
property of LDP mechanism, we expect that the
size of dataset single alone might night affect the
outcome if the dataset if the data is almost well
evenly distributed across all cells.
• We found the optimum number of clusters using
elbow technique on original datset. This is some-
thing that the aggregator does not know without
accessing the original data. In future direction,
we plan to investigate the impact of the number of
clusters on accuracy.
5 CONCLUSION
This study introduces a novel framework that lever-
ages Local Differential Privacy (LDP) to safeguard
individual data privacy, empowering users to take
proactive measures to protect their information be-
fore any sharing occurs with third parties in a non-
interactive engagement of users. Through a compre-
hensive series of experiments, we provide compelling
evidence of the efficacy of our approach in preserv-
ing data privacy while also enabling meaningful and
insightful clustering analysis.
SECRYPT 2024 - 21st International Conference on Security and Cryptography
824