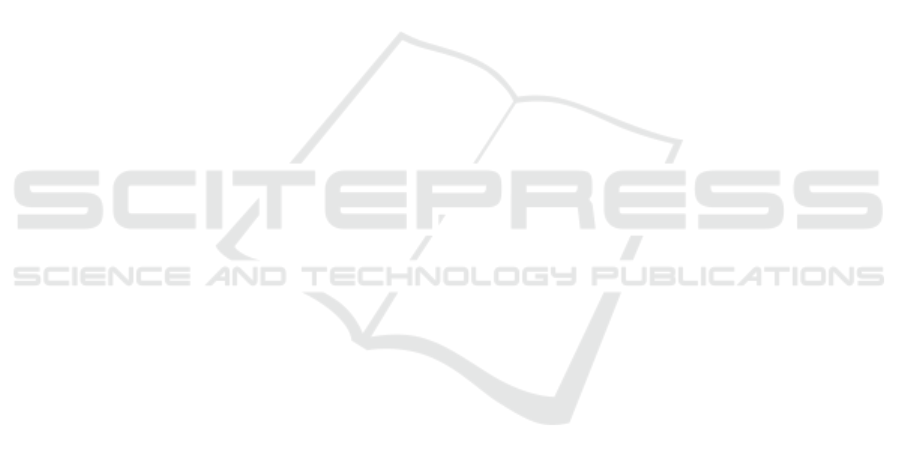
tack on PCA, showcasing the attacker’s success in re-
constructing the original databases. Our results reveal
that anonymized eigenvectors maintain good utility
compared to the original ones. Differential Privacy
(DP) is also utilised in FL-PCA (Grammenos et al.,
2020). In future, we will also consider DP to pro-
tect eigenvectors. As, in this work, we quantify in-
dividual privacy leakage arising from sharing of local
eigenvectors, which were derived from the data of in-
dividual clients. So, our future investigation will ex-
tend to privacy leakage post-aggregation. In the case,
when global eigenvectors are compromised, there is
a potential risk of the attacker to deduce the records
of specific individuals, particularly those who are in-
fluencing the aggregation step predominantly. Hence,
our future research aims to focus on the performance
of PP-PCA when aggregated or global eigenvectors are
compromised in a FL scenario.
ACKNOWLEDGEMENT
This study was partially funded by the Wallenberg AI;
Autonomous Systems and Software Program (WASP)
funded by the Knut and Alice Wallenberg Foun-
dation; the computations were enabled by the su-
percomputing resource Berzelius provided by Na-
tional Supercomputer Centre at Link
¨
oping University
and the Knut and Alice Wallenberg foundation; the
EU NextGenerationEU programme under the funding
schemes PNRR-PE-AI FAIR (Future Artificial Intel-
ligence Research); PNRR-“SoBigData.it - Strength-
ening the Italian RI for Social Mining and Big Data
Analytics” - Prot. IR0000013; the EU – Horizon 2020
Program under the scheme “INFRAIA-01-2018-2019
– Integrating Activities for Advanced Communities”
(G.A. n.871042) “SoBigData++: European Integrated
Infrastructure for Social Mining and Big Data Analyt-
ics” (http://www.sobigdata.eu).
REFERENCES
Abdi, H. and Williams, L. J. (2010). Principal component
analysis. Wiley interdisciplinary reviews: computa-
tional statistics, 2(4):433–459.
Briguglio, W., Yousef, W. A., Traor
´
e, I., and Mamun, M.
(2023). Federated supervised principal component
analysis. IEEE Transactions on Information Forensics
and Security.
Grammenos, A., Mendoza Smith, R., Crowcroft, J., and
Mascolo, C. (2020). Federated principal component
analysis. Advances in neural information processing
systems, 33:6453–6464.
Hartebrodt, A. and R
¨
ottger, R. (2022). Federated hori-
zontally partitioned principal component analysis for
biomedical applications. Bioinformatics Advances,
2(1):vbac026.
Kwatra, S. and Torra, V. (2023). Data reconstruction at-
tack against principal component analysis. In Interna-
tional Symposium on Security and Privacy in Social
Networks and Big Data, pages 79–92. Springer.
LeFevre, K., DeWitt, D. J., and Ramakrishnan, R.
(2006). Mondrian multidimensional k-anonymity. In
22nd International conference on data engineering
(ICDE’06), pages 25–25. IEEE.
Liang, Y., Balcan, M.-F. F., Kanchanapally, V., and
Woodruff, D. (2014). Improved distributed principal
component analysis. Advances in neural information
processing systems, 27.
McMahan, B., Moore, E., Ramage, D., Hampson, S., and
y Arcas, B. A. (2017). Communication-efficient learn-
ing of deep networks from decentralized data. In Ar-
tificial intelligence and statistics, pages 1273–1282.
PMLR.
Pathak, M. A. and Raj, B. (2011). Efficient protocols for
principal eigenvector computation over private data.
Trans. Data Priv., 4(3):129–146.
Samarati, P. (2001). Protecting respondents identities in mi-
crodata release. IEEE transactions on Knowledge and
Data Engineering, 13(6):1010–1027.
Samarati, P. and Sweeney, L. (1998). Protecting privacy
when disclosing information: k-anonymity and its en-
forcement through generalization and suppression.
Shokri, R., Stronati, M., Song, C., and Shmatikov, V.
(2017). Membership inference attacks against ma-
chine learning models. In 2017 IEEE symposium on
security and privacy (SP), pages 3–18. IEEE.
Sweeney, L. (2002). k-anonymity: A model for protecting
privacy. International journal of uncertainty, fuzziness
and knowledge-based systems, 10(05):557–570.
Voigt, P. and Von dem Bussche, A. (2017). The eu gen-
eral data protection regulation (gdpr). A Practical
Guide, 1st Ed., Cham: Springer International Pub-
lishing, 10(3152676):10–5555.
Xu, L., Skoularidou, M., Cuesta-Infante, A., and Veera-
machaneni, K. (2019). Modeling tabular data using
conditional gan. Advances in Neural Information Pro-
cessing Systems, 32.
Zari, O., Parra-Arnau, J.,
¨
Unsal, A., Strufe, T., and
¨
Onen,
M. (2022). Membership inference attack against prin-
cipal component analysis. In Privacy in Statisti-
cal Databases: International Conference, PSD 2022,
Paris, France, September 21–23, 2022, Proceedings,
pages 269–282. Springer.
Zhu, L., Liu, Z., and Han, S. (2019). Deep leakage from
gradients. Advances in neural information processing
systems, 32.
Balancing Act: Navigating the Privacy-Utility Spectrum in Principal Component Analysis
857