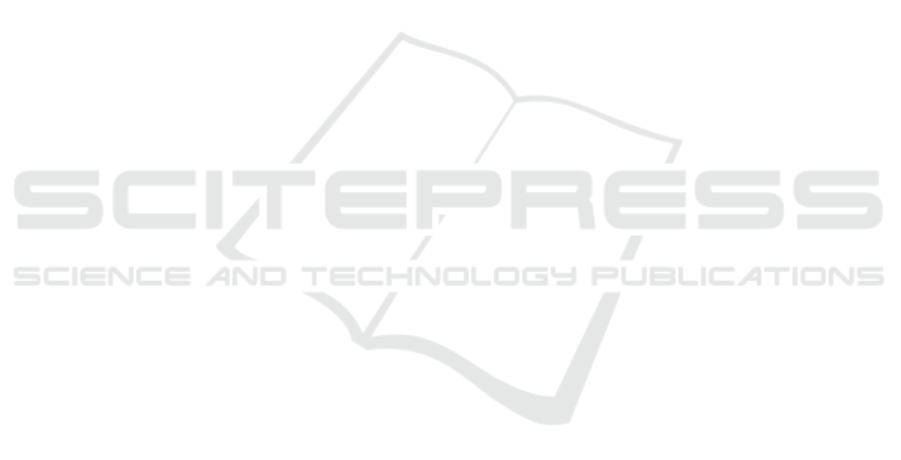
Golec, M., Iftikhar, S., Prabhakaran, P., Gill, S. S., and Uh-
lig, S. (2023c). Qos analysis for serverless comput-
ing using machine learning. In Serverless Computing:
Principles and Paradigms, pages 175–192. Springer.
Golec, M., Ozturac, R., Pooranian, Z., Gill, S. S., and
Buyya, R. (2021). Ifaasbus: A security-and privacy-
based lightweight framework for serverless comput-
ing using iot and machine learning. IEEE Transac-
tions on Industrial Informatics, 18(5):3522–3529.
Golec, M., Walia, G. K., Kumar, M., Cuadrado, F.,
Gill, S. S., and Uhlig, S. (2023d). Cold start la-
tency in serverless computing: A systematic review,
taxonomy, and future directions. arXiv preprint
arXiv:2310.08437.
Hossain Faruk, M. J., Alam, F., Islam, M., and Rahman,
A. (2024). Transforming online voting: a novel sys-
tem utilizing blockchain and biometric verification for
enhanced security, privacy, and transparency. Cluster
Computing, pages 1–20.
Iftikhar, S., Gill, S. S., Song, C., Xu, M., Aslanpour, M. S.,
Toosi, A. N., Du, J., Wu, H., Ghosh, S., Chowdhury,
D., et al. (2023). Ai-based fog and edge computing:
A systematic review, taxonomy and future directions.
Internet of Things, 21:100674.
Iftikhar, S., Golec, M., Chowdhury, D., Gill, S. S., and Uh-
lig, S. (2022a). Fogdlearner: A deep learning-based
cardiac health diagnosis framework using fog comput-
ing. In Proceedings of the 2022 Australasian Com-
puter Science Week, pages 136–144. ACM.
Iftikhar, S., Raj, U., Tuli, S., Golec, M., Chowd-
hury, D., Gill, S. S., and Uhlig, S. (2022b).
Tesco: Multiple simulations based ai-augmented
fog computing for qos optimization. In 2022
IEEE Smartworld, Ubiquitous Intelligence &
Computing, Scalable Computing & Communica-
tions, Digital Twin, Privacy Computing, Meta-
verse, Autonomous & Trusted Vehicles (Smart-
World/UIC/ScalCom/DigitalTwin/PriComp/Meta),
pages 2092–2099. IEEE.
Jacob, B., Brown, M., Fukui, K., Trivedi, N., et al. (2005).
Introduction to grid computing. IBM redbooks, pages
3–6.
Li, L., Fan, Y., Tse, M., and Lin, K.-Y. (2020). A review
of applications in federated learning. Computers &
Industrial Engineering, 149:106854.
Li, Y., Zhang, H., Zhang, C., Huang, T., and Yu, F. R.
(2024). A survey of quantum internet protocols from
a layered perspective. IEEE Communications Surveys
& Tutorials.
Liu, J., Liu, M., Liu, J.-P., Ye, Z., Wang, Y., Alexeev, Y.,
Eisert, J., and Jiang, L. (2024). Towards provably
efficient quantum algorithms for large-scale machine-
learning models. Nature Communications, 15(1):434.
Mammen, P. M. (2021). Federated learning: Opportunities
and challenges. arXiv preprint arXiv:2101.05428.
Murugesan, S. S., Velu, S., Golec, M., Wu, H., and Gill,
S. S. (2024). Neural networks based smart e-health
application for the prediction of tuberculosis using
serverless computing. IEEE Journal of Biomedical
and Health Informatics.
Nandhakumar, A. R., Baranwal, A., Choudhary, P., Golec,
M., and Gill, S. S. (2024). Edgeaisim: A toolkit
for simulation and modelling of ai models in edge
computing environments. Measurement: Sensors,
31:100939.
O’brien, J. L. (2007). Optical quantum computing. Science,
318(5856):1567–1570.
Pallewatta, S., Kostakos, V., and Buyya, R. (2023). Place-
ment of microservices-based iot applications in fog
computing: A taxonomy and future directions. ACM
Computing Surveys, 55(14s):1–43.
Plank, J. S. (1997). A tutorial on reed–solomon coding for
fault-tolerance in raid-like systems. Software: Prac-
tice and Experience, 27(9):995–1012.
Reyna, A., Mart
´
ın, C., Chen, J., Soler, E., and D
´
ıaz, M.
(2018). On blockchain and its integration with iot.
challenges and opportunities. Future generation com-
puter systems, 88:173–190.
Sarabia-J
´
acome, D., Gim
´
enez-Ant
´
on, S., Liatifis, A., Grasa,
E., Catal
´
an, M., and Pliatsios, D. (2024). Progressive
adoption of rina in iot networks: Enhancing scalability
and network management via sdn integration. Applied
Sciences, 14(6):2300.
Sen, A. A. A. and Yamin, M. (2021). Advantages of us-
ing fog in iot applications. International Journal of
Information Technology, 13:829–837.
Singh, R. and Gill, S. S. (2023). Edge ai: a survey. Internet
of Things and Cyber-Physical Systems, 3:71–92.
Teoh, Y. K., Gill, S. S., and Parlikad, A. K. (2021). Iot and
fog-computing-based predictive maintenance model
for effective asset management in industry 4.0 using
machine learning. IEEE Internet of Things Journal,
10(3):2087–2094.
Vailshery, L. S. (2024). Global iot connections 2030, by
application.
Walia, G. K., Kumar, M., and Gill, S. S. (2024). Ai-
empowered fog/edge resource management for iot ap-
plications: A comprehensive review, research chal-
lenges, and future perspectives. IEEE Communica-
tions Surveys & Tutorials, 26(1):619–669.
Wang, D., Ding, H., Yang, Y., Mi, Z., Liu, L., and Xiong, Z.
(2016). Qos and sla aware web service composition
in cloud environment. KSII Transactions on Internet
and Information Systems (TIIS), 10(12):5231–5248.
Wang, M., Fu, W., He, X., Hao, S., and Wu, X. (2020).
A survey on large-scale machine learning. IEEE
Transactions on Knowledge and Data Engineering,
34(6):2574–2594.
Wang, Y., Gao, J., Cheng, T., Gong, Y., and Wu, T.-H.
(2024). Does blockchain technology need to be intro-
duced in the closed-loop supply chain based on prod-
uct lifecycle management? Computers & Industrial
Engineering, 188:109881.
Wehner, S., Elkouss, D., and Hanson, R. (2018). Quan-
tum internet: A vision for the road ahead. Science,
362(6412):eaam9288.
ICSBT 2024 - 21st International Conference on Smart Business Technologies
14