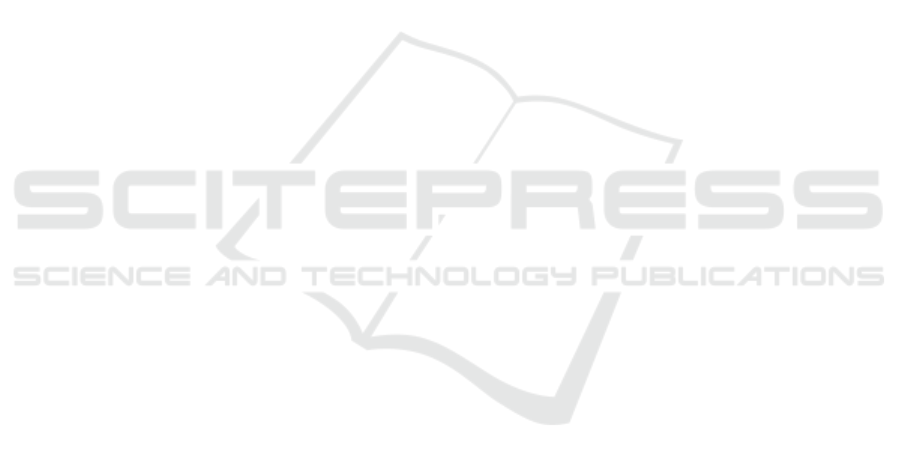
ing the model’s generalization capability. Accord-
ing to experimental results, the D-DMR-FBLS out-
performed other models in terms of accuracy, AUC,
F1-score and G-mean. Besides, its performance sur-
passes the FBLS and TSK fuzzy systems, especially
in scenarios with limited labeled samples. This new
neuro-fuzzy system shows promise for use in contexts
with limited medical resources, assisting the decision-
making for the allocation of medical care to organ
transplant recipients. Future research could further
explore the application of D-DMR-FBLS across other
medical datasets, validating its effectiveness in varied
medical contexts.
ACKNOWLEDGEMENTS
This paper is part of project
PID2022-143299OB-I00, financed by
MCIN/AEI/10.13030/501100011033/FEDER,UE.
REFERENCES
Cao, B., Mao, M., Viidu, S., and Philip, S. Y. (2017). Hit-
fraud: a broad learning approach for collective fraud
detection in heterogeneous information networks. In
2017 IEEE international conference on data mining
(ICDM), pages 769–774. IEEE.
Ershoff, B. D., Lee, C. K., Wray, C. L., Agopian, V. G., Ur-
ban, G., Baldi, P., and Cannesson, M. (2020). Train-
ing and validation of deep neural networks for the pre-
diction of 90-day post-liver transplant mortality using
unos registry data. In Transplantation proceedings,
volume 52, pages 246–258. Elsevier.
Feng, S. and Chen, C. P. (2018). Fuzzy broad learning
system: A novel neuro-fuzzy model for regression
and classification. IEEE transactions on cybernetics,
50(2):414–424.
Feng, S. and Chen, C. P. (2021). Performance analysis of
fuzzy bls using different cluster methods for classifi-
cation. Science China Information Sciences, 64:1–3.
Feng, S., Chen, C. P., Xu, L., and Liu, Z. (2020). On the
accuracy–complexity tradeoff of fuzzy broad learn-
ing system. IEEE Transactions on Fuzzy Systems,
29(10):2963–2974.
Gong, X., Zhang, T., Chen, C. P., and Liu, Z. (2021). Re-
search review for broad learning system: Algorithms,
theory, and applications. IEEE Transactions on Cy-
bernetics, 52(9):8922–8950.
Liu, C.-L., Soong, R.-S., Lee, W.-C., Jiang, G.-W., and Lin,
Y.-C. (2020). predicting short-term survival after liver
transplantation using machine learning. Scientific re-
ports, 10(1):1–10.
Liu, Z., Huang, S., Jin, W., and Mu, Y. (2021). Broad learn-
ing system for semi-supervised learning. Neurocom-
puting, 444:38–47.
Mamode, N., Ahmed, Z., Jones, G., Banga, N., Motalle-
bzadeh, R., Tolley, H., Marks, S., Stojanovic, J., Khur-
ram, M. A., Thuraisingham, R., et al. (2021). Mor-
tality rates in transplant recipients and transplanta-
tion candidates in a high-prevalence covid-19 environ-
ment. Transplantation, 105(1):212–215.
Mark, E., Goldsman, D., Gurbaxani, B., Keskinocak, P., and
Sokol, J. (2023). Predicting a kidney transplant pa-
tient’s pre-transplant functional status based on infor-
mation from waitlist registration. Scientific Reports,
13(1):6164.
Peng, C. and ChunHao, D. (2022). Monitoring multi-
domain batch process state based on fuzzy broad
learning system. Expert Systems with Applications,
187:115851.
Shihabudheen, K. and Pillai, G. N. (2018). Recent advances
in neuro-fuzzy system: A survey. Knowledge-Based
Systems, 152:136–162.
Worldometer (2024). Worldometer - COVID-19 Coro-
navirus Pandemic. https://www.worldometers.info/
coronavirus/. Accessed: 2024-01-10.
Xue, J., Jiang, Y., Wang, L., Sun, Z., and Xing, C. (2018).
Intelligent prediction of renal injury in diabetic kid-
ney disease patients based on a novel unbalanced zero-
order tsk fuzzy system. Journal of Medical Imaging
and Health Informatics, 8(8):1711–1717.
Zhang, L., Li, J., Lu, G., Shen, P., Bennamoun, M., Shah,
S. A. A., Miao, Q., Zhu, G., Li, P., and Lu, X. (2020).
Analysis and variants of broad learning system. IEEE
Transactions on Systems, Man, and Cybernetics: Sys-
tems, 52(1):334–344.
Zhang, X., Gavald
`
a, R., and Baixeries, J. (2022). Inter-
pretable prediction of mortality in liver transplant re-
cipients based on machine learning. Computers in bi-
ology and medicine, 151:106188.
SIMULTECH 2024 - 14th International Conference on Simulation and Modeling Methodologies, Technologies and Applications
422