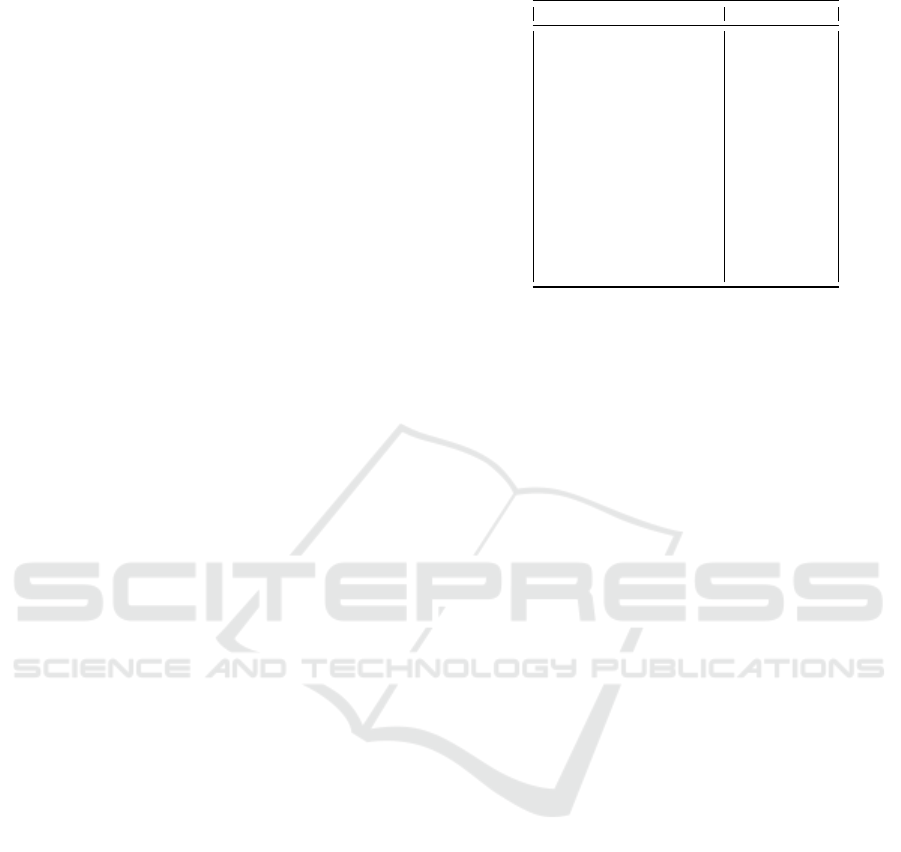
et al., 2021) (De Stefano et al., 2011) (Carchiolo et al.,
2022a) some examples of generating co-authorship
networks are presented with the idea of represent-
ing the collaborations of the authors. In other cases
(Carchiolo et al., 2022b), (Bordons et al., 2015) (Car-
chiolo et al., 2023), the data extracted from Scopus
have been used to analyze the importance of certain
researchers or their performance in terms of specific
indices.
Our analysis, grounded in a robust dataset of sci-
entific publications, maps the distribution of AI re-
search across these diverse disciplines. The data
speaks volumes: AI is not just a tool but a collabo-
rator, opening doors to new realms of knowledge and
understanding.
Section 2 introduces how the dataset was con-
structed, while Section 3 presents the temporal anal-
ysis and thematic distribution of the publications, and
some results are discussed in detail. Section 4 pro-
vides an overview of some of the most cited articles.
We finally consider further works and concluding re-
marks in Section 5.
2 DATASET
The study of scientific output in the field of Arti-
ficial Intelligence (AI) holds immense significance,
given its prominence as a contemporary research fo-
cus that extends beyond the confines of computer sci-
ence. Researchers across diverse disciplines, even
those seemingly distant from computer science, are
increasingly exploring the potential of AI as a vi-
able solution for their respective fields of study. For
this study, to capture the subset of articles focusing
on Artificial Intelligence, a query against the Sco-
pus database was performed using the keyword field.
Instead of using only the keyword ”Artificial Intel-
ligence,” 18 different keywords were chosen, the ta-
ble 1 lists the 18 keywords used and the number of
articles selected for each. With this keyword selec-
tion, a total of 2, 156, 387 articles were selected, of
which 2, 081, 397, about 96.5% were written in En-
glish. This percentage remains relatively constant
over the years; for example, in 2023, it is approxi-
mately 97%.
The analysis of these documents, totaling
2,156,387, reveals that a very small fraction (a few
thousand) are incorrectly categorized and cover a
topic do not related to artificial intelligence that, typ-
ically, pertain to keywords such as ”Pattern Recogni-
tion,” ”Reinforcement Learning,” ”Optimization Al-
gorithms,” and ”Data Analysis”. However, given the
small percentage, they cannot bias our analysis. Some
Table 1: Keywords in Artificial Intelligence.
Keyword Document number
Artificial Intelligence 460 755
Machine Learning 455 294
Deep Learning 348 589
Data Analysis 272 258
Pattern Recognition 227 417
Convolutional Neural Networks 182 998
Computer Vision 180 619
Artificial Neural Networks 154 046
Natural Language Processing 108 584
Optimization Algorithms 80 415
Reinforcement Learning 77 935
Expert Systems 56 364
Supervised Learning 45 794
Recurrent Neural Networks 43 456
Machine Learning Algorithms 17 022
Unsupervised Learning 14 376
Artificial Intelligence Applications 950
Cognitive Robotics 796
of the documents are quite old, dating back further
than expected (see Figure 1.(a)) It should not be sur-
prising to find articles from the early 1950s, as the
term ”AI” was coined by John McCarthy in 1955 dur-
ing a conference at Dartmouth College. McCarthy
and other scholars laid the groundwork for a new
research field aimed at developing machines capa-
ble of learning, reasoning, and problem-solving au-
tonomously. However, traces of AI-related concepts
can be found even before 1955 in the writings of Alan
Turing, Marvin Minsky, who founded the MIT Arti-
ficial Intelligence Laboratory in 1951, one of the pio-
neering research centers in AI, and Arthur Samuel,
who in 1959 developed the ”Gameplay” program,
considered one of the earliest examples of artificial
intelligence applied to a game, specifically checkers.
Nevertheless, in recent decades, due to technologi-
cal advancements, the availability of large amounts
of data, and improvements in algorithms, AI has ex-
perienced a resurgence and has begun to influence an
increasing number of societal sectors.
The initial analyses presented in this paper aimed
to delineate the sectors to which the publications
could be attributed. Scopus organizes its database by
assigning a ”Subject Area” to each publication based
on the publication venue. As depicted in Figure 1.(b),
it is evident that slightly over 50% of the publications
are categorized under Computer Science, a proportion
that has varied between 45% and 60% over the years.
This observation underscores the dominant presence
of Computer Science within the dataset.
Furthermore, we conducted an analysis of the
”Subject Areas” in which Scopus classifies docu-
ments, the table 2 lists all the ”Subject Areas” present
in Scopus, along with the number of publications se-
lected by us attributed to each area. In our case, the
documents are divided into Computer Science and
Engineering for more than 80% of the cases. More-
over, it can be appreciated that the most relevant ap-
plication fields in table 2
DATA 2024 - 13th International Conference on Data Science, Technology and Applications
578