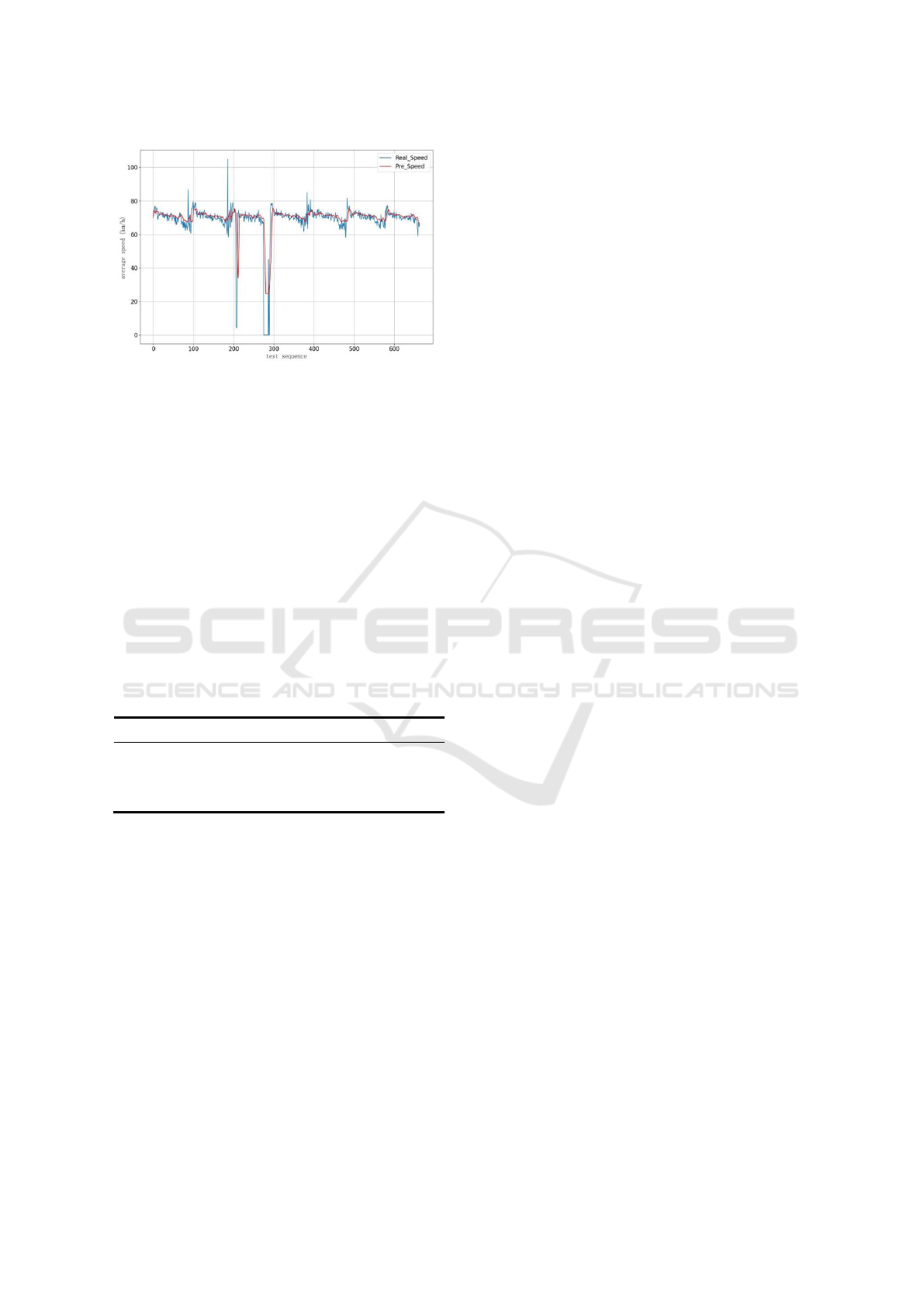
Figure 9: The speed prediction results for the " M25/7108B
priority 1 on link 200045820" (Original).
Following the predictive results of the LSTM
model, an error analysis was performed, and Table 6
presents the results of this analysis. According to the
error analysis, the MSE, RMSE, and MAE values for
both traffic volume and average vehicle speed
predictions were relatively small. However, an
anomaly was observed in the testing sequence from
200 to 300 in Fig. 8 and Fig. 9, where the average
vehicle speed dropped to 0. This anomaly may be
related to the actual road conditions. Excluding this
abnormal portion, the fit was generally good in the
remaining intervals, indicating satisfactory predictive
results.
Table 6: Error analysis for" M25/7108B priority 1 on link
200045820".
From the predictive results, it is observed that the
traffic volume exhibits roughly multi-modal peaks
daily, with overall higher values from 6:00 AM to
9:00 PM. The average vehicle speed, except for the
abnormal interval, remains relatively stable,
fluctuating around 70 km/h daily.
From the above predictive analyses of the three
sites, it can be observed that the sites with the
maximum traffic volume and the fastest average
vehicle speed exhibit similar trends in their
predictions. Both traffic volume and average vehicle
speed show a daily pattern of roughly bimodal peaks
and unimodal minima, likely influenced by peak
commuting hours. The overall higher traffic volume
from 6:00 AM to 9:00 PM and lower average vehicle
speed from 7:00 AM to 8:00 PM may be associated
with increased daytime airport operations, with more
flights departing and arriving, and fewer runway
maintenance activities during the night.
For the site located at the highway ramp, daily
traffic volume displays a multi-modal peak pattern,
with overall higher values from 6:00 AM to 9:00 PM.
This pattern is likely influenced by both peak
commuting hours and the airport flight schedule.
Apart from the abnormal interval where the vehicle
speed is zero, the average vehicle speed remains
relatively stable throughout the day, approximately at
70 km/h. This may be related to the speed limits
specified for the highway ramp.
These findings suggest that while there are subtle
differences in the daily variations of traffic volume
and average vehicle speed, the overall trend of higher
traffic volume corresponding to slower average
vehicle speed is consistent.
The fact that the model's predictive results align
with the observed patterns indicates its effectiveness
in capturing the general traffic flow around Heathrow
Airport. This reflects the preliminary correctness of
choosing the LSTM model for passenger flow
prediction. However, the selection of sites is still not
sufficiently representative. In the follow-up, it is
advisable to combine the Random Forest model to
assess the representativeness of each site.
4 CONCLUSION
This research involves the prediction and analysis of
traffic flow and average vehicle speeds on seven sites
along the M25 motorway near Heathrow Airport in
August 2019, using the Long Short-Term Memory
(LSTM) model in deep learning. The data
preprocessing phase includes the removal of
irrelevant data and the handling of missing values. In
the initial analysis stage, features in the temporal
dimension at respective sites on the M25 motorway
were extracted and analyzed. It was observed that
both traffic volume and average vehicle speed
exhibited periodic peaks and troughs, with daily
trends and patterns remaining generally consistent.
Three representative sites, namely "M25/4936A
priority 1 on link 200045641," "M25/4883A priority
1 on link 199131002," and "M25/7108B priority 1 on
link 200045820," were selected for LSTM prediction
analysis. Following preprocessing and initial
analysis, a 2-layer LSTM model with 80 neurons per
layer was created, using a prediction interval of 5 5-
time steps. According to the results of the prediction
analysis, excluding road anomalies, the LSTM model
effectively predicts traffic flow on the highway
ICDSE 2024 - International Conference on Data Science and Engineering
392