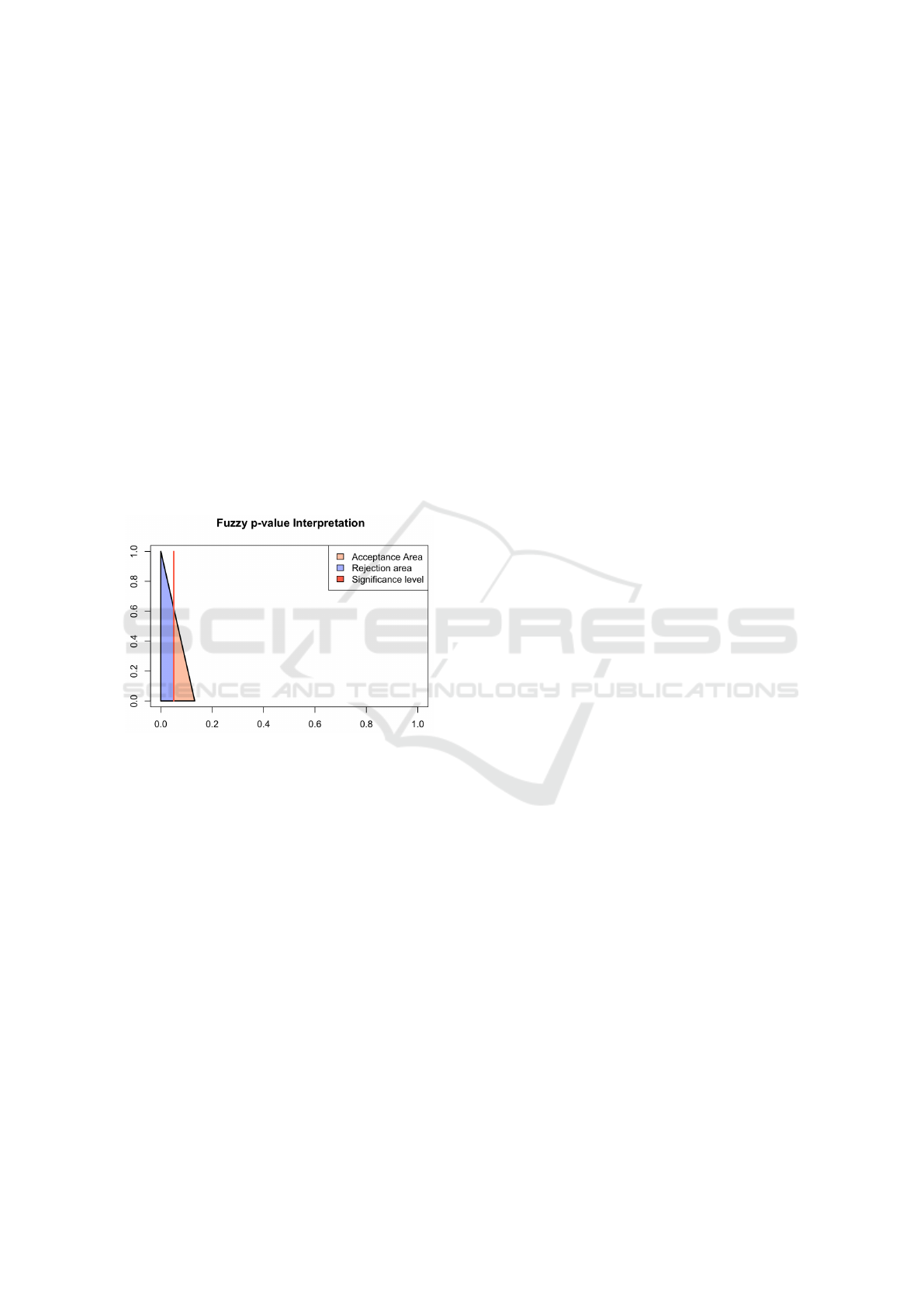
4, 5 show us how different shapes for the null hy-
pothesis yield to different non binary values r
re j
and
r
acc
. At last, Table 3 also depicts how shifting null
hypotheses from (2.25, 2.35, 2.40) to (2.5, 2.55, 2.6)
gives values r
acc
gradually going from 0 to 1 and val-
ues r
re j
gradually going from 1 to 0. Table 4 illus-
trates the same results, but this time for the opposite
side,
˜
H
0
: θ >
˜
θ
0
against
˜
H
1
: θ ≤
˜
θ
0
. Finally, for the
two-sided test illustrated by Table 5, we again observe
the same behaviour. Test 6 yields values r
acc
= 0 and
r
re j
= 1. As opposed to it, test 1 gives values r
acc
= 1
and r
re j
= 0. Tests 2, 3, 4, 5 yield non binary values
for r
acc
and r
re j
. As a whole, we observe that r
acc
gradually goes from 1 to 0 while r
re j
gradually goes
from 0 to 1 when considering the tests 1, 2, 3, 4, 5,
6. This allows us to empirically discover the fuzzy re-
gion in between (2.35,2.4,2.45) and (2.45,2.5,2.5)
where the null hypothesis is starting to be more and
more rejected, respectively less and less rejected de-
pending on the null hypothesis shape.
Figure 7: Crispier fuzzy p-value ˜p = (0, 0,0.132). r
acc
=
0.39 and r
re j
= 0.61.
6 CONCLUSION
In this paper, we introduced a new procedure to
find fuzzy p-values based on precedent works, which
generalises the computation of crisp p-values. Our
method revolves around generating a centred fuzzy
bootstrapped statistic distribution to test and count
how many of these bootstrapped observations are
greater than an observed statistic. Then, we explained
how the obtained fuzzy p-values could be interpreted
as a ratio of a rejection or acceptance area over the to-
tal area formed by the fuzzy p-value. We then enun-
ciated a fuzzy hypothesis testing procedure to be able
to compare fuzzy p-values to results obtained via this
testing procedure. The main takeaway from this com-
parison is that fuzzy p-values tend to be very impre-
cise, with the observations getting fuzzier. However,
it is still a helpful tool when the observations become
crisper. Indeed, in the latter scenario, fuzzy p-values
give us an idea of how much we’re inside the fuzzy
confidence interval or how much we’re outside of it.
In extreme cases, fuzzy p-values give the same binary
results coming from crisp p-values.
REFERENCES
Arefi, M., Viertl, R., and Taheri, S. M. (2012). Fuzzy den-
sity estimation. Metrika, 75(1):5–22.
Berkachy, R. and Donz
´
e, L. (2017). Testing Fuzzy Hy-
potheses with Fuzzy Data and Defuzzification of the
Fuzzy p-value by the Signed Distance Method. Pro-
ceedings of the 9th International Joint Conference on
Computational Intelligence, pages 255–264.
Berkachy, R. and Donz
´
e, L. (2020). Fuzzy Confi-
dence Intervals by the Likelihood Ratio with Boot-
strapped Distribution. Proceedings of the 12th Inter-
national Joint Conference on Computational Intelli-
gence, pages 231–242.
Berkachy, R. and Donz
´
e, L. (2022). Fuzzy Confidence In-
tervals by the Likelihood Ratio: Testing Equality of
Means—Application on Swiss SILC Data. SN Com-
puter Science, 3(5):374.
Chachi, J. and Taheri, S. M. (2011). Fuzzy confidence in-
tervals for mean of Gaussian fuzzy random variables.
Expert Systems with Applications, 38(5):5240–5244.
Grzegorzewski, P. and Romaniuk, M. (2021). Epistemic
Bootstrap for Fuzzy Data. Joint Proceedings of
the 19th World Congress of the International Fuzzy
Systems Association (IFSA), the 12th Conference of
the European Society for Fuzzy Logic and Technol-
ogy (EUSFLAT), and the 11th International Sum-
mer School on Aggregation Operators (AGOP), pages
538–545.
Hryniewicz, O. (2018). Statistical properties of the fuzzy p-
value. International Journal of Approximate Reason-
ing, 93(J. Am. Stat. Assoc. 95 452 2000):544–560.
Kahraman, C., Otay, I., and
¨
Oztays¸i, B. (2016). Fuzzy Ex-
tensions of Confidence Intervals: Estimation for µ, σ
2
,
and p, pages 129–154. Springer International Publish-
ing, Cham.
Kahraman, C.,
¨
Oztays¸i, B., and Onar, S. C. (2019).
Interval-Valued Intuitionistic Fuzzy Confidence Inter-
vals. Journal of Intelligent Systems, 28(2):307–319.
Kruse, R. and Meyer, K. D. (1987). Statistics with Vague
Data. D. Reidel Publishing Company.
Parchami, A. (2020). Fuzzy decision in testing hypotheses
by fuzzy data: Two case studies. Iranian Journal of
Fuzzy Systems.
Parchami, A., Taheri, S. M., and Mashinchi, M. (2010).
Fuzzy p-value in testing fuzzy hypotheses with crisp
data. Springer Stat Papers, 51:209–226.
Rosset, J. and Donz
´
e, L. (2024). New decision rules for
fuzzy statistical inferences. In Ansari, J., Fuchs, S.,
Trutschnig, W., Lubiano, M. A., Gil, M.
´
A., Grze-
gorzewski, P., and Hryniewicz, O., editors, Combin-
ing, Modelling and Analyzing Imprecision, Random-
Approximated Fuzzy p-values by Bootstrapped Fuzzy Distributions and Fuzzy Hypotheses Testing
393