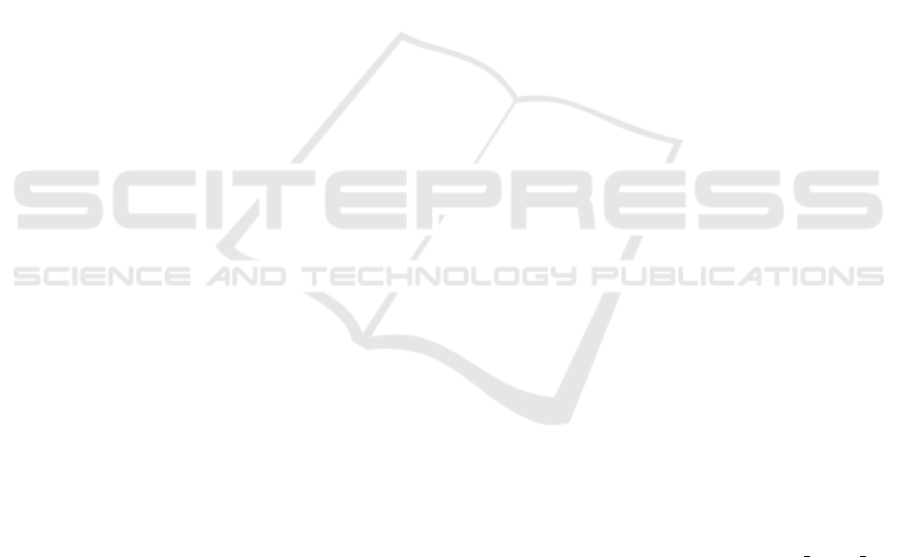
In this paper it was shown that a suitable low pass
filter frequency is f
c
= 0.11Hz. The offset error sig-
nal was decomposed into half-waves, which account
for the full drift and compensate snippets. It has been
shown, that the total length of these snippets is about
80% of the full data length, proving the generality of
the filtering and cutting. Secondly, the intervention
point when the driver switches from the drift to the
compensation phase was calculated. The offset error
extrema associated with the intervention are between
0.05m and 0.15m for most of the drivers. Thirdly,
the drift and compensation phases have been mod-
elled using an event-based Model Predictive Control
(MPC), with different weights for the two phases. The
values of these weights were calculated by optimis-
ing the model output against the drivers’ measure-
ments. Finally, it has been demonstrated that the pro-
posed driver model together with the MPC can ac-
curately reproduce the compensation path of the se-
lected drivers.
These results provide better understanding of the
drivers’ path following behaviour. Usually, the re-
sulting vehicle path can be measured (e.g., with ac-
curate GPS), which is the result of the driver’s de-
tection, perception, planning and control behaviour,
together. The environmental inputs, such as the po-
sition of the road edge or other objects, can also be
measured. However, the layers in between cannot be
observed properly from a single sequence of data. By
modelling the drift-away and compensation path, the
vehicle level compensation can be filtered out of the
measured data sequence. This enables the estimation
of the planned lane offset, and contributes to a more
accurate validation of driver models at the path plan-
ning level.
The drift-away and compensation model also con-
tributes to the design of a lane-keeping system with
human-like characteristics. Although the underly-
ing controllers are usually designed to give the best
path accuracy, another possibility is to mimic human
steering characteristics. This can contribute to an in-
creased level of trust in such driving systems. The
results of this paper give important inputs to achieve
more human-like lane following behaviour, on the
other hand requires further analysis with the inclusion
of other domains, such as path planning and actuator
control. We believe that an interesting next research
topic is to build predictive driver models, that enable
the intervention point and the compensation dynam-
ics to be calculated at runtime. It would also be an
exciting challenge to analyse the model parameters
for each driver and find ways to cluster them based
on these parameters.
While the results presented in this paper are
promising and validate the concept on a sample
dataset, these results are limited in their applicabil-
ity. Firstly, the number of drivers tested is limited by
the size of the dataset. Therefore, it is not yet proven
whether the concept is generic to a wider range of
drivers. Secondly, the results are generated by simu-
lation and vehicle control algorithms, such as MPCs,
are very sensitive to real-world conditions (e.g. ac-
curacy of the vehicle model). This may hinder the
realisation of the proposed algorithm.
ACKNOWLEDGEMENT
The research was supported by the European Union
within the framework of the National Laboratory for
Autonomous Systems. (RRF-2.3.1-21-2022-00002).
REFERENCES
Cody, D. and Gordon, T. (2007). Trb workshop on driver
models: A step towards a comprehensive model of
driving? Modelling Driver Behaviour in Automotive
Environments, 1(1):26–42.
Conlter, R. C. (1992). Implementation of the pure pursuit
path tracking algorithm.
D Salvucci, D. and Gray, R. (2004). A two-point visual
control model of steering. Perception, 33(1):1233–
1248.
Erno, H., Csaba, H., and Peter, K. (2019). Novel pure-
pursuit trajectory following approaches and their prac-
tical applications. In Proceedings of the 10th IEEE
International Conference on Cognitive Infocommuni-
cations, pages 1–6, Naples, Italy.
Evans, L. and Schwig, R. C. (1985). Human behavior and
traffic safety. Plenum Press, New York, NY.
Hess, R. and Modjtahedzadeh, A. (1990). A control theo-
retic model of driver steering behavior. IEEE Control
Systems Magazine, 10(5):3–8.
Ign
´
eczi, G. and Horv
´
ath, E. (19-21, September 2024).
Human-like behaviour for automated vehicles
(hlb4av) naturalistic driving dataset, data available at
https://jkk-research.github.io/dataset/jkk dataset 03/.
In IEEE 22nd International Symposium on Intelligent
Systems and Informatics (SISY 2024), Pula, Croatia.
Jiang, H., Tian, H., and Hua, Y. (2019). Model predictive
driver model considering the steering characteristics
of the skilled drivers. Advances in Mechanical Engi-
neering, 11(3):1–14.
Katriniok, A., Maschuw, J. P., et al. (2013). Optimal vehi-
cle dynamics control for combined longitudinal and
lateral autonomous vehicle guidance. In Proceed-
ings of European Control Conference, pages 974–979,
Z
¨
urich, Switzerland.
Klebersberg, D. (1971). Subjektive und objektive sicherheit
im strassenverkehr als aufgabe f
¨
ur die verkehrssicher-
Analysis of Drivers’ Path Follow Behaviour
99