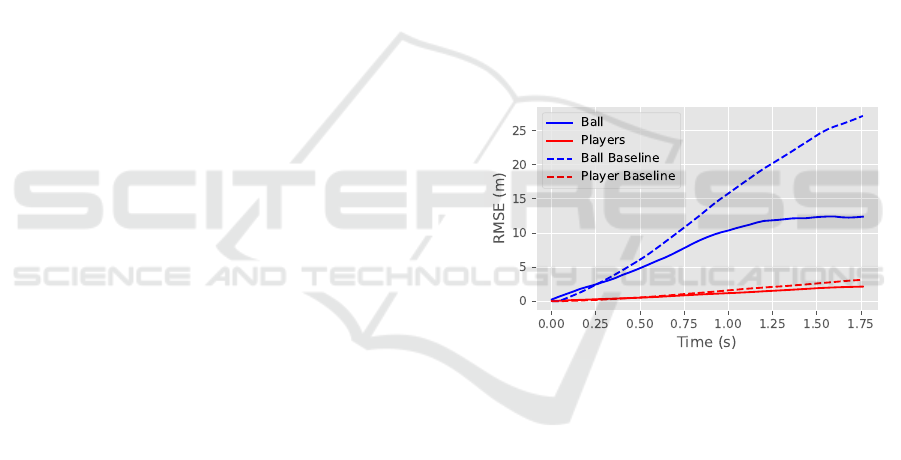
D., Czarnecki, W. M., Tassa, Y., Omidshafiei, S., Ab-
dolmaleki, A., et al. (2021). From motor control
to team play in simulated humanoid football. arXiv
preprint arXiv:2105.12196.
Miller, A., Bornn, L., Adams, R., and Goldsberry, K.
(2014). Factorized point process intensities: A spa-
tial analysis of professional basketball. In Xing, E. P.
and Jebara, T., editors, Proceedings of the 31st Inter-
national Conference on Machine Learning, volume 32
of Proceedings of Machine Learning Research, pages
235–243, Bejing, China. PMLR.
Omidshafiei, S., Hennes, D., Garnelo, M., Wang, Z., Re-
casens, A., Tarassov, E., Yang, Y., Elie, R., Con-
nor, J. T., Muller, P., et al. (2022). Multiagent off-
screen behavior prediction in football. Scientific re-
ports, 12(1):8638.
Tang, B., Zhong, Y., Neumann, U., Wang, G., Chen, S., and
Zhang, Y. (2021). Collaborative uncertainty in multi-
agent trajectory forecasting. Advances in Neural In-
formation Processing Systems, 34:6328–6340.
Teranishi, M., Tsutsui, K., Takeda, K., and Fujii, K. (2022).
Evaluation of creating scoring opportunities for team-
mates in soccer via trajectory prediction. In Interna-
tional Workshop on Machine Learning and Data Min-
ing for Sports Analytics, pages 53–73. Springer.
Vaswani, A., Shazeer, N., Parmar, N., Uszkoreit, J., Jones,
L., Gomez, A. N., Kaiser, Ł., and Polosukhin, I.
(2017). Attention is all you need. Advances in neural
information processing systems, 30.
Wang, Z., Veli
ˇ
ckovi
´
c, P., Hennes, D., Tomašev, N.,
Prince, L., Kaisers, M., Bachrach, Y., Elie, R., Wen-
liang, L. K., Piccinini, F., et al. (2023). Tacticai:
an ai assistant for football tactics. arXiv preprint
arXiv:2310.10553.
Wu, G., Zhao, S., Lin, J., and Silva, C. (2021). Basketball
gan: Sportingly acceptable trajectory prediction.
Yu, C., Yang, X., Gao, J., Chen, J., Li, Y., Liu, J., Xi-
ang, Y., Huang, R., Yang, H., Wu, Y., and Wang,
Y. (2023). Asynchronous multi-agent reinforcement
learning for efficient real-time multi-robot coopera-
tive exploration. In Proceedings of the 2023 Interna-
tional Conference on Autonomous Agents and Multia-
gent Systems, AAMAS ’23, page 1107–1115, Rich-
land, SC. International Foundation for Autonomous
Agents and Multiagent Systems.
Yuan, Y., Makoviychuk, V., Guo, Y., Fidler, S., Peng, X.,
and Fatahalian, K. (2023). Learning physically simu-
lated tennis skills from broadcast videos. ACM Trans.
Graph, 42(4).
Yue, Y., Lucey, P., Carr, P., Bialkowski, A., and Matthews,
I. (2014). Learning fine-grained spatial models for
dynamic sports play prediction. In 2014 IEEE inter-
national conference on data mining, pages 670–679.
IEEE.
Zhan, E., Zheng, S., Yue, Y., Sha, L., and Lucey, P. (2019).
Generating multi-agent trajectories using program-
matic weak supervision. In International Conference
on Learning Representations.
Zhao, Z., Chai, W., Hao, S., Hu, W., Wang, G., Cao, S.,
Song, M., Hwang, J.-N., and Wang, G. (2023). A
survey of deep learning in sports applications: Per-
ception, comprehension, and decision. arXiv preprint
arXiv:2307.03353.
Zheng, S., Yue, Y., and Hobbs, J. (2016). Generating long-
term trajectories using deep hierarchical networks.
Advances in Neural Information Processing Systems,
29.
APPENDIX
Prediction Error Versus Time. Figure 18 shows the
results from 200 simulations initialized from a ran-
dom point in a random rally. The simulations are
evolved for 1.75 seconds and the RMSE is plotted
compared with the ground truth data for the ball and
players. The baseline is taken as a linear extrapolation
of the velocity of the player and ball frozen at the time
the simulation begins. Our simulation performs better
than a linear extrapolation over a short time, indicat-
ing it has learned how to sensibly predict and update
the state vectors as a function of time.
Figure 18: Root Mean Squared Error (RMSE) compared
to real tennis data as a function of time, for both ball and
player positions when simulating forward from a random
in a rally. SportsNGEN performs better than a baseline of
linear extrapolation.
icSPORTS 2024 - 12th International Conference on Sport Sciences Research and Technology Support
130