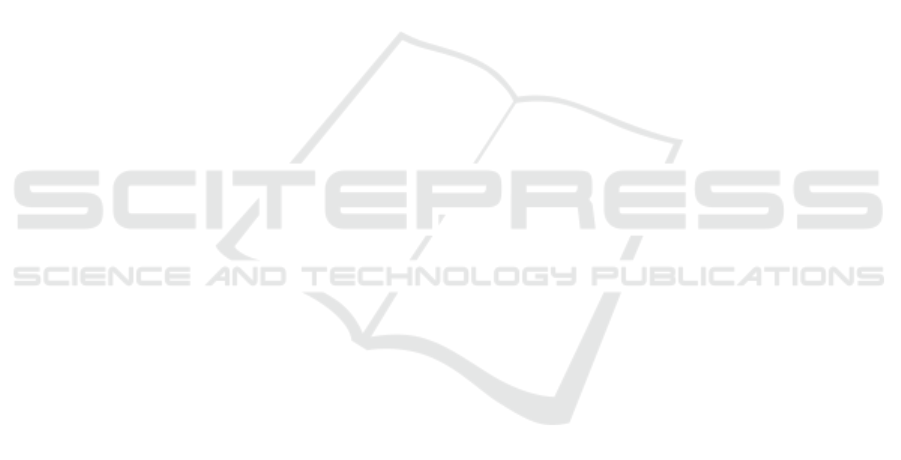
REFERENCES
Abbas, W., Zhang, Z., Asim, M., Chen, J., and Ahmad,
S. (2024). Ai-driven precision clothing classification:
Revolutionizing online fashion retailing with hybrid
two-objective learning. Information, 15(4):196.
Abd Alaziz, H. M., Elmannai, H., Saleh, H., Hadjouni, M.,
Anter, A. M., Koura, A., and Kayed, M. (2023). En-
hancing fashion classification with vision transformer
(vit) and developing recommendation fashion systems
using dinova2. Electronics, 12(20):4263.
Achiam, J., Adler, S., Agarwal, S., Ahmad, L., Akkaya, I.,
Aleman, F. L., Almeida, D., Altenschmidt, J., Altman,
S., Anadkat, S., et al. (2023). Gpt-4 technical report.
arXiv preprint arXiv:2303.08774.
Al-Rawi, M. and Beel, J. (2020). Towards an interopera-
ble data protocol aimed at linking the fashion industry
with ai companies. arXiv preprint arXiv:2009.03005.
Chen, Y.-C., Li, L., Yu, L., El Kholy, A., Ahmed, F., Gan,
Z., Cheng, Y., and Liu, J. (2020). Uniter: Universal
image-text representation learning. In European con-
ference on computer vision, pages 104–120. Springer.
Dosovitskiy, A., Beyer, L., Kolesnikov, A., Weissenborn,
D., Zhai, X., Unterthiner, T., Dehghani, M., Minderer,
M., Heigold, G., Gelly, S., et al. (2020). An image is
worth 16x16 words: Transformers for image recogni-
tion at scale. arXiv preprint arXiv:2010.11929.
Ferreira, B. Q., Costeira, J. P., and Gomes, J. P. (2021). Ex-
plainable noisy label flipping for multi-label fashion
image classification. In Proceedings of the IEEE/CVF
Conference on Computer Vision and Pattern Recogni-
tion, pages 3916–3920.
Guo, S., Huang, W., Zhang, X., Srikhanta, P., Cui, Y., Li, Y.,
Adam, H., Scott, M. R., and Belongie, S. (2019a). The
imaterialist fashion attribute dataset. In Proceedings
of the IEEE/CVF International Conference on Com-
puter Vision Workshops, pages 0–0.
Guo, W., Wang, J., and Wang, S. (2019b). Deep multi-
modal representation learning: A survey. Ieee Access,
7:63373–63394.
He, K., Zhang, X., Ren, S., and Sun, J. (2016). Deep resid-
ual learning for image recognition. In Proceedings of
the IEEE conference on computer vision and pattern
recognition, pages 770–778.
Inoue, N., Simo-Serra, E., Yamasaki, T., and Ishikawa,
H. (2017). Multi-label fashion image classification
with minimal human supervision. In Proceedings of
the IEEE international conference on computer vision
workshops, pages 2261–2267.
Kadam, S. and Vaidya, V. (2020). Review and analysis of
zero, one and few shot learning approaches. In In-
telligent Systems Design and Applications: 18th In-
ternational Conference on Intelligent Systems Design
and Applications (ISDA 2018) held in Vellore, In-
dia, December 6-8, 2018, Volume 1, pages 100–112.
Springer.
Kolisnik, B., Hogan, I., and Zulkernine, F. (2021).
Condition-cnn: A hierarchical multi-label fashion im-
age classification model. Expert Systems with Appli-
cations, 182:115195.
Koonce, B. and Koonce, B. (2021). Resnet 50. Convolu-
tional neural networks with swift for tensorflow: im-
age recognition and dataset categorization, pages 63–
72.
Krizhevsky, A., Sutskever, I., and Hinton, G. E. (2017). Im-
agenet classification with deep convolutional neural
networks. Communications of the ACM, 60(6):84–90.
LeCun, Y., Bottou, L., Bengio, Y., and Haffner, P. (1998).
Gradient-based learning applied to document recogni-
tion. Proceedings of the IEEE, 86(11):2278–2324.
Lu, J., Batra, D., Parikh, D., and Lee, S. (2019). Vil-
bert: Pretraining task-agnostic visiolinguistic repre-
sentations for vision-and-language tasks. Advances in
neural information processing systems, 32.
Mallavarapu, T., Cranfill, L., Kim, E. H., Parizi, R. M.,
Morris, J., and Son, J. (2021). A federated approach
for fine-grained classification of fashion apparel. Ma-
chine Learning with Applications, 6:100118.
Ngiam, J., Khosla, A., Kim, M., Nam, J., Lee, H., and Ng,
A. Y. (2011). Multimodal deep learning. In Proceed-
ings of the 28th international conference on machine
learning (ICML-11), pages 689–696.
Q. Ferreira, B. Costeira, J. R. G., Gui, L.-Y., and Gomes,
J. P. (2019). Pose guided attention for multi-label
fashion image classification. In Proceedings of the
IEEE/CVF International Conference on Computer Vi-
sion Workshops, pages 0–0.
Radford, A., Kim, J. W., Hallacy, C., Ramesh, A., Goh, G.,
Agarwal, S., Sastry, G., Askell, A., Mishkin, P., Clark,
J., et al. (2021). Learning transferable visual models
from natural language supervision. In International
conference on machine learning, pages 8748–8763.
PMLR.
Raffel, C., Shazeer, N., Roberts, A., Lee, K., Narang, S.,
Matena, M., Zhou, Y., Li, W., and Liu, P. J. (2020).
Exploring the limits of transfer learning with a unified
text-to-text transformer. Journal of machine learning
research, 21(140):1–67.
Saranya, M. and Geetha, P. (2022). Fashion image clas-
sification using deep convolution neural network. In
International Conference on Computer, Communica-
tion, and Signal Processing, pages 116–127. Springer.
Seo, Y. and Shin, K.-s. (2019). Hierarchical convolutional
neural networks for fashion image classification. Ex-
pert systems with applications, 116:328–339.
Szegedy, C., Liu, W., Jia, Y., Sermanet, P., Reed, S.,
Anguelov, D., Erhan, D., Vanhoucke, V., and Rabi-
novich, A. (2015). Going deeper with convolutions.
In Proceedings of the IEEE conference on computer
vision and pattern recognition, pages 1–9.
Xhaferra, E., Cina, E., and Toti, L. (2022). Classification
of standard fashion mnist dataset using deep learning
based cnn algorithms. In 2022 International Sym-
posium on Multidisciplinary Studies and Innovative
Technologies (ISMSIT), pages 494–498. IEEE.
Zhong, S., Ribul, M., Cho, Y., and Obrist, M. (2023).
Textilenet: A material taxonomy-based fashion textile
dataset. arXiv preprint arXiv:2301.06160.
Multi-Label Classification for Fashion Data: Zero-Shot Classifiers via Few-Shot Learning on Large Language Models
257