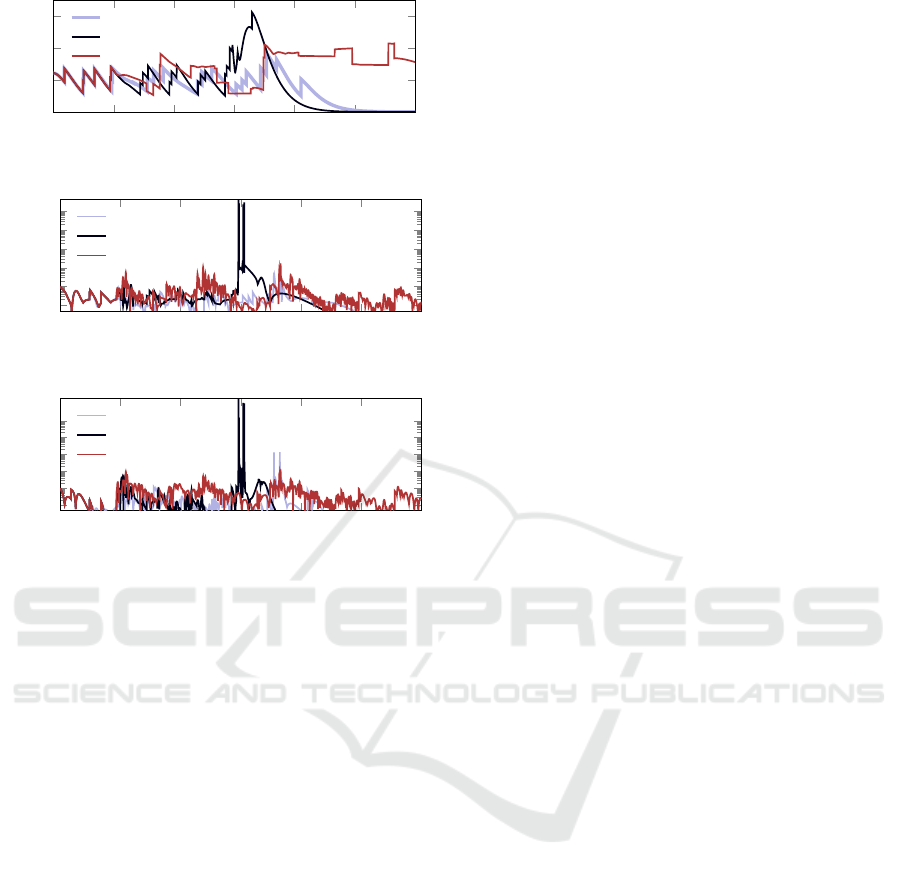
0
5
10
15
20
25
30
0
10
20
30
t (s)
∑
N
i=1
||z
i
−z
d
i
|| (m)
Proposed Control
Constant ∆
i j
Constant r
i j
& ∆
i j
Figure 7: Cumulative tracking error.
0
5
10
15
20
25
30
10
0
10
1
10
2
10
3
10
4
10
5
t (s)
∑
N
i=1
||f
i
|| (m)
Proposed Control
Constant ∆
i j
Constant r
i j
& ∆
i j
Figure 8: Cumulative linear force (in logarithm scale).
0
5
10
15
20
25
30
10
0
10
1
10
2
10
3
10
4
10
5
t (s)
∑
N
i=1
||τ
i
|| (m)
Proposed Control
Constant ∆
i j
Constant r
i j
& ∆
i j
Figure 9: Cumulative torque (in logarithm scale).
minimum safe distance that agents need to enforce,
taking into consideration the shape and orientation
of vehicles and obstacles and, therefore, reducing
the conservatism introduced by traditional APF-based
methods. Furthermore, the framework modulates the
avoidance maneuvers and reaction forces based on
the collision threat level that obstacles might repre-
sent. The synthesis of both approaches is a contin-
uously smooth and bounded control force provided
in analytical closed-form. Simulation results demon-
strated that the proposed avoidance control can in-
crease the maneuverability of vehicles through highly
constrained spaces when compared to the use of con-
stant minimum safe distance and reaction radii.
REFERENCES
Braquet, M. and Bakolas, E. (2022). Vector field-based
collision avoidance for moving obstacles with time-
varying elliptical shape. In Proc. AACC MECC, pages
587–592.
Brockett, R. W. (1983). Asymptotic stability and feedback
stabilization. In Brockett, R. W., Millman, R. S., and
Sussmann, H. J., editors, Differential Geometric Con-
trol Theory, pages 181–191. Birkhauser, Boston.
Du, Y., Zhang, X., and Nie, Z. (2019). A real-time colli-
sion avoidance strategy in dynamic airspace based on
dynamic artificial potential field algorithm. IEEE Ac-
cess, 7:169469–169479.
Gao, Y., Li, D., et al. (2024). Trajectory planning and
tracking control of autonomous vehicles based on im-
proved artificial potential field. IEEE Trans. Veh.
Technol., pages 1–16.
Ginesi, M., Meli, D., et al. (2021). Dynamic movement
primitives: Volumetric obstacle avoidance using dy-
namic potential functions. J. Intell. Robot. Syst.,
101:1–20.
Hoy, M., Matveev, A. S., and Savkin, A. V. (2014). Algo-
rithms for collision-free navigation of mobile robots in
complex cluttered environments: A survey. Robotica,
33(3):463–497.
Khalil, H. K. (2002). Nonlinear Systems. Prentice Hall,
New Jersey.
Khatib, O. (1986). Real-time obstacle avoidance for manip-
ulators and mobile robots. Int. J. Robot. Res., 5(1):90–
98.
Melchiorre, M., Scimmi, L. S., et al. (2022). Robot collision
avoidance based on artificial potential field with local
attractors. In Proc. ICINCO, pages 340–350.
Raibail, M., Rahman, A. H. A., et al. (2022). Decentralized
multi-robot collision avoidance: A systematic review
from 2015 to 2021. Symmetry, 14(3).
Rodr
´
ıguez-Seda, E. J. (2024a). Decentralized low-energy
avoidance control framework for multiple mobile
agents using irregular observations. IEEE Trans. Con-
trol Syst. Technol., 32(3):1027–1039.
Rodr
´
ıguez-Seda, E. J. (2024b). Reactive collision avoid-
ance control for nonholonomic vehicles and obstacles
of arbitrary shape. ASME. Letters Dyn. Sys. Control,
4(3):031005.
Rodr
´
ıguez-Seda, E. J., Stipanovi
´
c, D. M., and Spong,
M. W. (2016). Guaranteed collision avoidance for
autonomous systems with acceleration constraints
and sensing uncertainties. J. Optim. Theory Appl.,
168(3):1014–1038.
Rodr
´
ıguez-Seda, E. J. and Stipanovi
´
c, D. M. (2020). Coop-
erative avoidance control with velocity-based detec-
tion regions. IEEE Control Syst. Lett., 4(2):432–437.
Rodr
´
ıguez-Seda, E. J., Tang, C., et al. (2014). Trajec-
tory tracking with collision avoidance for nonholo-
nomic vehicles with acceleration constraints and lim-
ited sensing. Int. J. Robot. Res., 33(12):1569–1592.
Stipanovi
´
c, D. M., Hokayem, P. F., et al. (2007). Coopera-
tive avoidance control for multiagent systems. J. Dyn.
Syst. Meas. Control, 129:699–707.
Stipanovi
´
c, D. M., Tomlin, C. J., and Leitmann, G. (2012).
Monotone approximations of minimum and maxi-
mum functions and multi-objective problems. Appl.
Math. Optim., 66(3):455–473.
Xiao, X., Liu, B., et al. (2021). Toward agile maneuvers in
highly constrained spaces: Learning from hallucina-
tion. IEEE Rob. Autom. Lett., 6(2):1503–1510.
Zhang, W., Rodr
´
ıguez-Seda, E. J., et al. (2020). Avoid-
ance control with relative velocity information for la-
grangian dynamics. J. Intell. Robot. Syst., 99:229–
244.
Zimmermann, S., Busenhart, M., et al. (2022). Differen-
tiable collision avoidance using collision primitives.
In Proc. IEEE/RSJ Int. Conf. Intell. Robots Syst.,
pages 8086–8093.
ICINCO 2024 - 21st International Conference on Informatics in Control, Automation and Robotics
236