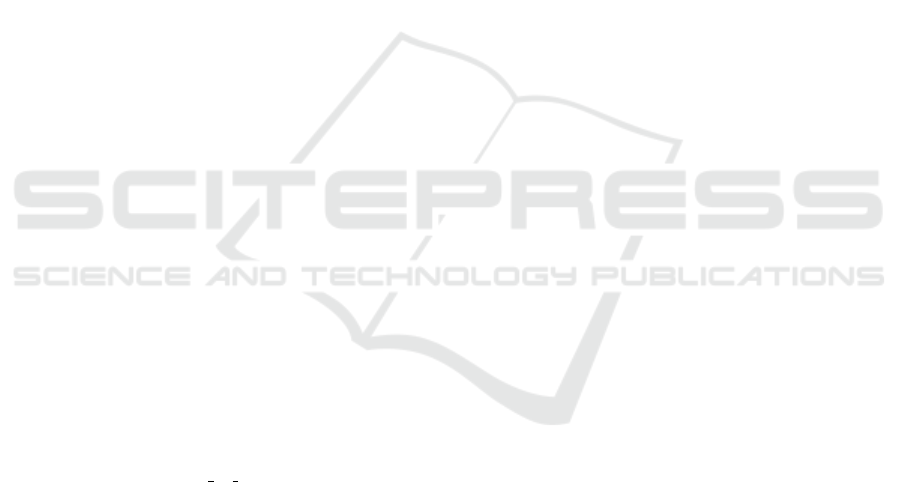
REFERENCES
Adamic, L. A. and Huberman, B. A. (2000a). The
nature of markets in the world wide web.
https://ssrn.com/abstract=166108.
Adamic, L. A. and Huberman, B. A. (2000b). Power-
law distribution of the world wide web. Science,
287(5461):2115–2115.
Agarwal, V. and Sastry, N. (2022). “way back then”: A
data-driven view of 25+ years of web evolution. In
Proceedings of the ACM Web Conference 2022, pages
3471–3479.
Cannon, R. (2008). More people are using
google to navigate, not just search [data].
https://www.searchenginejournal.com/is-google-
trumping-the-url/6820. Acessed on 2024-02-29.
CERN (1993). Software release of www into public do-
main. https://cds.cern.ch/record/1164399.
Chakrabarti, S., Joshi, M. M., Punera, K., and Pennock,
D. M. (2002). The structure of broad topics on the
web. In Proceedings of the 11th International Confer-
ence on World Wide Web, pages 251–262.
Constine, J. (2013). Quora signals it’s favoring search ads
for eventual monetization, launches author stats tool.
http://techcrunch.com/2013/11/12/quora-confirms-
its-favoring-search-ads-for-eventual-monetization-
launches-author-stats-tool. Acessed on 2024-04-10.
Crichton, K., Christin, N., and Cranor, L. F. (2021). How do
home computer users browse the web? ACM Trans.
Web, 16(1).
Diachuk, O., Loba, P., and Mirgorodskaya, O. (2021).
Comparing accuracy: Semrush vs similarweb.
http://www.owox.com/blog/articles/semrush-vs-
similarweb. Acessed on 2024-04-18.
Ernst & Young LLP (2014). What-
sapp inc. financial statements.
http://www.sec.gov/Archives/edgar/data/1326801/
000132680114000047/exhibit991auditedwhatsappi
.htm. Acessed on 2024-04-10.
Evans, P. and Gawer, A. (2016). The rise of
the platform enterprise: A global survey.
http://www.thecge.net/app/uploads/2016/01/PDF-
WEB-Platform-Survey
01 12.pdf. Acessed on
2024-04-18.
Georgieva, G., Arnab, S., Romero, M., and de Freitas, S.
(2015). Transposing freemium business model from
casual games to serious games. Entertainment Com-
puting, 9-10:29–41.
Hardwick, J. (2018). Find out how much traf-
fic a website gets: 3 ways compared.
http://ahrefs.com/blog/website-traffic. Acessed
on 2024-04-18.
Hein, A., Schreieck, M., Riasanow, T., Setzke, D. S., Wi-
esche, M., B
¨
ohm, M., and Krcmar, H. (2020). Digital
platform ecosystems. Electron Markets, 30:87–98.
Hermes, S., Clemons, E., Schreieck, M., Pfab, S., Mitre,
M., B
¨
ohm, M., Wiesche, M., and Krcmar, H. (2020a).
Breeding grounds of digital platforms: Exploring
the sources of american platform domination, china’s
platform self-sufficiency, and europe’s platform gap.
In Proceedings of the 28th European Conference on
Information Systems (ECIS).
Hermes, S., Hein, A., B
¨
ohm, M., Pfab, S., Weking, J., and
Krcmar, H. (2020b). Digital platforms and market
dominance: Insights from a systematic literature re-
view and avenues for future research. In Proceedings
of the 24th Pacific Asia Conference on Information
Systems: Information Systems (IS) for the Future.
Huberman, B. A. (2001). The Laws of the Web: Patterns in
the Ecology of Information. The MIT Press.
Huizingh, E. K. (2000). The content and design of web
sites: an empirical study. Information & Management,
37(3):123–134.
Jansen, B. J., Jung, S.-g., and Salminen, J. (2022). Measur-
ing user interactions with websites: A comparison of
two industry standard analytics approaches using data
of 86 websites. PLOS ONE, 17(5):1–27.
Kutcher, E., Nottebohm, O., and Sprague,
K. (2014). Grow fast or die slow.
http://www.mckinsey.com/industries/technology-
media-and-telecommunications/our-insights/grow-
fast-or-die-slow. Acessed on 2024-04-10.
Lee, E., Lee, J., and Lee, J. (2006). Reconsideration of the
winner-take-all hypothesis: Complex networks and
local bias. Management Science, 52(12):1838–1848.
Liu, J., Li, X., and Guo, Y. (2017). Periodicity analysis
and a model structure for consumer behavior on hotel
online search interest in the us. International Journal
of Contemporary Hospitality Management, 29.
Liu, Z., Yan, Y., and Hauskrecht, M. (2018). A flexi-
ble forecasting framework for hierarchical time se-
ries with seasonal patterns: A case study of web traf-
fic. In The 41st International ACM SIGIR Conference
on Research & Development in Information Retrieval,
pages 889–892.
Petrosyan, A. (2024). Number of internet and so-
cial media users worldwide as of january 2024.
http://www.statista.com/statistics/617136/digital-
population-worldwide. Acessed on 2024-02-27.
Prantl, D. and Prantl, M. (2018). Website traffic measure-
ment and rankings: competitive intelligence tools ex-
amination. International Journal of Web Information
Systems, 14(4):423–437.
Srnicek, N. (2016). Platform Capitalism. John Wiley &
Sons, 1 edition.
Varoufakis, Y. (2024). Technofeudalism: What Killed Cap-
italism. Melville House, 1 edition.
Verisign (2023). Verisign domain name industry brief:
354 million domain name registrations in the first
quarter of 2023. http://blog.verisign.com/domain-
names/verisign-q1-2023-the-domain-name-industry-
brief. Acessed on 2024-02-21.
Webster, J. G. and Lin, S.-F. (2002). The internet audience:
Web use as mass behavior. Journal of Broadcasting &
Electronic Media, 46(1):1–12.
Zuboff, S. (2019). The age of surveillance capitalism: the
fight for a human future at the new frontier of power.
Public Affairs, 1 edition.
WEBIST 2024 - 20th International Conference on Web Information Systems and Technologies
190