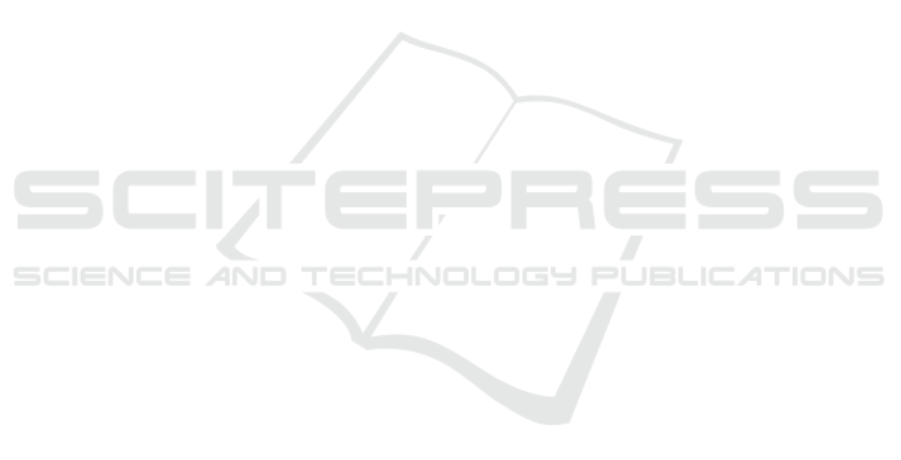
leveraging EMMO’s representational capabilities and
perspectives, this work has shown how four recently-
developed domain and application ontologies, i.e.
CHAMEO, BTO, HPO and MAEO, tackle different
application scenarios. EMMO offers an adaptable
framework for developing highly expressive domain
and application ontologies. By defining foundational
classes and properties and tracing them back to funda-
mental axioms of parthood, causation, persistence and
semiotics, EMMO ensures a comprehensive knowl-
edge representation, crucial for the advancement of
both semantic web technologies and neuro-symbolic
AI, and paves the way for improved interoperability
across diverse scientific and industrial domains.
ACKNOWLEDGEMENTS
This work was supported by the European Union’s
Horizon 2020 Research and Innovation Programme,
via NanoMECommons (G. A. n. 952869) and Open-
Model (G. A. n. 953167).
REFERENCES
Arp, R., Smith, B., and Spear, A. D. (2015). Building on-
tologies with basic formal ontology. MIT Press.
Atkin, A. (2023). Peirce’s theory of signs.
https://plato.stanford.edu/archives/spr2023/entries/
peirce-semiotics/. The Stanford Encyclopedia of
Philosophy (Spring 2023 Edition).
BIPM, IEC, IFCC, ILAC, ISO, IUPAC, IUPAP, and OIML
(2012). International vocabulary of metrology — Ba-
sic and general concepts and associated terms (VIM).
Technical report.
Bogen, J. and Woodward, J. (1988). Saving the phenomena.
The Philosophical Review, 97(3):303–352.
Bouraoui, Z., Cornu
´
ejols, A., Denoeux, T., Destercke, S.,
Dubois, D., Guillaume, R., Marques-Silva, J., Men-
gin, J., Prade, H., Schockaert, S., Serrurier, M., and
Vrain, C. (2019). From shallow to deep interac-
tions between knowledge representation, reasoning
and machine learning (kay r. amel group). CoRR,
abs/1912.06612.
Brickley, D. and Miller, L. (2014). Friend-Of-A-Friend.
http://xmlns.com/foaf/spec/.
De Baas, A., Nostro, P. D., Friis, J., Ghedini, E., Goldbeck,
G., Paponetti, I. M., Pozzi, A., Sarkar, A., Yang, L.,
Zaccarini, F. A., and Toti, D. (2023). Review and
Alignment of Domain-Level Ontologies for Materials
Science. IEEE Access, 11:120372–120401.
Del Nostro, P., Goldbeck, G., Pozzi, A., and Toti, D. (2023).
Modeling experts, knowledge providers and expertise
in Materials Modeling:MAEO as an application on-
tology of EMMO’s ecosystem. Applied Ontology,
18(2):99–118.
Del Nostro, P., Goldbeck, G., and Toti, D. (2022a).
CHAMEO: An ontology for the harmonisation of ma-
terials characterisation methodologies. Applied Ontol-
ogy, 17(3):401–421.
Del Nostro, P., Goldbeck, G., and Toti, D. (2022b). The
CHAMEO Ontology: Exploiting EMMO’s Multiper-
spective Versatility for Capturing Materials Character-
ization Procedures. In Proceedings of the 12th Inter-
national Workshop on Formal Ontologies meet Indus-
try (FOMI 2022), volume 3240 of CEUR Workshop
Proceedings. CEUR-WS.org.
Floridi, L. (2010). Information: A very short introduction.
OUP Oxford. p. 23.
Gangemi, A., Guarino, N., Masolo, C., Oltramari, A., and
Schneider, L. (2002). Sweetening ontologies with
DOLCE. In Knowledge Engineering and Knowl-
edge Management: Ontologies and the Semantic Web,
pages 166–181. Springer Berlin Heidelberg.
Garijo, D., Corcho, O., and Poveda-Villal
´
on, M. (2021).
FOOPS!: An Ontology Pitfall Scanner for the FAIR
Principles. 2980.
Goldbeck, G. and Toti, D. (2021). MAEO: An ontology
for modeling Agents, Experts and Expertise within
an open online Materials Modeling MarketPlace. In
CEUR Workshop Proceedings, volume 2969, pages
253–263.
Gruber, T. R. (1993). A translation approach to portable
ontology specifications. Knowledge Acquisition,
5(2):199–220.
Guarino, N., Oberle, D., and Staab, S. (2009). What is an
ontology? In Handbook on ontologies, pages 1–17.
Springer.
Lazzari, N., Giorgis, S. D., Gangemi, A., and Presutti, V.
(2024). Sandra - a neuro-symbolic reasoner based on
descriptions and situations. ArXiv, abs/2402.00591.
Levesque, H. J. and Brachman, R. J. (1987). Expressive-
ness and tractability in knowledge representation and
reasoning1. Computational Intelligence, 3(1):78–93.
Nostro, P. D., Goldbeck, G., Kienberger, F., Moertelmaier,
M., Pozzi, A., Al-Zubaidi-R-Smith, N., and Toti, D.
(2024). Battery Testing Ontology: an EMMO-based
Semantic Framework for Representing Knowledge in
Battery Testing and Battery Quality Control. Comput-
ers in Industry.
Partridge, C., Mitchell, A., and West, M. (2020). A sur-
vey of Top-Level Ontologies: To inform the ontologi-
cal choices for a Foundation Data Model.
Poveda-Villal
´
on, M., G
´
omez-P
´
erez, A., and Su
´
arez-
Figueroa, M. C. (2014). OOPS! (OntOlogy Pitfall
Scanner!): An On-line Tool for Ontology Evaluation.
International Journal on Semantic Web and Informa-
tion Systems (IJSWIS), 10(2):7–34.
Sider, T. (2001). Four-dimensionalism: An ontology of per-
sistence and time. Oxford University Press.
Trojahn, C., Vieira, R., Schmidt, D., Pease, A., and Guiz-
zardi, G. (2021). Foundational ontologies meet ontol-
ogy matching: A survey. Semantic Web, 13.
W3C (2012). Web Ontology Language. https://www.w3.
org/OWL/.
KEOD 2024 - 16th International Conference on Knowledge Engineering and Ontology Development
142