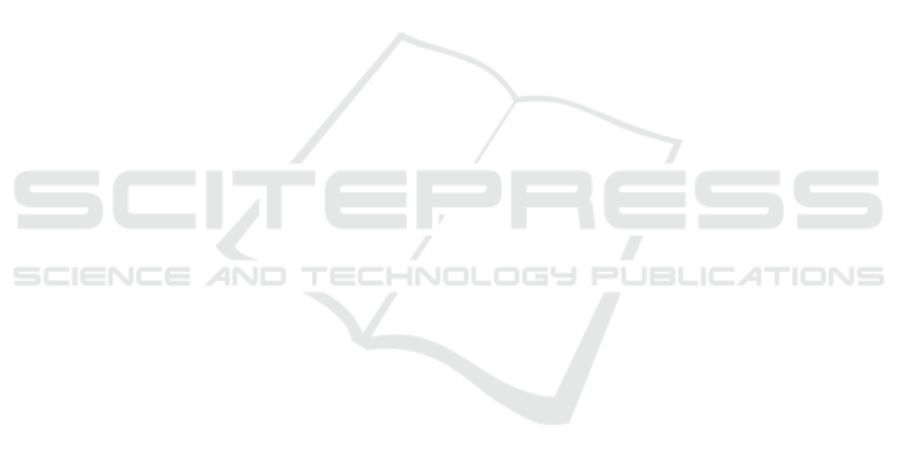
Concerning distance, while the relative distance
exerts a lesser impact on control performance com-
pared to speed, it still markedly influences the control
performance of the follower ship within the range of 1
to 1.2 times the ship’s length. Within this range, speed
fluctuations are particularly pronounced. Overall, the
interaction force between the two ships is governed by
both speed and distance, demonstrating greater sensi-
tivity to changes in speed. If these variations in force
are not incorporated into the controller design, they
could readily result in degraded control performance
or even failure.
The current study exclusively investigates a sce-
nario involving two ships in tandem and is designed
to visually demonstrate the impact of ship-to-ship in-
teraction forces on control. Controllers are specifi-
cally tailored for the follower ship, with the tracking
path constrained to straight lines. Future research will
broaden the scope of experimental scenarios to en-
compass various formation configurations, incorpo-
rating the interaction forces among ships within these
formations. Additionally, to verify the impact of ship-
to-ship interaction forces on control, this paper em-
ployed a relatively simple PID controller. This type
of controller has poor robustness and struggles to ac-
count for the inherent limitations of ASV, such as rud-
der angle rate and thruster output. Therefore, it is nec-
essary to design a more suitable controller specifically
tailored to these conditions to ensure the performance
of the control system.
ACKNOWLEDGMENTS
This research is supported by the "Researchlab Au-
tonomous Shipping (RAS)" of Delft University of
Technology.
REFERENCES
Bruggink, D., Cremer, Q., Groenewegen, R., and Klokgi-
eters, A. (2018). Differentiation of maneuvering co-
efficients for scaled model vessels. Technical report,
Technical report, Delft University of Technology.
Chen, C.-W., Chen, Y., and Cai, Q.-W. (2019).
Hydrodynamic-interaction analysis of an autonomous
underwater hovering vehicle and ship with wave ef-
fects. Symmetry, 11(10):1213.
Chen, L., Negenborn, R. R., Huang, Y., and Hopman, H.
(2021). Survey on cooperative control for waterborne
transport. IEEE Intelligent Transportation Systems
Magazine, 13(2):71–90.
De Decker, B. (2006). Ship-ship interaction during ligh-
tering operations. PhD thesis, MSc. thesis, NTNU,
Trondheim Norway.
Dong, Z., Liang, X., Guan, X., and Li, W. (2022). Forma-
tion optimization of various spacing configurations for
a fleet of unmanned surface vehicles based on a hydro-
dynamic energy-saving strategy. Ocean Engineering,
266:112824.
Du, Z., Negenborn, R. R., and Reppa, V. (2021a). Cooper-
ative multi-agent control for autonomous ship towing
under environmental disturbances. IEEE/CAA Jour-
nal of Automatica Sinica, 8(8):1365–1379.
Du, Z., Negenborn, R. R., and Reppa, V. (2021b). Cooper-
ative multi-agent control for autonomous ship towing
under environmental disturbances. IEEE/CAA Jour-
nal of Automatica Sinica, 8(8):1365–1379.
Fossen, T. I. (2011). Handbook of marine craft hydrody-
namics and motion control. John Wiley & Sons.
Gu, N., Wang, D., Peng, Z., and Liu, L. (2019). Observer-
based finite-time control for distributed path maneu-
vering of underactuated unmanned surface vehicles
with collision avoidance and connectivity preserva-
tion. IEEE Transactions on Systems, Man, and Cy-
bernetics: Systems, 51(8):5105–5115.
He, S., Wang, M., Dai, S.-L., and Luo, F. (2019).
Leader–follower formation control of usvs with pre-
scribed performance and collision avoidance. IEEE
Transactions on Industrial Informatics, 15(1):572–
581.
He, Y., Mou, J., Chen, L., Zeng, Q., Chen, P., and Zhang,
S. (2021). Survey on hydrodynamic effects on coop-
erative control of maritime autonomous surface ships.
Ocean Engineering, 235:109300.
He, Y., Mou, J., Chen, L., Zeng, Q., Huang, Y., Chen, P.,
and Zhang, S. (2022). Will sailing in formation re-
duce energy consumption? numerical prediction of
resistance for ships in different formation configura-
tions. Applied Energy, 312:118695.
Lataire, E., Vantorre, M., and Delefortrie, G. (2009). Cap-
tive model testing for ship to ship operations. In Inter-
national Conference on Marine Simulation and Ship
Maneuverability (MARSIM’09). Panama Canal Au-
thoritiy; International Marine Simulator Forum.
Lataire, E., Vantorre, M., Vandenbroucke, J., and Eloot, K.
(2011). Ship to ship interaction forces during lighter-
ing operations. In 2nd International conference on
Ship Manoeuvring in Shallow and Confined Water:
Ship to ship interaction, pages 211–222. Royal Insti-
tution of Naval Architects.
Liu, L., Wang, D., Peng, Z., and Li, T. (2017). Modular
adaptive control for los-based cooperative path ma-
neuvering of multiple underactuated autonomous sur-
face vehicles. IEEE Transactions on Systems, Man,
and Cybernetics: Systems, 47(7):1613–1624.
Liu, L., Wang, D., Peng, Z., Li, T., and Chen, C. P.
(2018). Cooperative path following ring-networked
under-actuated autonomous surface vehicles: Algo-
rithms and experimental results. IEEE transactions
on Cybernetics, 50(4):1519–1529.
Liu, L., Xu, Y., Huang, Z., Wang, H., and Wang, A. (2022).
Safe cooperative path following with relative-angle-
based collision avoidance for multiple underactuated
autonomous surface vehicles. Ocean Engineering,
258:111670.
Influence of Ship-to-Ship Interaction on Formation Control of Multi-Vessel Systems
35