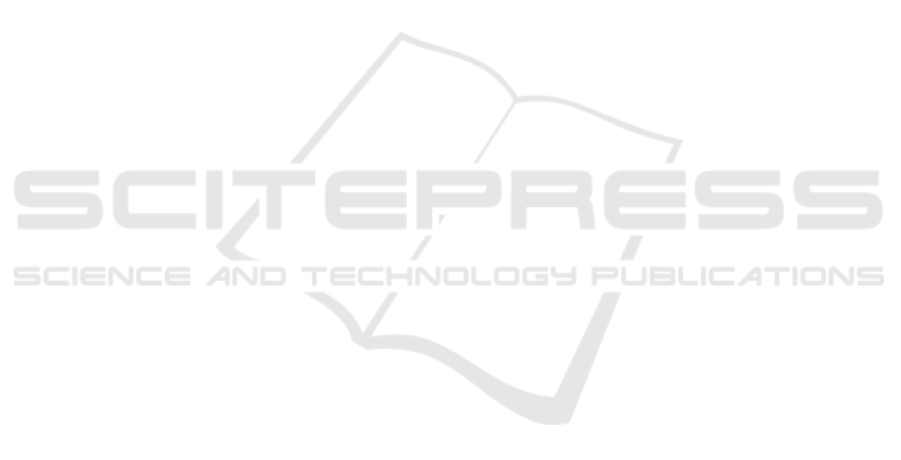
working memory, as attested by simplified computer-
based tasks, can significantly predict flight simulator
performance (Causse et al., 2011; Smit et al., 2021).
Looking more closely at the subscores of the three ex-
ecutive functions (i.e, inhibition, updating, shifting)
we found that only shifting could explain part of the
simulator performance. One explanation could stand
in the fact that working memory in flight management
has been mostly shown to be used for radio communi-
cation (Morrow et al., 2003; Smit et al., 2021), which
was absent in our simulator task.
4.1 Limits
It should be noted that this protocol is part of a larger
study on cognitive training of airplane pilots. The
present article focuses on the first flight simulator
session only, and subsequent results from this larger
study may be addressed in a future publication. The
sample size of pilots is not large enough to prevent
from false positive\negative correlation effects or to
test more advanced prediction models (e.g., multi-
ple regression models). Finally, in this first approach
study, neither the flight experience, nor the video
game experience have been taking into account, al-
though these could be covariates that explain a part
of variance in complex task or flight simulator perfor-
mance.
4.2 Conclusion
As a conclusion, our results suggest that the abil-
ity to handle the different subtasks in our most dif-
ficult flight simulator scenario seems to rely on being
able to handle interconnected tasks by switching ef-
ficiently between them and taking into account their
interdependence rather than just relying on working
memory, inhibition or unrelated multitasking compo-
nents.
Further research is planned to refine these predic-
tive models with a higher statistical power and more
exhaustive flight simulator performance assessment.
It will allow for more sophisticated models taking
into account pilot’s demographics and performance in
subtasks of the flying activity.
ACKNOWLEDGEMENTS
The authors wish to thank Stephane Perrey for his
help in obtaining the local committee approval. This
study is part of a research project funded by the
French procurement agency (DGA).
REFERENCES
Boot, W. R., Basak, C., Erickson, K. I., Neider, M., Simons,
D. J., Fabiani, M., Gratton, G., Voss, M. W., Prakash,
R., Lee, H., et al. (2010). Transfer of skill engendered
by complex task training under conditions of variable
priority. Acta psychologica, 135(3):349–357.
Causse, M., Dehais, F., and Pastor, J. (2011). Executive
functions and pilot characteristics predict flight simu-
lator performance in general aviation pilots. The Inter-
national Journal of Aviation Psychology, 21(3):217–
234.
Chenot, Q., Hamery, C., Lepron, E., Besson, P., De Boisse-
zon, X., Perrey, S., and Scannella, S. (2022). Perfor-
mance after training in a complex cognitive task is en-
hanced by high-definition transcranial random noise
stimulation. Scientific Reports, 12(1):4618.
Cristofori, I., Cohen-Zimerman, S., and Grafman, J. (2019).
Executive functions. Handbook of clinical neurology,
163:197–219.
Dehais, F., Dupr
`
es, A., Blum, S., Drougard, N., Scannella,
S., Roy, R. N., and Lotte, F. (2019a). Monitoring pi-
lot’s mental workload using erps and spectral power
with a six-dry-electrode eeg system in real flight con-
ditions. Sensors, 19(6):1324.
Dehais, F., Roy, R. N., and Scannella, S. (2019b). Inat-
tentional deafness to auditory alarms: Inter-individual
differences, electrophysiological signature and sin-
gle trial classification. Behavioural brain research,
360:51–59.
Durantin, G., Scannella, S., Gateau, T., Delorme, A., and
Dehais, F. (2016). Processing functional near in-
frared spectroscopy signal with a kalman filter to as-
sess working memory during simulated flight. Fron-
tiers in human neuroscience, 9:707.
Friedman, N. P. and Miyake, A. (2017). Unity and diver-
sity of executive functions: Individual differences as a
window on cognitive structure. Cortex, 86:186–204.
Friedman, N. P., Miyake, A., Young, S. E., DeFries, J. C.,
Corley, R. P., and Hewitt, J. K. (2008). Individual
differences in executive functions are almost entirely
genetic in origin. Journal of experimental psychology:
General, 137(2):201.
Gateau, T., Ayaz, H., and Dehais, F. (2018). In silico vs.
over the clouds: on-the-fly mental state estimation of
aircraft pilots, using a functional near infrared spec-
troscopy based passive-bci. Frontiers in human neu-
roscience, 12:187.
Gopher, D., Well, M., and Bareket, T. (1994). Transfer of
skill from a computer game trainer to flight. Human
factors, 36(3):387–405.
Jaeggi, S. M., Buschkuehl, M., Perrig, W. J., and Meier, B.
(2010). The concurrent validity of the n-back task as a
working memory measure. Memory, 18(4):394–412.
Kennedy, Q., Taylor, J. L., Reade, G., and Yesavage, J. A.
(2010). Age and expertise effects in aviation decision
making and flight control in a flight simulator. Avia-
tion, space, and environmental medicine, 81(5):489–
497.
From Laboratory to Cockpit: Evaluating the Predictive Value of Cognitive Tasks on Flight Simulator Performance
19