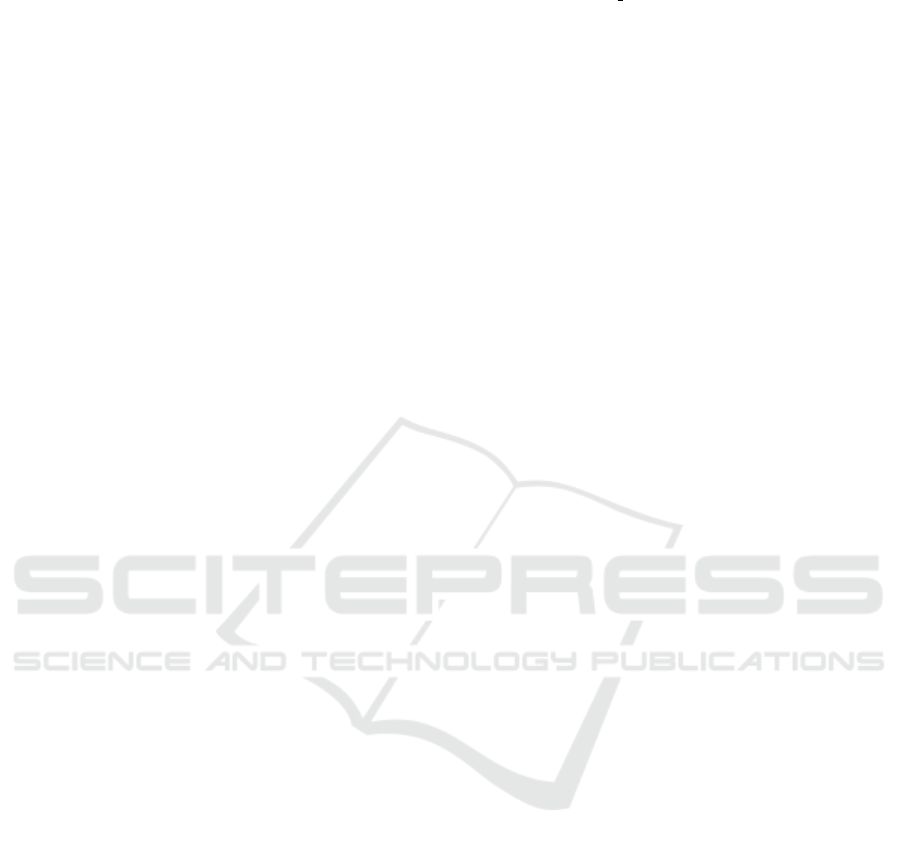
ity and friction) are included during simulations. Fur-
thermore, adding periodic activation functions, such
as tangent and cosine, could induce other patterns
of more efficient upward bending movements. An-
other avenue of future work may focus on improving
the performance of HyperNEAT with a broader ex-
ploration of hyper-parameters, such as the number of
neurons, hidden layers, and activation functions used
to design the substrate.
ACKNOWLEDGEMENTS
This project has received funding from the European
Union’s Horizon Europe research and innovation pro-
gramme under grant agreement No. 101070328.
UWE researchers were funded by the UK Research
and Innovation grant No. 10044516.
REFERENCES
Alcaraz-Herrera, H. and Cartlidge, J. (2022). Exploration of
ontological representations for evolutionary computa-
tion. In 2022 IEEE Congress on Evolutionary Com-
putation (CEC), pages 1–8.
Alcaraz-Herrera, H., Tsompanas, M.-A., Adamatzky, A.,
and Balaz, I. (2024a). Neuroevolution algorithms ap-
plied in the designing process of biohybrid actuators.
https://arxiv.org/abs/2408.07671.
Alcaraz-Herrera, H., Tsompanas, M.-A., Adamatzky,
A., and Balaz, I. (2024b). Using neuroevo-
lution for designing soft medical devices.
https://arxiv.org/abs/2408.09107.
Caceres, C., Rosario, J. M., and Amaya, D. (2017).
Approach of kinematic control for a nonholonomic
wheeled robot using artificial neural networks and ge-
netic algorithms. In 2017 International Conference
and Workshop on Bioinspired Intelligence (IWOBI),
pages 1–6.
Cheney, N., MacCurdy, R., Clune, J., and Lipson, H.
(2014). Unshackling evolution: evolving soft robots
with multiple materials and a powerful generative en-
coding. ACM SIGEVOlution, 7(1):11–23.
Glorot, X., Bordes, A., and Bengio, Y. (2011). Deep sparse
rectifier neural networks. In Proceedings of the Four-
teenth International Conference on Artificial Intelli-
gence and Statistics, Proceedings of Machine Learn-
ing Research, pages 315–323.
Hiller, J. and Lipson, H. (2014). Dynamic simulation of soft
multimaterial 3d-printed objects. Soft robotics, 1(1).
Hsiao, J.-H., Chang, J.-Y., and Cheng, C.-M. (2019). Soft
medical robotics: clinical and biomedical applica-
tions, challenges, and future directions. Advanced
Robotics, 33(21):1099–1111.
Kriegman, S., Blackiston, D., Levin, M., and Bongard,
J. (2020). A scalable pipeline for designing recon-
figurable organisms. https://github.com/skriegman/
reconfigurable
organisms. Accessed: May 29, 2024.
Risi, S. and Stanley, K. O. (2013). Confronting the chal-
lenge of learning a flexible neural controller for a
diversity of morphologies. In Proceedings of the
15th Annual Conference on Genetic and Evolutionary
Computation, GECCO ’13, page 255–262.
Rus, D. and Tolley, M. T. (2015). Design, fabrication and
control of soft robots. Nature, 521(7553):467–475.
Schulz, A., Sung, C., Spielberg, A., Zhao, W., Cheng, R.,
Grinspun, E., Rus, D., and Matusik, W. (2016). In-
teractive robogami: An end-to-end system for design
of robots with ground locomotion. The International
Journal of Robotics Research, 36(10):1131–1147.
Seriani, S., Marcini, L., Caruso, M., Gallina, P., and Med-
vet, E. (2021). Crowded environment navigation with
neat: Impact of perception resolution on controller op-
timization. Journal of Intelligent & Robotic Systems,
101(2).
Sokolov, M., Afanasyev, I., Klimchik, A., and Mavridis,
N. (2017). Hyperneat-based flipper control for a
crawler robot motion in 3d simulation environment.
In 2017 IEEE International Conference on Robotics
and Biomimetics (ROBIO), pages 2652–2656.
Stanley, K. O. (2007a). Compositional pattern pro-
ducing networks: A novel abstraction of develop-
ment. Genetic Programming and Evolvable Ma-
chines, 8(2):131–162.
Stanley, K. O. (2007b). Compositional pattern pro-
ducing networks: A novel abstraction of develop-
ment. Genetic Programming and Evolvable Ma-
chines, 8(2):131–162.
Stanley, K. O., D’Ambrosio, D. B., and Gauci, J. (2009). A
Hypercube-Based Encoding for Evolving Large-Scale
Neural Networks. Artificial Life, 15(2):185–212.
Stanley, K. O. and Miikkulainen, R. (2002). Evolving neu-
ral networks through augmenting topologies. Evolu-
tionary Computation, 10(2):99–127.
Tanaka, F. and Aranha, C. (2022). Co-evolving morphology
and control of soft robots using a single genome. In
2022 IEEE Symposium Series on Computational In-
telligence (SSCI), pages 1235–1242.
Tibermacine, A. and Djedi, N. (2014). Neat neural net-
works to control and simulate virtual creature’s loco-
motion. In 2014 International Conference on Multi-
media Computing and Systems (ICMCS), pages 9–14.
Tsompanas, M.-A. (2024). Incremental growth on composi-
tional pattern producing networks based optimization
of biohybrid actuators. In International Conference on
the Applications of Evolutionary Computation (Part of
EvoStar), pages 275–289. Springer.
Tsompanas, M.-A. and Balaz, I. (2024). Outline of an evo-
lutionary morphology generator towards the modular
design of a biohybrid catheter. Frontiers in Robotics
and AI, 11.
Wang, J. and Chortos, A. (2022). Control strategies for soft
robot systems. Advanced Intelligent Systems, 4(5):88–
101.
ECTA 2024 - 16th International Conference on Evolutionary Computation Theory and Applications
204