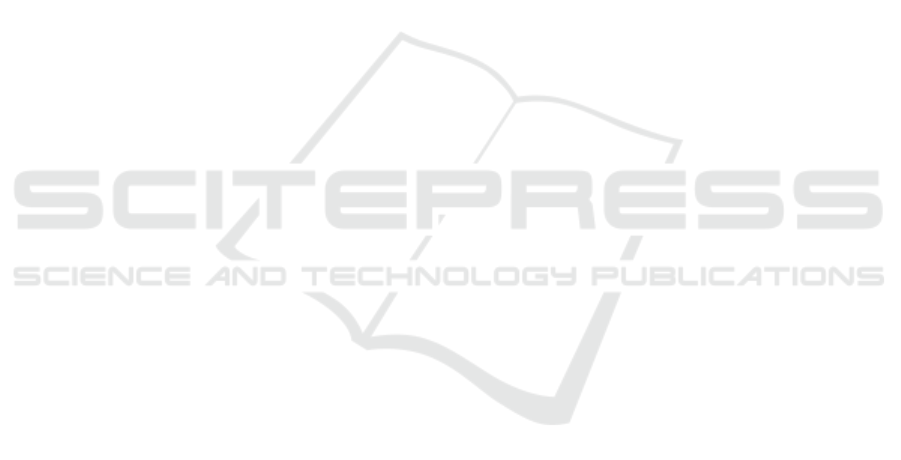
The real-time nature of the problem and the hard
task to precisely construct mathematical models for
such events on highway scenarios both contribute
to the choice of Machine Learning-based solutions.
This study demonstrates the efficacy and scalability
of a Cooperative Multi-Agent Reinforcement Learn-
ing (cMARL) approach.
As detailed in its context, three important meth-
ods have been utilized as benchmarks in our exten-
sive comparison scheme, being the free-flow condi-
tion with no control enabled, the MCS currently used
in real world application in Sweden, and the MTFC,
which can achieve the best performance in certain
flow metrics according to a survey carried out among
VSLC techniques.
The results, obtained from extensive simulations
in both normal and high traffic density conditions,
highlight the significant advantages of cMARL over
traditional traffic management methods. Specifically,
cMARL consistently outperforms baseline methods
in reducing average travel times, waiting times, and
queue lengths, thus enhancing overall traffic flow effi-
ciency. Furthermore, the approach proves to be highly
effective in lowering fuel consumption and emissions
of CO
2
and NO
x
.
Overall, the dual benefits of improved traffic flow
and reduced environmental impact make cMARL a
promising solution for modern traffic management
challenges. The results of this study pave the way
for future research: further development of the RL
abstraction terms would certainly yield to even better
results, such as a sliding kernel-type state representa-
tion for the ease of interpretability.
ACKNOWLEDGEMENTS
This work was supported by the European Union
within the framework of the National Labora-
tory for Autonomous Systems (RRF-2.3.1-21-2022-
00002). T.B. was supported by BO/00233/21/6: the
J
´
anos Bolyai Research Scholarship of the Hungarian
Academy of Sciences.
REFERENCES
Alvarez Lopez, P., Behrisch, M., Bieker-Walz, L., Erdmann,
J., Fl
¨
otter
¨
od, Y.-P., Hilbrich, R., L
¨
ucken, L., Rum-
mel, J., Wagner, P., and Wießner, E. (2018). Micro-
scopic traffic simulation using sumo. In 2019 IEEE In-
telligent Transportation Systems Conference (ITSC),
pages 2575–2582. IEEE.
An, S.-h., Lee, B.-H., and Shin, D.-R. (2011). A sur-
vey of intelligent transportation systems. In 2011
third international conference on computational intel-
ligence, communication systems and networks, pages
332–337. IEEE.
F
´
enyes, D., N
´
emeth, B., and G
´
asp
´
ar, P. (2021). A novel
data-driven modeling and control design method for
autonomous vehicles. Energies, 14(2):517.
Grumert, E. F., Tapani, A., and Ma, X. (2018). Characteris-
tics of variable speed limit systems. European trans-
port research review, 10:1–12.
Huang, D., Shere, S., and Ahn, S. (2010). Dynamic high-
way congestion detection and prediction based on
shock waves. In Proceedings of the seventh ACM in-
ternational workshop on VehiculAr InterNETworking,
pages 11–20.
Kavas-Torris, O., Gelbal, S. Y., Cantas, M. R., Aksun Gu-
venc, B., and Guvenc, L. (2022). V2x commu-
nication between connected and automated vehicles
(cavs) and unmanned aerial vehicles (uavs). Sensors,
22(22):8941.
Khondaker, B. and Kattan, L. (2015). Variable speed limit:
an overview. Transportation Letters, 7(5):264–278.
Kim, Y., Kang, K., Park, N., Park, J., and Oh, C. (2024).
Reinforcement learning approach to develop variable
speed limit strategy using vehicle data and simula-
tions. Journal of Intelligent Transportation Systems,
pages 1–18.
K
˝
ov
´
ari, B., Sz
˝
oke, L., B
´
ecsi, T., Aradi, S., and G
´
asp
´
ar, P.
(2021). Traffic signal control via reinforcement learn-
ing for reducing global vehicle emission. Sustainabil-
ity, 13(20):11254.
Ku
ˇ
si
´
c, K., Ivanjko, E., Greguri
´
c, M., and Mileti
´
c, M.
(2020). An overview of reinforcement learning meth-
ods for variable speed limit control. Applied Sciences,
10(14):4917.
K
˝
ov
´
ari, B., Kn
´
ab, I., and B
´
ecsi, T. (EasyChair, 2024). Vari-
able speed limit control for highway scenarios a multi-
agent reinforcement learning based appraoch. Easy-
Chair Preprint no. 13400.
Lahmiss, H. and Khatory, A. (2020). Variable speed limit
(vsl) system applications in motorways. In 2020 IEEE
13th International Colloquium of Logistics and Sup-
ply Chain Management (LOGISTIQUA), pages 1–5.
IEEE.
M
¨
uller, E. R., Carlson, R. C., Kraus, W., and Papageorgiou,
M. (2013). Microscopic simulation analysis of main-
stream traffic flow control with variable speed lim-
its. In 16th International IEEE Conference on Intelli-
gent Transportation Systems (ITSC 2013), pages 998–
1003. IEEE.
Richey Jr, R. G., Chowdhury, S., Davis-Sramek, B., Gian-
nakis, M., and Dwivedi, Y. K. (2023). Artificial intel-
ligence in logistics and supply chain management: A
primer and roadmap for research.
Van Toorenburg, J. and De Kok, M. (1999). Automatic in-
cident detection in the motorway control system mtm.
Bureau Transpute, Gouda.
ICINCO 2024 - 21st International Conference on Informatics in Control, Automation and Robotics
124