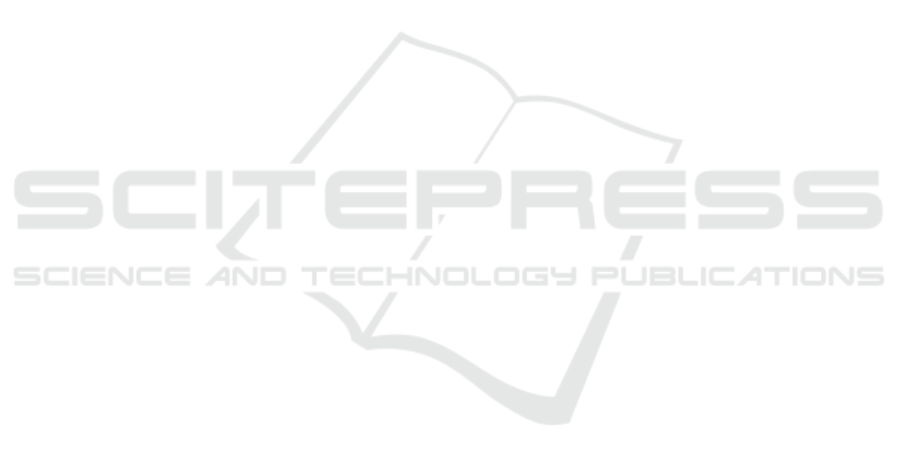
Cottier, B. (2023). Trends in the dollar training cost of ma-
chine learning systems. Accessed: 2023-12-18.
Dimitrakopoulos, G. and Demestichas, P. (2010). Intelli-
gent transportation systems. IEEE Vehicular Technol-
ogy Magazine, 5(1):77–84.
Fenger, J. (1999). Urban air quality. Atmospheric environ-
ment, 33(29):4877–4900.
Kesting, A. and Treiber, M. (2008). How reaction time, up-
date time, and adaptation time influence the stability
of traffic flow. Computer-Aided Civil and Infrastruc-
ture Engineering, 23(2):125–137.
Khondaker, B. and Kattan, L. (2015). Variable speed limit:
an overview. Transportation Letters, 7(5):264–278.
Kolat, M., K
˝
ov
´
ari, B., B
´
ecsi, T., and Aradi, S. (2023).
Multi-agent reinforcement learning for traffic signal
control: A cooperative approach. Sustainability,
15(4):3479.
K
˝
ov
´
ari, B., Kn
´
ab, I., and B
´
ecsi, T. (2024). Variable speed
limit control for highway scenarios a multi-agent rein-
forcement learning based appraoch. Technical report,
EasyChair.
K
¨
ov
´
ari, B., Pelenczei, B., and B
´
ecsi, T. (2022). Monte carlo
tree search to compare reward functions for reinforce-
ment learning. In 2022 IEEE 16th International Sym-
posium on Applied Computational Intelligence and
Informatics (SACI), pages 000123–000128. IEEE.
Krajzewicz, D., Hausberger, S., Wagner, P., Behrisch, M.,
and Krumnow, M. (2014). Second generation of pol-
lutant emission models for sumo. In SUMO2014 -
Second SUMO User Conference, Reports of the DLR-
Institute of Transportation Systems.
Kustija, J. et al. (2023). Scats (sydney coordinated adaptive
traffic system) as a solution to overcome traffic con-
gestion in big cities. International Journal of Research
and Applied Technology (INJURATECH), 3(1):1–14.
Mirchandani, P. and Wang, F.-Y. (2005). Rhodes to intelli-
gent transportation systems. IEEE Intelligent Systems,
20(1):10–15.
Mnih, V., Kavukcuoglu, K., Silver, D., Rusu, A. A., Ve-
ness, J., Bellemare, M. G., Graves, A., Riedmiller, M.,
Fidjeland, A. K., Ostrovski, G., et al. (2015). Human-
level control through deep reinforcement learning. na-
ture, 518(7540):529–533.
Nadarajan, R. and Sulaiman, N. (2021). Comparative anal-
ysis in execution of machine learning in breast cancer
identification: A review. Journal of Physics: Confer-
ence Series, 1874:012032.
Now
´
e, A., Vrancx, P., and De Hauwere, Y.-M. (2012).
Game theory and multi-agent reinforcement learning.
Reinforcement Learning: State-of-the-Art, pages 441–
470.
Qureshi, K. N. and Abdullah, A. H. (2013). A survey on in-
telligent transportation systems. Middle-East Journal
of Scientific Research, 15(5):629–642.
Reddy, S., Allan, S., Coghlan, S., and Cooper, P. (2020). A
governance model for the application of ai in health
care. Journal of the American Medical Informatics
Association, 27(3):491–497.
Ritchie, H. (2020). Sector by sector: where do global green-
house gas emissions come from? Our World in Data.
https://ourworldindata.org/ghg-emissions-by-sector.
Ritchie, H. (2024). Tracking global data on
electric vehicles. Our World in Data.
https://ourworldindata.org/electric-car-sales.
Ross, P. and Maynard, K. (2021). Towards a 4th industrial
revolution.
Rotake, D. and Karmore, S. (2012). Intelligent traffic signal
control system using embedded system. Innovative
systems design and engineering, 3(5):11–20.
Singh, B. and Gupta, A. (2015). Recent trends in intelligent
transportation systems: a review. Journal of transport
literature, 9:30–34.
Sutton, R. S. and Barto, A. G. (2018). Reinforcement learn-
ing: An introduction. MIT press.
Sutton, R. S., Barto, A. G., et al. (1999). Reinforce-
ment learning. Journal of Cognitive Neuroscience,
11(1):126–134.
Szepesv
´
ari, C. (2022). Algorithms for reinforcement learn-
ing. Springer nature.
Wang, F.-Y., Zhang, J. J., Zheng, X., Wang, X., Yuan, Y.,
Dai, X., Zhang, J., and Yang, L. (2016). Where does
alphago go: From church-turing thesis to alphago the-
sis and beyond. IEEE/CAA Journal of Automatica
Sinica, 3(2):113–120.
Wei, H., Zheng, G., Gayah, V., and Li, Z. (2021). Recent
advances in reinforcement learning for traffic signal
control: A survey of models and evaluation. ACM
SIGKDD Explorations Newsletter, 22(2):12–18.
Wei, H., Zheng, G., Yao, H., and Li, Z. (2018). Intellilight:
A reinforcement learning approach for intelligent traf-
fic light control. In Proceedings of the 24th ACM
SIGKDD international conference on knowledge dis-
covery & data mining, pages 2496–2505.
Wiering, M. A. et al. (2000). Multi-agent reinforcement
learning for traffic light control. In Machine Learning:
Proceedings of the Seventeenth International Confer-
ence (ICML’2000), pages 1151–1158.
Ye, B.-L., Wu, W., Ruan, K., Li, L., Chen, T., Gao, H., and
Chen, Y. (2019). A survey of model predictive control
methods for traffic signal control. IEEE/CAA Journal
of Automatica Sinica, 6(3):623–640.
Zhang, J., Wang, F.-Y., Wang, K., Lin, W.-H., Xu, X., and
Chen, C. (2011). Data-driven intelligent transporta-
tion systems: A survey. IEEE Transactions on Intelli-
gent Transportation Systems, 12(4):1624–1639.
Expanded Applicability: Multi-Agent Reinforcement Learning-Based Traffic Signal Control in a Variable-Sized Environment
25