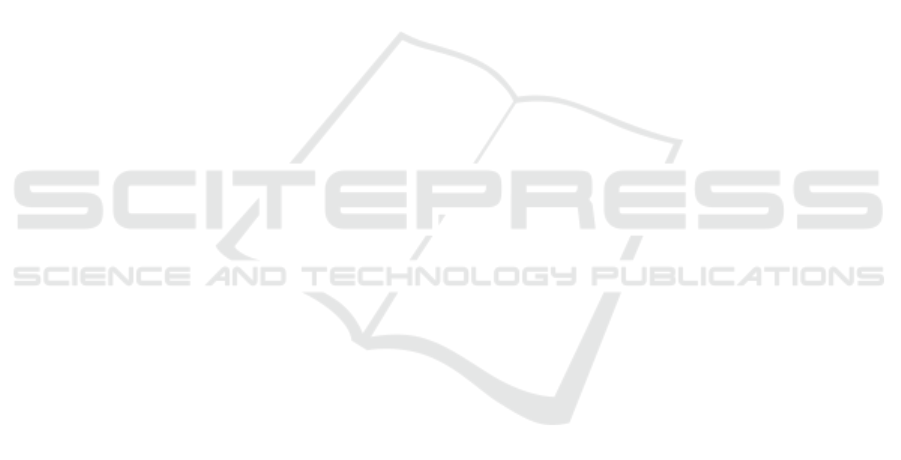
Cho, N. H., Kirigia, J., Mbanya, J. C., Ogurstova, K., Guar-
iguata, L., and Rathmann, W. (2015). IDF Diabetes
Atlas-8th. International Diabetes Federation.
da C. Oliveira, Leandro, A., d. Carvalho, A. B., and Dantas,
D. O. (2021). Faster r-cnn approach for diabetic foot
ulcer detection. In VISIGRAPP (4: VISAPP) , pages
677–684.
Das, S. K., Roy, P., and Mishra, A. K. (2022). Dfu-spnet:
A stacked parallel convolution layers based cnn to im-
prove diabetic foot ulcer classification. ICT Express,
8(2):271–275.
Filipe, V., Teixeira, P., and Teixeira, A. (2022). Auto-
matic classification of foot thermograms using ma-
chine learning techniques. Algorithms, 15(7):236.
Ghanassia, E., Villon, L., d. Dieudonn
´
e, J.-F. T., Boeg-
ner, C., Avignon, A., and Sultan, A. (2008). Long-
term outcome and disability of diabetic patients hos-
pitalized for diabetic foot ulcers: a 6.5-year follow-up
study. Diabetes Care, 31(7):1288–1292.
Haque, F., Reaz, M. B. I., Chowdhury, M. E. H., Ezed-
din, M., Kiranyaz, S., Alhatou, M., Ali, S. H. M.,
Bakar, A. A. A., and Srivastava, G. (2022). Machine
learning-based diabetic neuropathy and previous foot
ulceration patients detection using electromyography
and ground reaction forces during gait. Sensors,
22(9):3507.
Hossain, M. S., Nakamura, T., Kimura, F., Yagi, Y., and
Yamaguchi, M. (2018). Practical image quality eval-
uation for whole slide imaging scanner. In Biomedi-
cal Imaging and Sensing Conference, volume 10711,
pages 203–206. SPIE.
Jeffcoate, W. J. and Harding, K. G. (2003). Diabetic foot
ulcers. The Lancet, 361(9368):1545–1551.
Kendrick, C., Cassidy, B., Pappachan, J. M., O’Shea, C.,
Fernandez, C. J., Chacko, E., Jacob, K., Reeves,
N. D., and Yap, M. H. (2022). Translating clin-
ical delineation of diabetic foot ulcers into ma-
chine interpretable segmentation. arXiv preprint
arXiv:2204.11618.
Khandakar, A., Chowdhury, M. E. H., Reaz, M. B. I., Ali, S.
H. M., Abbas, T. O., Alam, T., Ayari, M. A., Mahbub,
Z. B., Habib, R., and Rahman, T. (2022a). Thermal
change index-based diabetic foot thermogram image
classification using machine learning techniques. Sen-
sors, 22(5):1793.
Khandakar, A., Chowdhury, M. E. H., Reaz, M. B. I., Ali,
S. H. M., Hasan, M. A., Kiranyaz, S., Rahman, T.,
Alfkey, R., Bakar, A. A. A., and Malik, R. A. (2021).
A machine learning model for early detection of di-
abetic foot using thermogram images. Computers in
Biology and Medicine, 137:104838.
Khandakar, A., Chowdhury, M. E. H., Reaz, M. B. I., Ali, S.
H. M., Kiranyaz, S., Rahman, T., Chowdhury, M. H.,
Ayari, M. A., Alfkey, R., and Bakar, A. A. A. (2022b).
A novel machine learning approach for severity clas-
sification of diabetic foot complications using thermo-
gram images. Sensors, 22(11):4249.
Laith (2021). Diabetic foot ulcer (dfu).
Munadi, K., Saddami, K., Oktiana, M., Roslidar, R.,
Muchtar, K., Melinda, M., Muharar, R., Syukri, M.,
Abidin, T. F., and Arnia, F. (2022). A deep learning
method for early detection of diabetic foot using de-
cision fusion and thermal images. Applied Sciences,
12(15):7524.
Ponirakis, G., Elhadd, T., Chinnaiyan, S., Dabbous,
Z., Siddiqui, M., Al-muhannadi, H., Petropou-
los, I. N., Khan, A., Ashawesh, K. A. E., and
Dukhan, K. M. O. (2020). Prevalence and man-
agement of diabetic neuropathy in secondary care in
qatar. Diabetes/Metabolism Research and Reviews,
36(4):e3286.
Saeedi, P., Petersohn, I., Salpea, P., Malanda, B., Karu-
ranga, S., Unwin, N., Colagiuri, S., Guariguata, L.,
Motala, A. A., and Ogurtsova, K. (2019). Global and
regional diabetes prevalence estimates for 2019 and
projections for 2030 and 2045: Results from the inter-
national diabetes federation diabetes atlas. Diabetes
Research and Clinical Practice, 157:107843.
Scebba, G., Zhang, J., Catanzaro, S., Mihai, C., Distler,
O., Berli, M., and Karlen, W. (2022). Detect-and-
segment: a deep learning approach to automate wound
image segmentation. Informatics in Medicine Un-
locked, 29:100884.
Singh, G. and Chawla, S. (2006). Amputation in dia-
betic patients. Medical Journal Armed Forces India,
62(1):36–39.
Thotad, P. N., Bharamagoudar, G. R., and Anami, B. S.
(2023). Diabetic foot ulcer detection using deep learn-
ing approaches. Sensors International, 4:100210.
Xie, P., Li, Y., Deng, B., Du, C., Rui, S., Deng, W., Wang,
M., Boey, J., Armstrong, D. G., and Ma, Y. (2022). An
explainable machine learning model for predicting in-
hospital amputation rate of patients with diabetic foot
ulcer. International Wound Journal, 19(4):910–918.
Yap, M. H., Hachiuma, R., Alavi, A., Br
¨
ungel, R., Cassidy,
B., Goyal, M., Zhu, H., R
¨
uckert, J., Olshansky, M.,
and Huang, X. (2021). Deep learning in diabetic foot
ulcers detection: a comprehensive evaluation. Com-
puters in Biology and Medicine, 135:104596.
ICINCO 2024 - 21st International Conference on Informatics in Control, Automation and Robotics
292