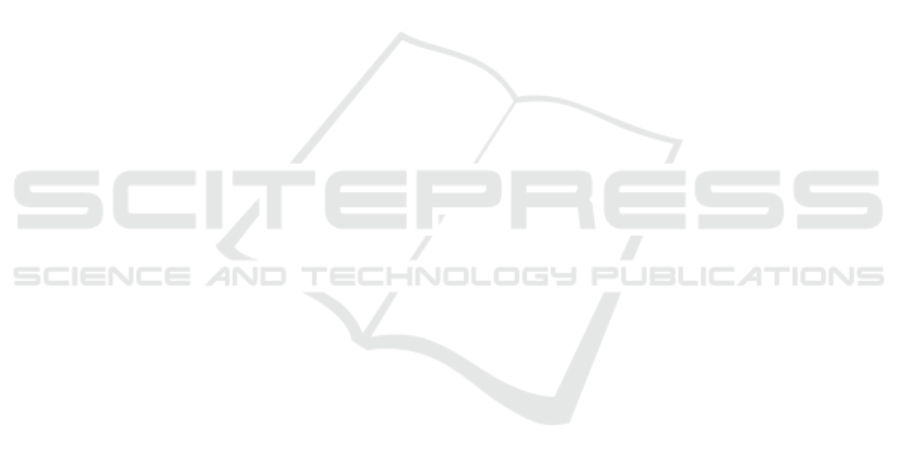
ferent methods than PHI. Furthermore, we can di-
rectly understand the differences between the com-
pared methods through PHI
+
values. The experimen-
tal results show that PHI
+
can more clearly reflect the
changes in the solutions within the ROI.
Although PHI
+
has more advantages than PHI,
there is still room for further improvement. An as-
pect to explore further is the situation where multiple
methods do not obtain a solution in the modified ROI.
In this case, it is not easy to distinguish these methods
because PHI
+
values for them are all zero. Therefore,
our next step is to evaluate solutions outside the mod-
ified ROI so that PHI
+
can work properly even when
there is no solution in the modified ROI.
Additionally, to provide information in the PHI
+
values about the attainability of the desirable ranges,
we had to accept some discontinuity in the PHI
+
val-
ues near 1. From our observations, resolving this dis-
continuity without harming other valuable properties
is challenging. Therefore, this is also our future work.
ACKNOWLEDGEMENTS
This research has received part of the funding from
the European Union – NextGenerationEU instrument
and was therefore partly funded by the Research
Council of Finland, grant number 352784, partly by
grant number 355346 of the same Council and is re-
lated to the thematic research area Decision Analyt-
ics utilizing Causal Models and Multiobjective Opti-
mization (jyu.fi/demo) of the University of Jyvaskyla.
REFERENCES
Afsar, B., Miettinen, K., and Ruiz, F. (2021). Assessing the
performance of interactive multiobjective optimiza-
tion methods: A survey. ACM Computing Surveys,
54(4):1–27.
Aghaei Pour, P., Bandaru, S., Afsar, B., Emmerich, M.,
and Miettinen, K. (2024). A performance indicator
for interactive evolutionary multiobjective optimiza-
tion methods. IEEE Transactions on Evolutionary
Computation, 28(3):778–787.
Aghaei Pour, P., Bandaru, S., Afsar, B., and Miettinen,
K. (2022). Desirable properties of performance indi-
cators for assessing interactive evolutionary multiob-
jective optimization methods. In Proceedings of the
Genetic and Evolutionary Computation Conference,
Companion, pages 1803–1811. ACM.
Branke, J., Deb, K., Miettinen, K., and Slowinski, R., edi-
tors (2008). Multiobjective Optimization: Interactive
and Evolutionary Approaches. Springer.
Cheng, R., Jin, Y., Olhofer, M., and Sendhoff, B. (2016).
A reference vector guided evolutionary algorithm for
many-objective optimization. IEEE Transactions on
Evolutionary Computation, 20(5):773–791.
Hakanen, J., Chugh, T., Sindhya, K., Jin, Y., and Miet-
tinen, K. (2016). Connections of reference vectors
and different types of preference information in in-
teractive multiobjective evolutionary algorithms. In
2016 IEEE Symposium Series on Computational In-
telligence (SSCI), pages 1–8. IEEE.
L
´
arraga, G. and Miettinen, K. (2022). Interactive MOEA/D
with multiple types of preference information. In
Proceedings of the Genetic and Evolutionary Com-
putation Conference, Companion, pages 1826–1834.
ACM.
Luque, M., Ruiz, F., and Miettinen, K. (2011). Global for-
mulation for interactive multiobjective optimization.
OR Spectrum, 33:27–48.
Manuel, M., Hien, B., Conrady, S., Kreddig, A., Doan, N.
A. V., and Stechele, W. (2022). Region of interest
based non-dominated sorting genetic algorithm-II: an
invite and conquer approach. In Proceedings of the
Genetic and Evolutionary Computation Conference,
pages 556–564. ACM.
Miettinen, K. (1999). Nonlinear Multiobjective Optimiza-
tion. Kluwer.
Miettinen, K., Hakanen, J., and Podkopaev, D. (2016). In-
teractive nonlinear multiobjective optimization meth-
ods. In Multiple Criteria Decision Analysis: State of
the Art Surveys, pages 927–976. Springer.
Miettinen, K., Ruiz, F., and Wierzbicki, A. P. (2008). Intro-
duction to multiobjective optimization: Interactive ap-
proaches. In Multiobjective Optimization: Interactive
and Evolutionary Approaches, pages 27–57. Springer.
Misitano, G., Saini, B. S., Afsar, B., Shavazipour, B., and
Miettinen, K. (2021). DESDEO: The modular and
open source framework for interactive multiobjective
optimization. IEEE Access, 9:148277–148295.
Sawaragi, Y., Nakayama, H., and Tanino, T. (1985). Theory
of Multiobjective Optimization. Elsevier.
Tanabe, R. and Ishibuchi, H. (2020). An easy-to-use real-
world multi-objective optimization problem suite. Ap-
plied Soft Computing, 89:106078.
Tanabe, R. and Li, K. (to appear). Quality indicators
for preference-based evolutionary multi-objective op-
timization using a reference point: A review and anal-
ysis. IEEE Transactions on Evolutionary Computa-
tion. doi: 10.1109/TEVC.2023.3319009.
Wierzbicki, A. P. (1980). The use of reference objectives
in multiobjective optimization. In Multiple Criteria
Decision Making Theory and Application, pages 468–
486. Springer.
Zitzler, E. and Thiele, L. (1998). Multiobjective optimiza-
tion using evolutionary algorithms — A comparative
case study. In Parallel Problem Solving from Nature
– PPSN V 5th International Conference, Proceedings,
pages 292–301. Springer.
A Modified Preference-Based Hypervolume Indicator for Interactive Evolutionary Multiobjective Optimization Methods
221