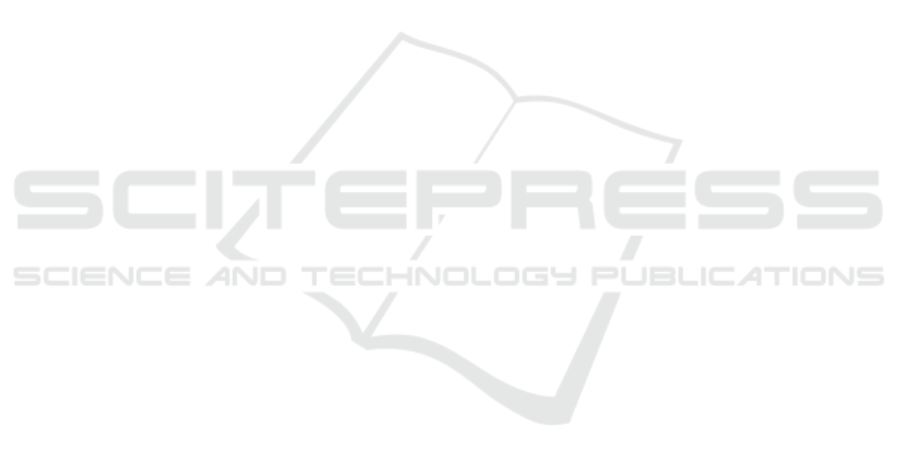
improved the ability to generate concise and mean-
ingful video summaries, enhancing their performance
across various tasks.
Despite the progress made, several challenges re-
main. Current techniques often struggle with limita-
tions in real-world scenarios, particularly in achiev-
ing real-time summarization and context-awareness.
Additionally, there is a lack of robust multi-modal
datasets that incorporate diverse features such as text,
audio, and visual data, which are critical for further
improving the adaptability of summarization models
across different applications, from personalized video
recommendations to security monitoring.
Looking forward, future research must focus on
addressing these limitations by developing techniques
that are more efficient and accurate, particularly in
real-time and context-sensitive environments. Fur-
thermore, the creation of more comprehensive, multi-
modal datasets will be essential to unlocking the full
potential of video summarization technologies.
In summary, while significant progress has been
made, this review has identified key gaps in current
methodologies. Overcoming these challenges will
be vital to fully harness the potential of video sum-
marization as video content continues to proliferate
across various domains.
REFERENCES
Apostolidis, E., Adamantidou, E., Metsai, A. I., Mezaris,
V., and Patras, I. (2020). Ac-sum-gan: Connecting
actor-critic and generative adversarial networks for
unsupervised video summarization. IEEE Transac-
tions on Circuits and Systems for Video Technology,
31(8):3278–3292.
Apostolidis, E., Adamantidou, E., Metsai, A. I., Mezaris,
V., and Patras, I. (2021). Video summarization using
deep neural networks: A survey. Proceedings of the
IEEE, 109(11):1838–1863.
Banjar, A., Dawood, H., Javed, A., and Zeb, B. (2024).
Sports video summarization using acoustic symmet-
ric ternary codes and svm. Applied Acoustics,
216:109795.
Basavarajaiah, M. and Sharma, P. (2021). Gvsum: generic
video summarization using deep visual features. Mul-
timedia Tools and Applications, 80(9):14459–14476.
Benedetto, I., La Quatra, M., Cagliero, L., Canale, L., and
Farinetti, L. (2023). Abstractive video lecture summa-
rization: applications and future prospects. Education
and Information Technologies, 29(3):2951–2971.
Elfeki, M., Wang, L., and Borji, A. (2022). Multi-stream
dynamic video summarization. In Proceedings of
the IEEE/CVF Winter Conference on Applications of
Computer Vision, pages 339–349.
Ester, M., Kriegel, H.-P., Sander, J., and Xu, X. (1996).
A density-based algorithm for discovering clusters in
large spatial databases with noise. In Knowledge Dis-
covery and Data Mining.
Ghauri, J. A., Hakimov, S., and Ewerth, R. (2020).
Classification of important segments in educational
videos using multimodal features. arXiv preprint
arXiv:2010.13626.
Gong, B., Chao, W.-L., Grauman, K., and Sha, F. (2014).
Diverse sequential subset selection for supervised
video summarization. Advances in neural information
processing systems, 27.
Gygli, M., Grabner, H., Riemenschneider, H., and
Van Gool, L. (2014). Creating summaries from user
videos. In Computer Vision–ECCV 2014: 13th Euro-
pean Conference, Zurich, Switzerland, September 6-
12, 2014, Proceedings, Part VII 13, pages 505–520.
Springer.
Hassan, S., Saleh, M., Kubba, M., et al. (2018). Wikihow:
A large scale text summarization dataset. In Proceed-
ings of the 2018 Conference on Empirical Methods in
Natural Language Processing, pages 3243–3252.
He, B., Wang, J., Qiu, J., Bui, T., Shrivastava, A., and Wang,
Z. (2023). Align and attend: Multimodal summariza-
tion with dual contrastive losses. In Proceedings of
the IEEE/CVF Conference on Computer Vision and
Pattern Recognition, pages 14867–14878.
He, X., Hua, Y., Song, T., Zhang, Z., Xue, Z., Ma, R.,
Robertson, N., and Guan, H. (2019). Unsupervised
video summarization with attentive conditional gener-
ative adversarial networks. In Proceedings of the 27th
ACM International Conference on multimedia, pages
2296–2304.
Kumar, K., Shrimankar, D. D., and Singh, N. (2016). Equal
partition based clustering approach for event summa-
rization in videos. In 2016 12th International Confer-
ence on Signal-Image Technology & Internet-Based
Systems (SITIS), pages 119–126.
Lee, Y. J., Ghosh, J., and Grauman, K. (2012). Discover-
ing important people and objects for egocentric video
summarization. In 2012 IEEE Conference on Com-
puter Vision and Pattern Recognition, pages 1346–
1353.
Li, P., Ye, Q., Zhang, L., Yuan, L., Xu, X., and Shao, L.
(2021). Exploring global diverse attention via pair-
wise temporal relation for video summarization. Pat-
tern Recognition, 111:107677.
Lin, J., Zhong, S.-h., and Fares, A. (2022). Deep hier-
archical lstm networks with attention for video sum-
marization. Computers & Electrical Engineering,
97:107618.
Liu, T., Meng, Q., Huang, J.-J., Vlontzos, A., Rueckert, D.,
and Kainz, B. (2022). Video summarization through
reinforcement learning with a 3d spatio-temporal u-
net. IEEE Transactions on Image Processing, 31.
Mahmoud, K. M., Ismail, M. A., and Ghanem, N. M.
(2013). VSCAN: An Enhanced Video Summariza-
tion Using Density-Based Spatial Clustering, page
733–742. Springer Berlin Heidelberg.
Miech, A., Laptev, I., and Sivic, J. (2019). Howto100m:
Learning a text-video embedding by watching hun-
dred million video clips. In Proceedings of the
Video Summarization Techniques: A Comprehensive Review
147