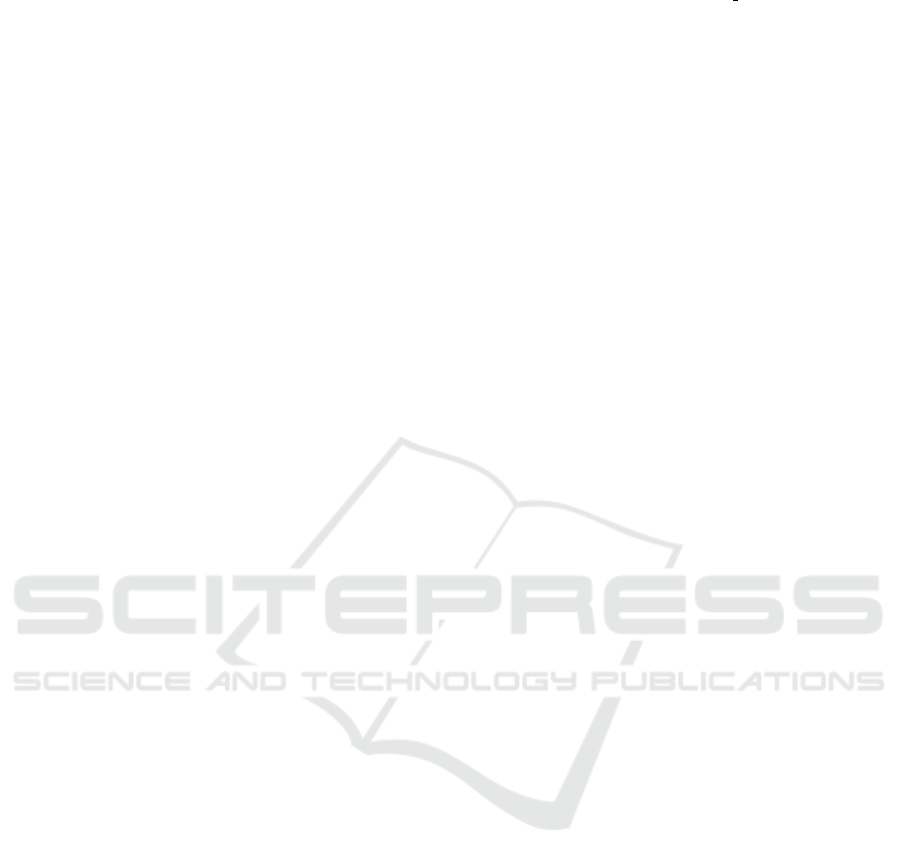
Anderson, C. (2006). The Long Tail: Why the Future of
Business Is Selling Less of More. Hyperion.
Bobadilla, J., Ortega, F., Hernando, A., and Guti
´
errez,
A. (2013). Recommender systems sur-
vey. Knowledge-Based Systems, 46:109–132.
https://www.sciencedirect.com/science/article/abs/
pii/S0950705113001044.
Bolch, G., Greiner, S., de Meer, H., and Trivedi, K. S.
(1998). Queueing Networks and Markov Chains:
Modeling and Performance Evaluation with Com-
puter Science Applications. Wiley-Interscience, New
York, NY, USA.
Brynjolfsson, E. (2011). Goodbye pareto principle, hello
long tail: The effect of search costs on the concentra-
tion of product sales. Management Science, 57(8).
Burke, R. (2002). Sistemas de recomendac¸
˜
ao h
´
ıbridos: Lev-
antamento e experimentos. User Modeling and User-
Adapted Interaction, 12(4):331–370.
de Sousa Silva, D. V., de Oliveira, A. C., Almeida,
F., and Dur
˜
ao, F. A. (2020). Explorando similar-
idades em grafos com agrupamento para melhorar
recomendac¸
˜
oes de itens de cauda longa. In de Salles
Soares Neto, C., editor, WebMedia ’20: Simp
´
osio
Brasileiro sobre Multim
´
ıdia e a Web, pages 193–200,
S
˜
ao Lu
´
ıs, Brasil. ACM.
Gomez-Uribe, C. A. and Hunt, N. (2015). The netflix rec-
ommender system: Algorithms, business value, and
innovation. ACM Transactions on Management Infor-
mation Systems, 6(4):13:1–13:19.
Harper, F. M. and Konstan, J. A. (2015). The movielens
datasets: History and context. ACM Trans. Interact.
Intell. Syst., 5(4):19:1–19:19.
Likert, R. (1932). A technique for the measurement of atti-
tudes. Archives of Psychology.
Lin, J., Chen, S., and Wang, J. (2022). Graph neural net-
works with dynamic and static representations for so-
cial recommendation. In et al., A. B., editor, Database
Systems for Advanced Applications, pages 264–271,
Cham. Springer International Publishing.
Luke, A., Johnson, J., and Ng, Y.-K. (2018). Recommend-
ing long-tail items using extended tripartite graphs. In
Proceedings of the 2018 IEEE International Confer-
ence on Big Knowledge (ICBK), pages 123–130, Sin-
gapore.
Pang, J., Guo, J., and Zhang, W. (2019). Using multi-
objective optimization to solve the long tail problem
in recommender system. In Yang, Q., Zhou, Z.-H.,
Gong, Z., Zhang, M.-L., and Huang, S.-J., editors,
Advances in Knowledge Discovery and Data Mining,
pages 302–313, Cham. Springer International Pub-
lishing.
Qin, J. (2021). A survey of long-tail item recommendation
methods. Wireless Communications and Mobile Com-
puting. [Online]. Available: https://doi.org/10.1155/
2021/7536316.
Qin, J., Zhang, Q., and Wang, B. (2020). Recommenda-
tion method with focus on long tail items. Journal of
Computer Applications, 40(2):454–458.
Snyder, K. (2024). 35 e-commerce statistics of
2024. https://www.forbes.com/advisor/business/
ecommerce-statistics/#sources section. Accessed:
2024-06-06.
Sreepada, R. S. and Patra, B. K. (2021). Enhancing long
tail item recommendation in collaborative filtering:
An econophysics-inspired approach. Electronic Com-
merce Research and Applications, 49:101089.
Wang, F., Ma, S., Yang, L., and Li, T. (2006). Recommen-
dation on item graphs. In Proceedings of the Sixth In-
ternational Conference on Data Mining (ICDM’06),
pages 1119–1123.
Yadav, N., Pal, S., Singh, A. K., and Singh, K. (2022). Clus-
dr: Cluster-based pre-trained model for diverse rec-
ommendation generation. Journal of King Saud Uni-
versity - Computer and Information Sciences, 34(8,
Part B):6385–6399.
Yamashita, K., McIntosh, S., Kamei, Y., Hassan, A. E.,
and Ubayashi, N. (2015). Revisiting the applicabil-
ity of the pareto principle to core development teams
in open source software projects. In Proceedings of
the 14th International Workshop on Principles of Soft-
ware Evolution (IWPSE 2015), pages 46–55, Berg-
amo, Italy. ACM.
Yang, L., Schnabel, T., Bennett, P. N., and Dumais, S.
(2021). Local factor models for large-scale induc-
tive recommendation. In Fifteenth ACM Conference
on Recommender Systems (RecSys ’21), pages 252–
262, New York, NY, USA. Association for Computing
Machinery.
Yin, H., Cui, B., Li, J., Yao, J., and Chen, C. (2012). Chal-
lenging the long tail recommendation. In Proc. VLDB
Endow., volume 5, pages 896–907.
WEBIST 2024 - 20th International Conference on Web Information Systems and Technologies
58