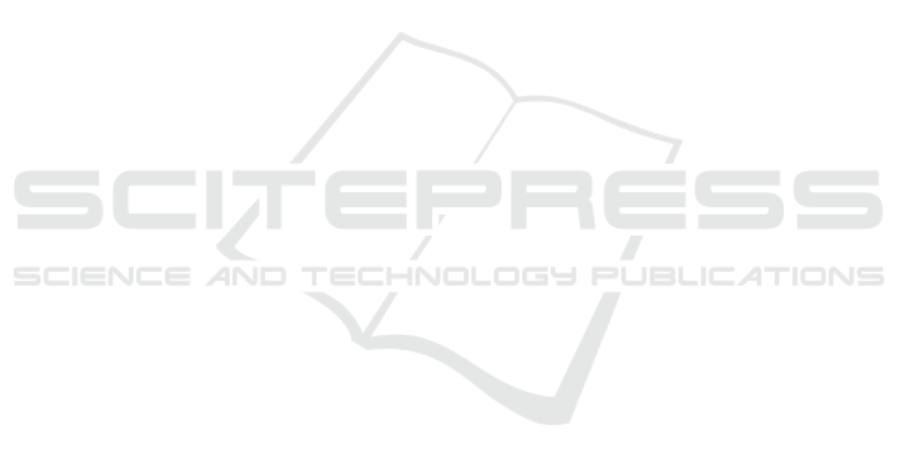
CNN for tumor brain classification. Diagnostics,
13(12):2050.
Antonelli, M., Ducange, P., Lazzerini, B., and Marcel-
loni, F. (2016). Multi-objective evolutionary design of
granular rule-based classifiers. Granular Computing,
1:37–58.
Bera, K., Braman, N., Gupta, A., Velcheti, V., and Mad-
abhushi, A. (2022). Predicting cancer outcomes with
radiomics and artificial intelligence in radiology. Na-
ture reviews Clinical oncology, 19(2):132–146.
Cao, J., Zhou, T., Zhi, S., Lam, S., Ren, G., Zhang, Y.,
Wang, Y., Dong, Y., and Cai, J. (2024). Fuzzy infer-
ence system with interpretable fuzzy rules: Advancing
explainable artificial intelligence for disease diagno-
sis—a comprehensive review. Information Sciences,
662:120212.
Carr
´
e, A., Klausner, G., Edjlali, M., Lerousseau, M.,
Briend-Diop, J., Sun, R., Ammari, S., Reuz
´
e, S., Al-
varez Andres, E., Estienne, T., et al. (2020). Stan-
dardization of brain MR images across machines and
protocols: bridging the gap for MRI-based radiomics.
Scientific reports, 10(1):12340.
Cheng, J., Huang, W., Cao, S., Yang, R., Yang, W.,
Yun, Z., Wang, Z., and Feng, Q. (2015). En-
hanced performance of brain tumor classification via
tumor region augmentation and partition. PloS one,
10(10):e0140381.
Chmiel, W., Kwiecie
´
n, J., and Motyka, K. (2023). Saliency
map and deep learning in binary classification of brain
tumours. Sensors, 23(9):4543.
Cho, H.-h., Lee, S.-h., Kim, J., and Park, H. (2018). Classi-
fication of the glioma grading using radiomics analy-
sis. PeerJ, 6:e5982.
Decuyper, M., Bonte, S., and Van Holen, R. (2018). Binary
glioma grading: radiomics versus pre-trained cnn fea-
tures. In Medical Image Computing and Computer As-
sisted Intervention–MICCAI 2018: 21st International
Conference, Granada, Spain, September 16-20, 2018,
Proceedings, Part III 11, pages 498–505. Springer.
Du, P., Wu, X., Liu, X., Chen, J., Chen, L., Cao, A., and
Geng, D. (2023). The application of decision tree
model based on clinicopathological risk factors and
pre-operative mri radiomics for predicting short-term
recurrence of glioblastoma after total resection: a ret-
rospective cohort study. American Journal of Cancer
Research, 13(8):3449.
Ghamry, F. M., Emara, H. M., Hagag, A., El-Shafai, W.,
El-Banby, G. M., Dessouky, M. I., El-Fishawy, A. S.,
El-Hag, N. A., and El-Samie, F. E. A. (2023). Efficient
algorithms for compression and classification of brain
tumor images. Journal of Optics, 52(2):818–830.
Hulsen, T. (2023). Explainable artificial intelligence (XAI):
Concepts and challenges in healthcare. AI, 4(3):652–
666.
Kaifi, R. (2023). A review of recent advances in brain tumor
diagnosis based on AI-based classification. Diagnos-
tics, 13(18):3007.
Kalam, R., Thomas, C., and Rahiman, M. A. (2023). Brain
tumor detection in MRI images using adaptive-anfis
classifier with segmentation of tumor and edema. Soft
Computing, 27(5):2279–2297.
Khalighi, S., Reddy, K., Midya, A., Pandav, K. B., Madab-
hushi, A., and Abedalthagafi, M. (2024). Artificial
intelligence in neuro-oncology: advances and chal-
lenges in brain tumor diagnosis, prognosis, and pre-
cision treatment. NPJ Precision Oncology, 8(1):80.
Khan, M. A., Khan, A., Alhaisoni, M., Alqahtani,
A., Alsubai, S., Alharbi, M., Malik, N. A., and
Dama
ˇ
sevi
ˇ
cius, R. (2023). Multimodal brain tumor
detection and classification using deep saliency map
and improved dragonfly optimization algorithm. In-
ternational Journal of Imaging Systems and Technol-
ogy, 33(2):572–587.
Mandloi, S., Zuber, M., and Gupta, R. K. (2024). An
explainable brain tumor detection and classification
model using deep learning and layer-wise relevance
propagation. Multimedia Tools and Applications,
83(11):33753–33783.
Maqsood, S., Dama
ˇ
sevi
ˇ
cius, R., and Maskeli
¯
unas, R.
(2022). Multi-modal brain tumor detection using
deep neural network and multiclass SVM. Medicina,
58(8):1090.
Muhammad, K., Khan, S., Del Ser, J., and De Albuquerque,
V. H. C. (2020). Deep learning for multigrade brain
tumor classification in smart healthcare systems: A
prospective survey. IEEE Transactions on Neural Net-
works and Learning Systems, 32(2):507–522.
Saidak, Z., Laville, A., Soudet, S., Sevestre, M.-A., Con-
stans, J.-M., and Galmiche, A. (2024). An MRI ra-
diomics approach to predict the hypercoagulable sta-
tus of gliomas. Cancers, 16(7):1289.
Segatori, A., Marcelloni, F., and Pedrycz, W. (2018). On
distributed fuzzy decision trees for big data. IEEE
Transactions on Fuzzy Systems, 26(1):174–192.
Simonyan, K. and Zisserman, A. (2014). Very deep con-
volutional networks for large-scale image recognition.
arXiv preprint arXiv:1409.1556.
Unde, M. and Rathore, A. S. (2024). Brain mri image anal-
ysis for alzheimer’s disease diagnosis using mask r-
cnn. International Journal of Intelligent Systems and
Applications in Engineering, 12(13s):137–149.
Van der Velden, B. H., Kuijf, H. J., Gilhuijs, K. G., and
Viergever, M. A. (2022). Explainable artificial in-
telligence (xai) in deep learning-based medical image
analysis. Medical Image Analysis, 79:102470.
Wang, A. Q., Karaman, B. K., Kim, H., Rosenthal, J.,
Saluja, R., Young, S. I., and Sabuncu, M. R. (2024).
A framework for interpretability in machine learning
for medical imaging. IEEE Access.
Zhang, Y., Yang, D., Lam, S., Li, B., Teng, X., Zhang,
J., Zhou, T., Ma, Z., Ying, T.-C., and Cai, J. (2022).
Radiomics-based detection of covid-19 from chest X-
ray using interpretable soft label-driven TSK fuzzy
classifier. Diagnostics, 12(11):2613.
Zhou, S. K., Greenspan, H., and Shen, D. (2023). Deep
learning for medical image analysis. Academic Press.
Zwanenburg, A., Valli
`
eres, M., Abdalah, M. A., Aerts,
H. J., Andrearczyk, V., Apte, A., Ashrafinia, S.,
Bakas, S., Beukinga, R. J., Boellaard, R., et al. (2020).
The image biomarker standardization initiative: stan-
dardized quantitative radiomics for high-throughput
image-based phenotyping. Radiology, 295(2):328–
338.
Deep Learning and Multi-Objective Evolutionary Fuzzy Classifiers: A Comparative Analysis for Brain Tumor Classification in MRI Images
115