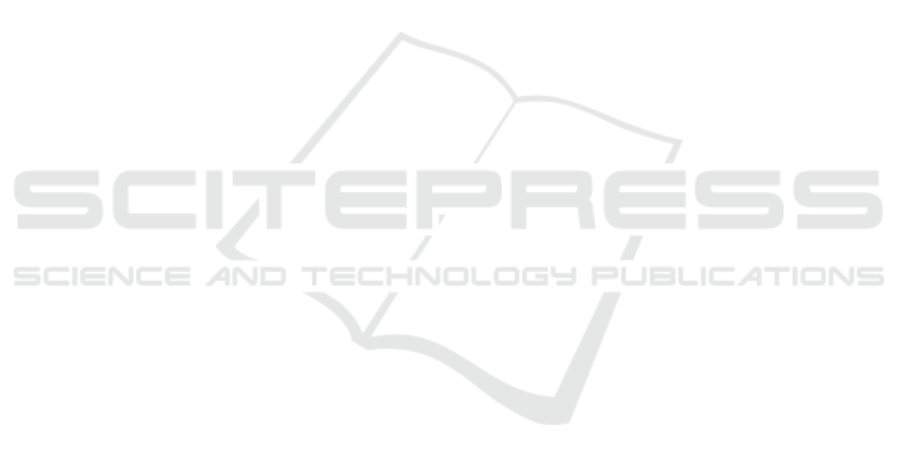
Arnaout, R., Curran, L., Zhao, Y., Levine, J. C., Chinn, E.,
and Moon-Grady, A. J. (2021). An ensemble of neural
networks provides expert-level prenatal detection of
complex congenital heart disease. Nature Medicine.
Barda, A., Horvat, C., and Hochheiser, H. (2020). A
qualitative research framework for the design of user-
centered displays of explanations for machine learn-
ing model predictions in healthcare. BMC medical in-
formatics and decision making, 20:257.
Borys, K., Schmitt, Y. A., Nauta, M., Seifert, C., Kr
¨
amer,
N., Friedrich, C. M., and Nensa, F. (2023). Explain-
able AI in medical imaging: An overview for clinical
practitioners – saliency-based XAI approaches. Euro-
pean Journal of Radiology, 162:110787.
Cerekci, E., Alis, D., Denizoglu, N., Camurdan, O., Ege
Seker, M., Ozer, C., Hansu, M. Y., Tanyel, T., Ok-
suz, I., and Karaarslan, E. (2024). Quantitative eval-
uation of saliency-based explainable artificial intelli-
gence (XAI) methods in deep learning-based mam-
mogram analysis. European Journal of Radiology,
173:111356.
Chattopadhay, A., Sarkar, A., Howlader, P., and Balasub-
ramanian, V. N. (2018). Grad-cam++: Generalized
gradient-based visual explanations for deep convolu-
tional networks. In 2018 IEEE winter conference on
applications of computer vision (WACV), pages 839–
847. IEEE.
Cooper, J., Arandjelovi
´
c, O., and Harrison, D. J. (2022).
Believe the HiPe: Hierarchical perturbation for fast,
robust, and model-agnostic saliency mapping. Pattern
Recognition, 129:108743.
Cruz-Roa, A., Basavanhally, A., Gonz
´
alez, F., Gilmore, H.,
Feldman, M., Ganesan, S., Shih, N., Tomaszewski, J.,
and Madabhushi, A. (2014). Automatic detection of
invasive ductal carcinoma in whole slide images with
convolutional neural networks. Progress in Biomedi-
cal Optics and Imaging - Proceedings of SPIE, 9041.
DeGrave, A. J., Janizek, J. D., and Su-In, L. (2021). Ai
for radiographic COVID-19 detection selects short-
cuts over signal. Nature Machine Intelligence.
Doshi-Velez, F. and Kim, B. (2017). Towards a rigorous
science of interpretable machine learning.
Geirhos, R., Jacobsen, J.-H., Michaelis, C., Zemel, R. S.,
Brendel, W., Bethge, M., and Wichmann, F. (2020).
Shortcut learning in deep neural networks. Nature
Machine Intelligence, 2:665 – 673.
Guidotti, R., Monreale, A., Ruggieri, S., Turini, F., Gian-
notti, F., and Pedreschi, D. (2018). A survey of meth-
ods for explaining black box models. ACM Comput.
Surv., 51(5).
Hannun, A. Y., Rajpurkar, P., Haghpanahi, M., Tison, G. H.,
Bourn, C., Turakhia, M. P., and Ng, A. Y. (2019).
Cardiologist-level arrhythmia detection and classifi-
cation in ambulatory electrocardiograms using a deep
neural network. Nature Medicine, 25(1):65–69.
Holzinger, A., Biemann, C., Pattichis, C., and Kell, D.
(2017). What do we need to build explainable ai sys-
tems for the medical domain?
Hwang, J., Lee, T., Lee, H., and Byun, S. (2022). A clin-
ical decision support system for sleep staging tasks
with explanations from artificial intelligence: User-
centered design and evaluation study. J Med Internet
Res, 24(1):e28659.
Krizhevsky, A., Sutskever, I., and Hinton, G. E. (2012). Im-
agenet classification with deep convolutional neural
networks. Advances in neural information processing
systems, 25.
Lapuschkin, S., W
¨
aldchen, S., Binder, A., Montavon, G.,
Samek, W., and M
¨
uller, K.-R. (2019). Unmasking
clever hans predictors and assessing what machines
really learn. Nature Communications, 10(1):1096.
LeCun, Y., Bottou, L., Bengio, Y., and Haffner, P. (1998).
Gradient-based learning applied to document recogni-
tion. Proceedings of the IEEE, 86(11):2278–2324.
Litjens, G., Kooi, T., Bejnordi, B. E., Setio, A. A. A.,
Ciompi, F., Ghafoorian, M., van der Laak, J. A., van
Ginneken, B., and S
´
anchez, C. I. (2017). A survey
on deep learning in medical image analysis. Medical
Image Analysis, 42:60–88.
Magrabi, F., Ammenwerth, E., McNair, J. B., Keizer, N.
F. D., Hypp
¨
onen, H., Nyk
¨
anen, P., Rigby, M., Scott,
P. J., Vehko, T., Wong, Z. S., and Georgiou, A.
(2019). Artificial intelligence in clinical decision sup-
port: Challenges for evaluating ai and practical impli-
cations. Yearbook of Medical Informatics, 28(1):128–
134. Epub 2019 Apr 25.
Petsiuk, V., Das, A., and Saenko, K. (2018). Rise: Ran-
domized input sampling for explanation of black-box
models. arXiv preprint arXiv:1806.07421.
Raghu, M. and Schmidt, E. (2020). A survey of deep learn-
ing for scientific discovery.
Ribeiro, M. T., Singh, S., and Guestrin, C. (2016). ”why
should i trust you?”: Explaining the predictions of any
classifier.
Samek, W., Montavon, G., Lapuschkin, S., Anders, C. J.,
and M
¨
uller, K.-R. (2021). Explaining deep neural net-
works and beyond: A review of methods and applica-
tions. Proceedings of the IEEE, 109(3):247–278.
Scherer, D., M
¨
uller, A., and Behnke, S. (2010). Evaluation
of pooling operations in convolutional architectures
for object recognition. In International conference on
artificial neural networks, pages 92–101. Springer.
Selvaraju, R. R., Das, A., Vedantam, R., Cogswell, M.,
Parikh, D., and Batra, D. (2016). Grad-CAM: Why
did you say that? arXiv preprint arXiv:1611.07450.
Shen, D., Wu, G., and Suk, H.-I. (2017). Deep learning in
medical image analysis. Annual Review of Biomedical
Engineering, 19(1):221–248. PMID: 28301734.
Shinde, P. P. and Shah, S. (2018). A review of ma-
chine learning and deep learning applications. In
2018 Fourth International Conference on Comput-
ing Communication Control and Automation (IC-
CUBEA), pages 1–6.
Shortliffe, E. H. and Sep
´
ulveda, M. J. (2018). Clinical
Decision Support in the Era of Artificial Intelligence.
JAMA, 320(21):2199–2200.
Simonyan, K., Vedaldi, A., and Zisserman, A. (2014).
Deep inside convolutional networks: Visualising im-
age classification models and saliency maps.
EXPLAINS 2024 - 1st International Conference on Explainable AI for Neural and Symbolic Methods
36