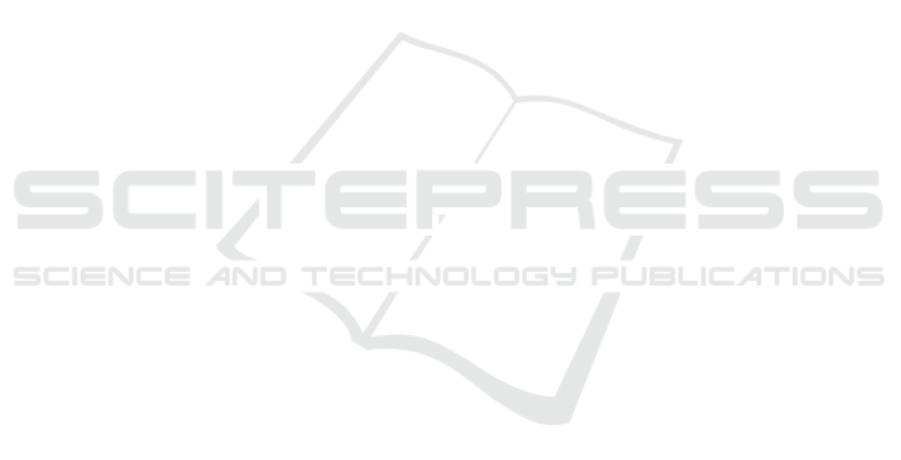
tried to counteract this behavior by fixing the sensor
as securely as possible to the subject without hinder-
ing the runner. Since there are no consumer-ready 5G
IMU sensors available the choice of smartphones as a
replacement was only pragmatic.
One of the benefits of a distributed BSN is the
increased processing power on the off-site process-
ing device. Nonetheless, the gait-symmetry algorithm
can hardly be described as very resource-intensive. It
would certainly be possible to do the same calcula-
tions on the smartphones themselves. This was done
intentionally, as the point of this work was mainly to
assess the overall performance of a distributed 5G-
based BSN biofeedback system.
In the future it would be interesting to leverage
the increased (off-site) processing power and include
more resource intense machine learning algorithms
and new physiological sensor data like skin temper-
ature, power or heart-rate.
Another critical point is the fact that the system
was tested with only one BSN in the 5G network. This
was done to eliminate potential interactions of devices
and effects on the performance of the 5G network. In
the future, a comparison of the behavior of the net-
work and the time delays in scenarios with several si-
multaneously active devices would be interesting.
ACKNOWLEDGEMENTS
This project is partially funded by the Austrian state
of Salzburg under the program “WISS 2025” contract
number 20102-F2001049-FPR.
REFERENCES
Attar, H., Issa, H., Ababneh, J., Abbasi, M., Solyman, A.
A. A., Khosravi, M., and Said Agieb, R. (2022). 5G
System Overview for Ongoing Smart Applications:
Structure, Requirements, and Specifications. Compu-
tational Intelligence and Neuroscience, 2022:1–11.
Grouios, G., Ziagkas, E., Loukovitis, A., Chatzinikolaou,
K., and Koidou, E. (2022). Accelerometers in Our
Pocket: Does Smartphone Accelerometer Technology
Provide Accurate Data? Sensors (Basel, Switzerland),
23(1):192.
James, C., Lam, G. W., Guppy, F., Mu
˜
niz-Pardos, B., An-
geloudis, K., Keramitsoglou, I., Knopp, M., Ruiz, D.,
Racinais, S., and Pitsiladis, Y. (2024). The integra-
tion of multi-sensor wearables in elite sport. Gatorade
Sports Science Exchange, 37:1–10.
Lai, X., Liu, Q., Wei, X., Wang, W., Zhou, G., and Han, G.
(2013). A Survey of Body Sensor Networks. Sensors
(Basel, Switzerland), 13(5):5406–5447.
Lee, J. B., Sutter, K. J., Askew, C. D., and Burkett, B. J.
(2010). Identifying symmetry in running gait using a
single inertial sensor. Journal of Science and Medicine
in Sport, 13(5):559–563.
Radzak, K. N., Putnam, A. M., Tamura, K., Hetzler, R. K.,
and Stickley, C. D. (2017). Asymmetry between lower
limbs during rested and fatigued state running gait in
healthy individuals. Gait & Posture, 51:268–274.
Sigrist, R., Rauter, G., Riener, R., and Wolf, P. (2013). Aug-
mented visual, auditory, haptic, and multimodal feed-
back in motor learning: A review. Psychonomic Bul-
letin & Review, 20(1):21–53.
Tanenbaum, A. S. and Wetherall, D. J. (2010). Computer
Networks. Pearson, Boston Amsterdam, 5 edition.
Telekom-Control-Kommission (TKK) (2018). Ausschrei-
bungsunterlage im Verfahren betreffend Frequenz-
zuteilungen im Frequenzbereich 3410 bis 3800 MHz.
Tenforde, A. S., Borgstrom, H. E., Outerleys, J., and Davis,
I. S. (2019). Is Cadence Related to Leg Length and
Load Rate? Journal of Orthopaedic & Sports Physi-
cal Therapy, 49(4):280–283.
Umek, A. and Kos, A. (2016). The Role of High Perfor-
mance Computing and Communication for Real-Time
Biofeedback in Sport. Mathematical Problems in En-
gineering, 2016:1–11.
Van Hooren, B., Goudsmit, J., Restrepo, J., and Vos, S.
(2020). Real-time feedback by wearables in run-
ning: Current approaches, challenges and sugges-
tions for improvements. Journal of Sports Sciences,
38(2):214–230.
Virtanen, P., Gommers, R., Oliphant, T. E., Haberland, M.,
Reddy, T., Cournapeau, D., Burovski, E., Peterson, P.,
Weckesser, W., Bright, J., van der Walt, S. J., Brett,
M., Wilson, J., Millman, K. J., Mayorov, N., Nel-
son, A. R. J., Jones, E., Kern, R., Larson, E., Carey,
C. J., Polat,
˙
I., Feng, Y., Moore, E. W., VanderPlas,
J., Laxalde, D., Perktold, J., Cimrman, R., Henriksen,
I., Quintero, E. A., Harris, C. R., Archibald, A. M.,
Ribeiro, A. H., Pedregosa, F., van Mulbregt, P., and
SciPy 1.0 Contributors (2020). SciPy 1.0: Fundamen-
tal algorithms for scientific computing in python. Na-
ture Methods, 17:261–272.
Zijlstra, W. and Hof, A. L. (2003). Assessment of spatio-
temporal gait parameters from trunk accelerations
during human walking. Gait and Posture, 18(2):1–10.
icSPORTS 2024 - 12th International Conference on Sport Sciences Research and Technology Support
184