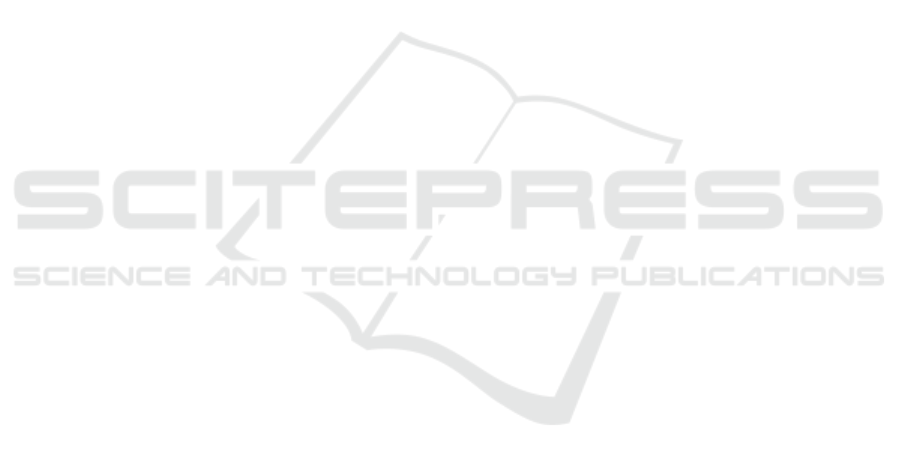
generation for large language models: A survey. arXiv
preprint arXiv:2312.10997.
Garcia, G. L., Paiola, P. H., Morelli, L. H., Candido, G.,
J
´
unior, A. C., Jodas, D. S., Afonso, L., Guilherme,
I. R., Penteado, B. E., and Papa, J. P. (2024). In-
troducing bode: A fine-tuned large language model
for portuguese prompt-based task. arXiv preprint
arXiv:2401.02909.
Gu, Y., Han, X., Liu, Z., and Huang, M. (2021). Ppt: Pre-
trained prompt tuning for few-shot learning. arXiv
preprint arXiv:2109.04332.
Hu, E. J., Shen, Y., Wallis, P., Allen-Zhu, Z., Li, Y., Wang,
S., Wang, L., and Chen, W. (2021). Lora: Low-rank
adaptation of large language models. arXiv preprint
arXiv:2106.09685.
Kieckbusch, D. S., Geraldo Filho, P., Di Oliveira, V., and
Weigang, L. (2021). Scan-nf: A cnn-based system for
the classification of electronic invoices through short-
text product description. In WEBIST, pages 501–508.
Larcher, C., Piau, M., Finardi, P., Gengo, P., Esposito, P.,
and Carid
´
a, V. (2023). Cabrita: closing the gap for
foreign languages. arXiv preprint arXiv:2308.11878.
Lewis, P., Perez, E., Piktus, A., Petroni, F., Karpukhin,
V., Goyal, N., K
¨
uttler, H., Lewis, M., Yih, W.-t.,
Rockt
¨
aschel, T., et al. (2020). Retrieval-augmented
generation for knowledge-intensive nlp tasks. Ad-
vances in Neural Information Processing Systems,
33:9459–9474.
Lopes, R., Magalh
˜
aes, J., and Semedo, D. (2024). Gl\’oria-
a generative and open large language model for por-
tuguese. arXiv preprint arXiv:2402.12969.
Ma, H., Zhang, C., Bian, Y., Liu, L., Zhang, Z., Zhao,
P., Zhang, S., Fu, H., Hu, Q., and Wu, B. (2024).
Fairness-guided few-shot prompting for large lan-
guage models. Advances in Neural Information Pro-
cessing Systems, 36.
Marinho, M. C., Di Oliveira, V., Neto, S. A., Weigang, L.,
and Borges, V. R. (2022). Visual analysis of electronic
invoices to identify suspicious cases of tax frauds. In
International Conference on Information Technology
& Systems, pages 185–195. Springer.
MERCOSUR (2024a). Mercosur - consultas
`
a nomen-
clatura comum e
`
a tarifa externa. https://www.
mercosur.int/pt-br/politica-comercial/ncm/ Accessed
on Jun 4th, 2024.
MERCOSUR (2024b). Mercosur contries. https://www.
mercosur.int/en/about-mercosur/mercosur-countries/
Accessed on Jun 6th, 2024.
Meta, A. (2024). Introducing meta llama 3: The most ca-
pable openly available llm to date. Last accessed June
3th, 2024 https://ai.meta.com/blog/meta-llama-3/.
Pires, R., Abonizio, H., Almeida, T. S., and Nogueira, R.
(2023). Sabi
´
a: Portuguese large language models.
In Brazilian Conference on Intelligent Systems, pages
226–240. Springer.
Radosavovic, I., Zhang, B., Shi, B., Rajasegaran, J., Kamat,
S., Darrell, T., Sreenath, K., and Malik, J. (2024). Hu-
manoid locomotion as next token prediction. arXiv
preprint arXiv:2402.19469.
Schulhoff, S., Ilie, M., Balepur, N., Kahadze, K., Liu,
A., Si, C., Li, Y., Gupta, A., Han, H., Schulhoff,
S., et al. (2024). The prompt report: A system-
atic survey of prompting techniques. arXiv preprint
arXiv:2406.06608.
Schulte, J. P., Giuntini, F. T., Nobre, R. A., Nascimento, K.
C. d., Meneguette, R. I., Li, W., Gonc¸alves, V. P., and
Rocha Filho, G. P. (2022). Elinac: autoencoder ap-
proach for electronic invoices data clustering. Applied
Sciences, 12(6):3008.
Souza, F., Nogueira, R., and Lotufo, R. (2020). Bertimbau:
pretrained bert models for brazilian portuguese. In In-
telligent Systems: 9th Brazilian Conference, BRACIS
2020, Rio Grande, Brazil, October 20–23, 2020, Pro-
ceedings, Part I 9, pages 403–417. Springer.
Touvron, H., Lavril, T., Izacard, G., Martinet, X., Lachaux,
M.-A., Lacroix, T., Rozi
`
ere, B., Goyal, N., Hambro,
E., Azhar, F., et al. (2023a). Llama: Open and ef-
ficient foundation language models. arXiv preprint
arXiv:2302.13971.
Touvron, H., Martin, L., Stone, K., Albert, P., Almahairi,
A., Babaei, Y., Bashlykov, N., Batra, S., Bhargava,
P., Bhosale, S., et al. (2023b). Llama 2: Open foun-
dation and fine-tuned chat models. arXiv preprint
arXiv:2307.09288.
Valenc¸a, P. R. M. et al. (2023). Essays on foreign trade,
labor, innovation and environment.
Van Engelen, J. E. and Hoos, H. H. (2020). A sur-
vey on semi-supervised learning. Machine learning,
109(2):373–440.
Vaswani, A., Shazeer, N., Parmar, N., Uszkoreit, J., Jones,
L., Gomez, A. N., Kaiser, Ł., and Polosukhin, I.
(2017). Attention is all you need. Advances in neural
information processing systems, 30.
Warnakulasuriya, S. and Hapuarachchi, K. (2024). From
knowledge to action: Leveraging retrieval augmented
fine tuning (raft) to empower quick and confident first-
aid decisions in emergencies. Researchgate (Preprint)
http://dx.doi.org/10.13140/RG.2.2.35911.30888.
WCO (2018). THE HARMONIZED SYSTEM A universal
language for international trade. World Customs
Organization. https://www.wcoomd.org/-/media/
wco/public/global/pdf/topics/nomenclature/activities-
and-programmes/30-years-hs/hs-compendium.pdf
(Visited 2024-06-04).
WCO (2024). List of contracting parties to the hs con-
vention and countries using the hs - world customs
organization. https://www.wcoomd.org/en/topics/
nomenclature/overview/list-of-contracting-parties-
to-the-hs-convention-and-countries-using-the-
hs.aspx Accessed on Jun 6th, 2024.
Yadav, B. K. (2023). Impact of regulation and conformity
assessment procedures on global trade. In Handbook
of Quality System, Accreditation and Conformity As-
sessment, pages 1–21. Springer.
Zhang, T., Patil, S. G., Jain, N., Shen, S., Zaharia, M., Sto-
ica, I., and Gonzalez, J. E. (2024). Raft: Adapting
language model to domain specific rag. arXiv preprint
arXiv:2403.10131.
SLIM-RAFT: A Novel Fine-Tuning Approach to Improve Cross-Linguistic Performance for Mercosur Common Nomenclature
241