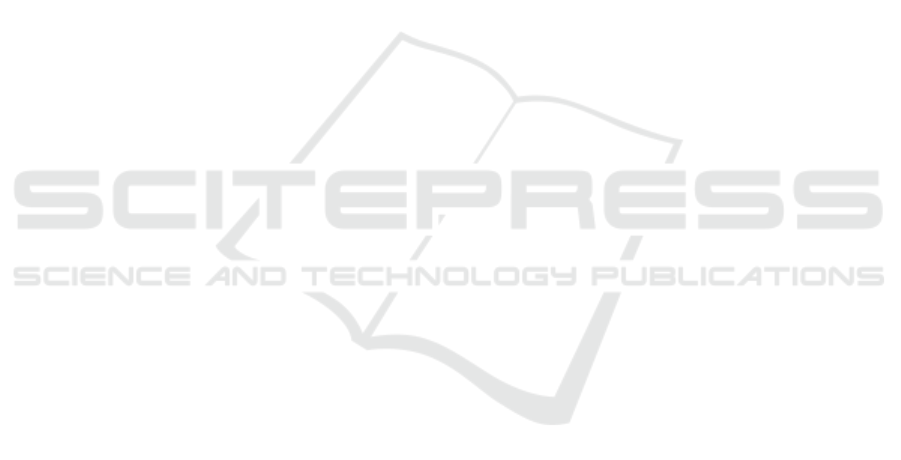
Barsocchi, P., Crivello, A., La Rosa, D., and Palumbo, F.
(2016). A multisource and multivariate dataset for
indoor localization methods based on wlan and geo-
magnetic field fingerprinting. In 2016 International
Conference on Indoor Positioning and Indoor Navi-
gation (IPIN), pages 1–8. IEEE.
Berahmand, K., Mohammadi, M., Faroughi, A., and Mo-
hammadiani, R. P. (2022). A novel method of spec-
tral clustering in attributed networks by constructing
parameter-free affinity matrix. Cluster Computing,
pages 1–20.
Cui, Z., Jing, X., Zhao, P., Zhang, W., and Chen, J. (2021).
A new subspace clustering strategy for ai-based data
analysis in iot system. IEEE Internet of Things Jour-
nal, 8(16):12540–12549.
El Khediri, S., Fakhet, W., Moulahi, T., Khan, R., Thaljaoui,
A., and Kachouri, A. (2020). Improved node local-
ization using k-means clustering for wireless sensor
networks. Computer Science Review, 37:100284.
Ester, M., Kriegel, H.-P., Sander, J., Xu, X., et al. (1996).
A density-based algorithm for discovering clusters in
large spatial databases with noise. In kdd, volume 96,
pages 226–231.
Fang, S.-G., Huang, D., Cai, X.-S., Wang, C.-D., He, C.,
and Tang, Y. (2023). Efficient multi-view clustering
via unified and discrete bipartite graph learning. IEEE
Transactions on Neural Networks and Learning Sys-
tems.
Junyi, G., Li, S., Xiongxiong, H., and Jiajia, C. (2021). A
novel clustering algorithm by adaptively merging sub-
clusters based on the normal-neighbor and merging
force. Pattern Analysis and Applications, 24(3):1231–
1248.
Lee, S. G. and Lee, C. (2020). Developing an improved
fingerprint positioning radio map using the k-means
clustering algorithm. In 2020 International Confer-
ence on Information Networking (ICOIN), pages 761–
765. IEEE.
Mavridis, L., Nath, N., and Mitchell, J. B. (2013). Pfclust:
a novel parameter free clustering algorithm. BMC
bioinformatics, 14:1–21.
Paparrizos, J. and Gravano, L. (2015). k-shape: Efficient
and accurate clustering of time series. In Proceedings
of the 2015 ACM SIGMOD international conference
on management of data, pages 1855–1870.
Rafique, H., Almagrabi, A. O., Shamim, A., Anwar, F., and
Bashir, A. K. (2020). Investigating the acceptance of
mobile library applications with an extended technol-
ogy acceptance model (tam). Computers & Educa-
tion, 145:103732.
Rafique, H., Patti, D., Palesi, M., and catania, V. (2023a).
m-bmc: Exploration of magnetic field measurements
for indoor positioning using mini-batch magnetome-
ter calibration. In 2023 First IEEE International Con-
ference on Mobility: Operations, Services, and Tech-
nologies (MOST), pages 55–61. IEEE.
Rafique, H., Patti, D., Palesi, M., La Delfa, G. C., and Cata-
nia, V. (2023b). Optimization technique for indoor
localization: A multi-objective approach to sampling
time and error rate trade-off. In 2023 IEEE Third In-
ternational Conference on Signal, Control and Com-
munication (SCC), pages 01–06. IEEE.
Rafique, H., Ul Islam, Z., and Shamim, A. (2023c). Accep-
tance of e-learning technology by government school
teachers: Application of extended technology accep-
tance model. Interactive Learning Environments,
pages 1–19.
Ren, J., Wang, Y., Niu, C., Song, W., and Huang, S. (2019).
A novel clustering algorithm for wi-fi indoor position-
ing. IEEE Access, 7:122428–122434.
Singh, M. and Soni, S. K. (2019). Fuzzy based novel clus-
tering technique by exploiting spatial correlation in
wireless sensor network. Journal of Ambient Intel-
ligence and Humanized Computing, 10:1361–1378.
Vinciguerra, E., Russo, E., Palesi, M., Ascia, G., and
Rafique, H. (2024). Improving lstm-based indoor po-
sitioning via simulation-augmented geomagnetic field
dataset. In 2024 IEEE International Conference
on Mobility, Operations, Services and Technologies
(MOST), pages 251–259. IEEE.
Vo-Van, T., Nguyen-Hai, A., Tat-Hong, M., and Nguyen-
Trang, T. (2020). A new clustering algorithm and
its application in assessing the quality of underground
water. Scientific Programming, 2020:1–12.
Von Luxburg, U. (2007). A tutorial on spectral clustering.
Statistics and computing, 17:395–416.
Xu, Y., Huang, D., Wang, C.-D., and Lai, J.-H. (2024).
Deep image clustering with contrastive learning and
multi-scale graph convolutional networks. Pattern
Recognition, 146:110065.
ICINCO 2024 - 21st International Conference on Informatics in Control, Automation and Robotics
308