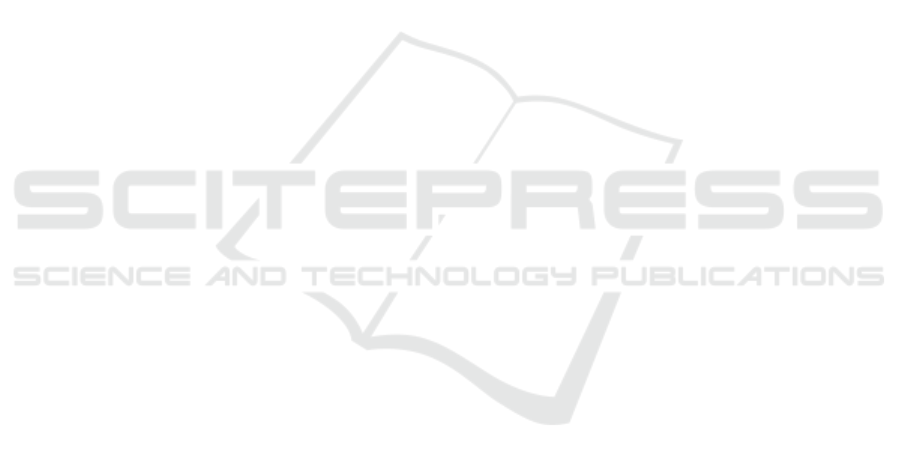
6 CONCLUSION AND FUTURE
WORK
In conclusion, this paper has presented a novel infor-
mation model designed to address the challenges in-
herent in the planning of simulation sequences, ac-
cording to the question raised at the beginning. The
model represents simulations, their ability to generate
specific knowledge, and their respective quality cri-
teria, thereby facilitating the generation of simulation
sequences. The development of the model was guided
by specific requirements and has proven practical in
an application example. This underscores the model’s
utility in providing necessary information to support
users in simulation selection and planning. However,
instantiating the information model requires expertise
in both the semantic web domain and the simulation
domain, as well as a solid understanding of the pro-
posed information model. Furthermore, the model on
its own only offers limited added value to a user, as
it is intended to serve as a foundation for further de-
velopments and applications that build upon it. In
this way the information model presented in this pa-
per paves the way for further research into automated
support for simulation sequence creation, with the ul-
timate goal of reducing the effort required to generate
necessary process information.
In terms of future work, there are several potential
extensions and developments building on the work
presented in this paper. The first aspect is the integra-
tion of the presented information model into a system
that provides an intuitive user interface. This allows
users to interact with the information model in a way
that is significantly useful to them, as the formula-
tion of SPARQL queries is not feasible for most users.
In this context, the question of how the information
model can be automatically instantiated for existing
simulations and during simulation creation could be
examined more closely. The second important area
of development is the creation of a planning logic for
automated selection of simulations and planning of
simulation sequences, as users would benefit signif-
icantly from a higher degree of automation in simu-
lation planning. In this scenario, it could be benefi-
cial to examine the evaluation of quality criteria for
specific use cases more closely, as well as the uncer-
tainties that are inherently associated with the assess-
ment of this quality criteria. Developing a prototype
for the automated generation of simulation sequences
is a practical step towards implementing this plan-
ning logic. As a possible extension to the informa-
tion model it could be beneficial to incorporate a skill
component, meaning the executable implementation
of the abstract functions in addition to the capabili-
ties describing these functions. (K
¨
ocher et al., 2023)
This would allow for a representation of how to exe-
cute simulations. In this context, it would be valuable
to assess the feasibility of automating the execution
of simulations, which would involve evaluating the
extent to which simulation parameterization and ex-
ecution can be automated, identifying limitations or
challenges that may arise.
ACKNOWLEDGEMENTS
This contribution originates from the LaiLa project,
funded by dtec.bw – Digitalization and Technology
Research Center of the Bundeswehr which we grate-
fully acknowledge. dtec.bw is funded by the Euro-
pean Union – NextGenerationEU
REFERENCES
Barth, M., Ristic, M., and J
¨
akel, J. (2023). A role-based
Metric to determine the Quality of Simulation Mod-
els. In 2023 IEEE 28th International Conference
on Emerging Technologies and Factory Automation
(ETFA), volume 2023. IEEE.
DIN EN 61360-1 (07.2018). Standard data element types
with associated classification scheme - Part 1: Defini-
tions - Principles and methods (IEC 61360-1:2017).
Fumagalli, L., Polenghi, A., Negri, E., and Roda, I. (2019).
Framework for simulation software selection. Journal
of Simulation, 13(4):286–303.
Gangemi, A. and Presutti, V. (2009). Ontology Design Pat-
terns. In Staab, S. and Studer, R., editors, Handbook
on Ontologies, pages 221–243. Springer Berlin Hei-
delberg, Berlin, Heidelberg.
Grolinger, K., Capretz, M. A. M., Marti, J. R., and Sri-
vastava, K. D. (2012). Ontology-based Representa-
tion of Simulation Models. In Proceedings of the 24th
International Conference on Software Engineering &
Knowledge Engineering (SEKE’2012), pages 432–
437. Knowledge Systems Institute Graduate School.
Hildebrandt, C., K
¨
ocher, A., Kustner, C., Lopez-Enriquez,
C.-M., Muller, A. W., Caesar, B., Gundlach, C. S.,
and Fay, A. (2020). Ontology Building for Cyber–
Physical Systems: Application in the Manufacturing
Domain. IEEE Transactions on Automation Science
and Engineering, 17(3):1266–1282.
ISO/IEC 25010:2011 (2011). Systems and software egni-
neering - Systems and software Quality Requirements
and Evaluation (SquaRE) - System and software qual-
ity models.
J
¨
arvenp
¨
a
¨
a, E., Siltala, N., Hylli, O., and Lanz, M. (2017).
Capability Matchmaking Procedure to Support Rapid
Configuration and Re-configuration of Production
Systems. Procedia Manufacturing, 11:1053–1060.
Semantic Capability Model for the Simulation of Manufacturing Processes
49