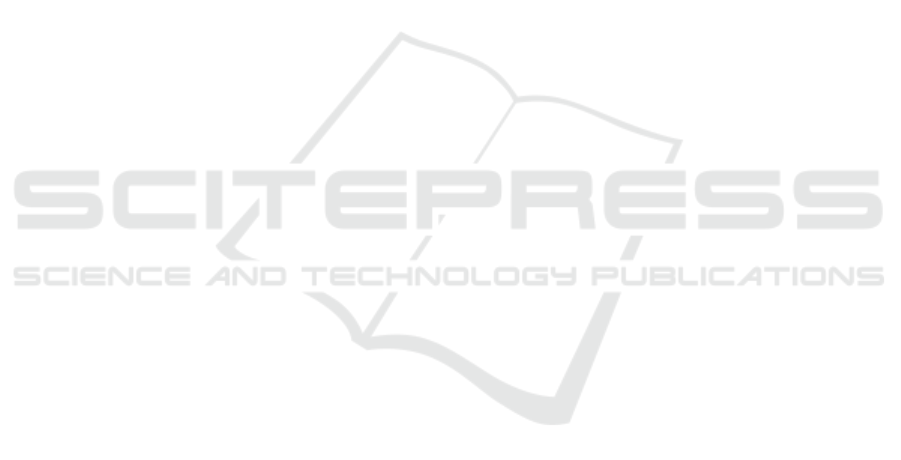
6 CONCLUSIONS AND FUTURE
WORK
In the paper the analysis of the effects of a temporary
increment of a population on the epidemic character-
istics and on the increment of the number of infected
individuals. The flux is assumed coming from a sec-
ond population with its own infection status. A SEIR
model is assumed for describing both the population,
being important to put in evidence the effects on the
infected individuals only. Steady state conditions are
initially considered, with the analysis carried out an-
alytically, giving the conditions for having the classi-
cal epidemic free or endemic status, along with their
stability conditions. A reference to the reproduction
number has been also addressed to evaluate the epi-
demic spread conditions.
While at steady state it is easy to evaluate the ef-
fects of the people flux, being the new equilibria the
combinations of the previous ones, a different ap-
proach has been used to study the transients, trying to
quantify the effects in terms of increment of infected
individuals in population 1. This analysis has been
performed in a numerical way, studying the effects of
the possible different contributions of the people vari-
ation and of the epidemic status of the populations to
the worsening of the infection conditions. Numeri-
cally, the case study in mind has been the analysis of
the possible epidemic effects of the Jubilee 2025 on
the Italian or, suitably scaled, Roman situation during
the year of intense pilgrim/tourists income.
The main result is that it would be necessary to
considered a sanitary prevention plan for an effec-
tive approach to the effects of the disease contain-
ment. Moreover, these considerations can be ex-
tended, qualitatively, to any infectious illness: im-
proving the analytical aspects it is possible to address
a more general class of infections and different pop-
ulations, being possible to extend the results, suitably
scaled, also to several different case like high density
touristic places. Moreover, a more specific analysis
of how and how much these fast increments of pop-
ulations can affect the infection rate β is going to be
faced.
REFERENCES
Casagrandi, R., Bolzoni, L., Levin, S., and Andreasen, V.
(2006). The SIRC model and influenza. A. Mathe-
matical Biosciences, 200:152–169.
Contreras, S., Villavicencio, H., Medina-Ortiz, D., Biron-
Lattes, J., and Olivera-Nappa, A. (2020). A multi-
group seira model for the spread of covid-19 among
heterogeneous populations. Chaos, Solitons and Frac-
tals, 136.
Daley, D. J. and Gani, J. (1999). Epidemic Modelling: An
Introduction. Cambridge Studies in Mathematical Bi-
ology. Cambridge University Press.
Di Giamberardino, P., Caldarella, R., and Iacoviello, D.
(2021a). A control based mathematical model for
the evaluation of intervention lines in COVID-19 epi-
demic spread: The Italian case study. Symmetry,
13(5).
Di Giamberardino, P. and Iacoviello, D. (2017). Optimal
control of SIR epidemic model with state dependent
switching cost index. Biomedical Signal Processing
and Control, 31.
Di Giamberardino, P., Iacoviello, D., Albano, F., and
Frasca, F. (2020). Age based modelling of sars-cov-2
contagion: The italian case. pages 274–279.
Di Giamberardino, P., Iacoviello, D., Papa, F., and Sinis-
galli, C. (2021b). A data-driven model of the covid-19
spread among interconnected populations: epidemio-
logical and mobility aspects following the lockdown
in italy. Nonlinear Dynamics, 106(2):1239–1266.
Iranzo, V. and P
´
erez–Gonz
´
alez, S. (2021). Epidemiological
models and covid-19: a comparative view. Hist Philos
Life Sci., 43(3).
Ledzewicz, U. and Schattler, E. (2011). On optimal sin-
gular controls for a general SIR-model with vaccina-
tion and treatment. Discrete and continuous dynami-
cal systems, pages 981–990.
Martcheva, M. (2015). An introduction to mathemati-
cal epidemiology. Text in Applied Mathematics 61,
Springer.
Ng, O., Marimuthu, K., Chia, P., Koh, V., Chiew, C., and
et al. (2020). SARS-CoV-2 infection among trav-
elers returning from wuhan, china. N Engl J Med.,
382(15):1476–1478.
van den Driessche, P. (2017). Reproduction numbers of
infectious disease models. Infectious Disease Mod-
elling, 2:288–303.
Yue, Y., Tan, Y., and Tang, S. (2023). Stability analysis of
the covid-19 model with age structure under media ef-
fect. Computational and Applied Mathematics, 42(5).
ICINCO 2024 - 21st International Conference on Informatics in Control, Automation and Robotics
578