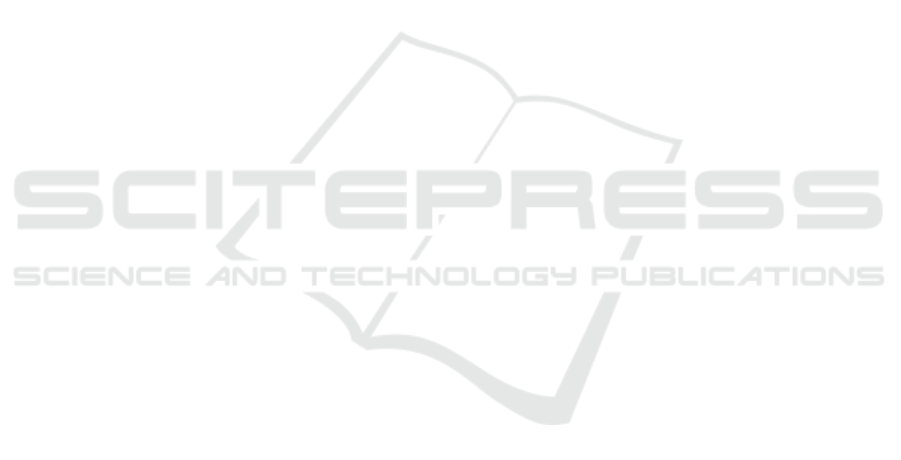
simple text features. Additionally, personalization
improves open rates (Sahni et al., 2016), a finding
corroborated by this research, which identifies the
Sender Open Rate as a pivotal predictor. Moreover,
this work extends prevous work (Balakrishnan and
Parekh, 2014) by integrating sophisticated machine
learning techniques like CatBoost with operational
features and PCA, thereby achieving improved pre-
dictive accuracy. Also, this paper confirms the utility
of deep learning and embeddings (Joshi and Banerjee,
2023), and expands upon by illustrating that combin-
ing these embeddings with operational features effec-
tively boosts model performance.
Future research should focus on enhancing the
model’s capability to handle extreme open rates, pos-
sibly through the use of more detailed datasets and
advanced nonlinear modeling techniques. Exploring
Large Language Models (LLMs) to refine text em-
beddings and their direct integration into regression
tasks could markedly improve prediction accuracy
and adaptability.
Optimizing this model could facilitate real-time
adjustments based on campaign feedback, reducing
reliance on traditional A/B testing, which is often
time-intensive and costly. Such an approach enables
highly personalized strategies that respond dynam-
ically to individual recipient behaviors, optimizing
ROI by enhancing the precision and effectiveness of
email marketing campaigns. By merging cutting-edge
machine learning technologies with email analytics,
this methodology adapts to the evolving dynamics of
consumer interactions in the digital era, offering sig-
nificant enhancements over traditional methods.
Ultimately, this article bridges the gap between
theoretical machine learning techniques and their
practical application in marketing, providing a com-
prehensive methodology for enhancing email cam-
paign strategies through data-driven insights, thus
contributing to a more strategic and economically ef-
ficient approach to digital marketing.
ACKNOWLEDGEMENTS
The authors thank the Pontif
´
ıcia Universidade
Cat
´
olica de Minas Gerais – PUC-Minas and
Coordenac¸
˜
ao de Aperfeic¸oamento de Pessoal de
N
´
ıvel Superior — CAPES (CAPES – Grant PROAP
88887.842889/2023-00 – PUC/MG, Grant PDPG
88887.708960/2022-00 – PUC/MG - Inform
´
atica,
and Finance Code 001).
REFERENCES
Advisor, F. (2024). Best email marketing software 2024.
Balakrishnan, R. and Parekh, R. (2014). Learning to pre-
dict subject-line opens for large-scale email market-
ing. pages 579–584.
Bellman, R. (1961). Adaptive Control Processes: A Guided
Tour. Princeton University Press, Princeton, NJ.
Conceic¸
˜
ao, A. G. d. (2019). Main factors driving the open
rate of email marketing campaigns. Dissertation for
master in modelling, data analysis and decision sup-
port systems, University of Porto, Porto, Portugal.
Etus Media Holding (2024). About Etus Media Holding.
Feld, S., Frenzen, H., Krafft, M., Peters, K., and Verhoef,
P. C. (2013). The effects of mailing design character-
istics on direct mail campaign performance. Interna-
tional Journal of Research in Marketing, 30(2):143–
159.
Hastie, T., Tibshirani, R., and Friedman, J. (2009). The Ele-
ments of Statistical Learning: Data Mining, Inference,
and Prediction. Springer, New York, NY, 2nd edition.
Joshi, S. and Banerjee, I. (2023). Ngram-lstm open rate pre-
diction model (nlorpm): Simple, effective, and easy to
implement approach to predict open rates for market-
ing email. International Journal of Digital Marketing.
Mikolov, T., Chen, K., Corrado, G., and Dean, J. (2013).
Efficient estimation of word representations in vector
space. In Proceedings of the International Conference
on Learning Representations (ICLR).
Miller, R. and Charles, E. (2016). A psychological based
analysis of marketing email subject lines. In 2016
International Conference on Advances in ICT for
Emerging Regions (ICTer), pages 58–65. IEEE.
OpenAI (2024). Openai api.
Paulo, M., Migu
´
eis, V. L., and Pereira, I. (2022). Leverag-
ing email marketing: Using the subject line to antici-
pate the open rate. Expert Systems With Applications,
207:117974.
Sahni, N. S., Wheeler, S. C., and Chintagunta, P. K. (2016).
Personalization in email marketing: The role of non-
informative advertising content. Marketing Science,
35(2):216–233.
Salton, G. and Buckley, C. (1988). Term-weighting ap-
proaches in automatic text retrieval. Information Pro-
cessing & Management, 24(5):513–523.
Stupar-Rutenfrans, S., de Koff, D., and van den Elst, J.
(2019). The effect of subject lines on open rates of
email marketing messages. Advances in Social Sci-
ences Research Journal, 6(7):181–188.
The Radicati Group, I. (2023). Email statistics report, 2023-
2027.
Wainer, J., Dabbish, L., and Kraut, R. (2011). Should i open
this email? In Proceedings of the SIGCHI Conference
on Human Factors in Computing Systems, pages 343–
352. ACM.
WEBIST 2024 - 20th International Conference on Web Information Systems and Technologies
70