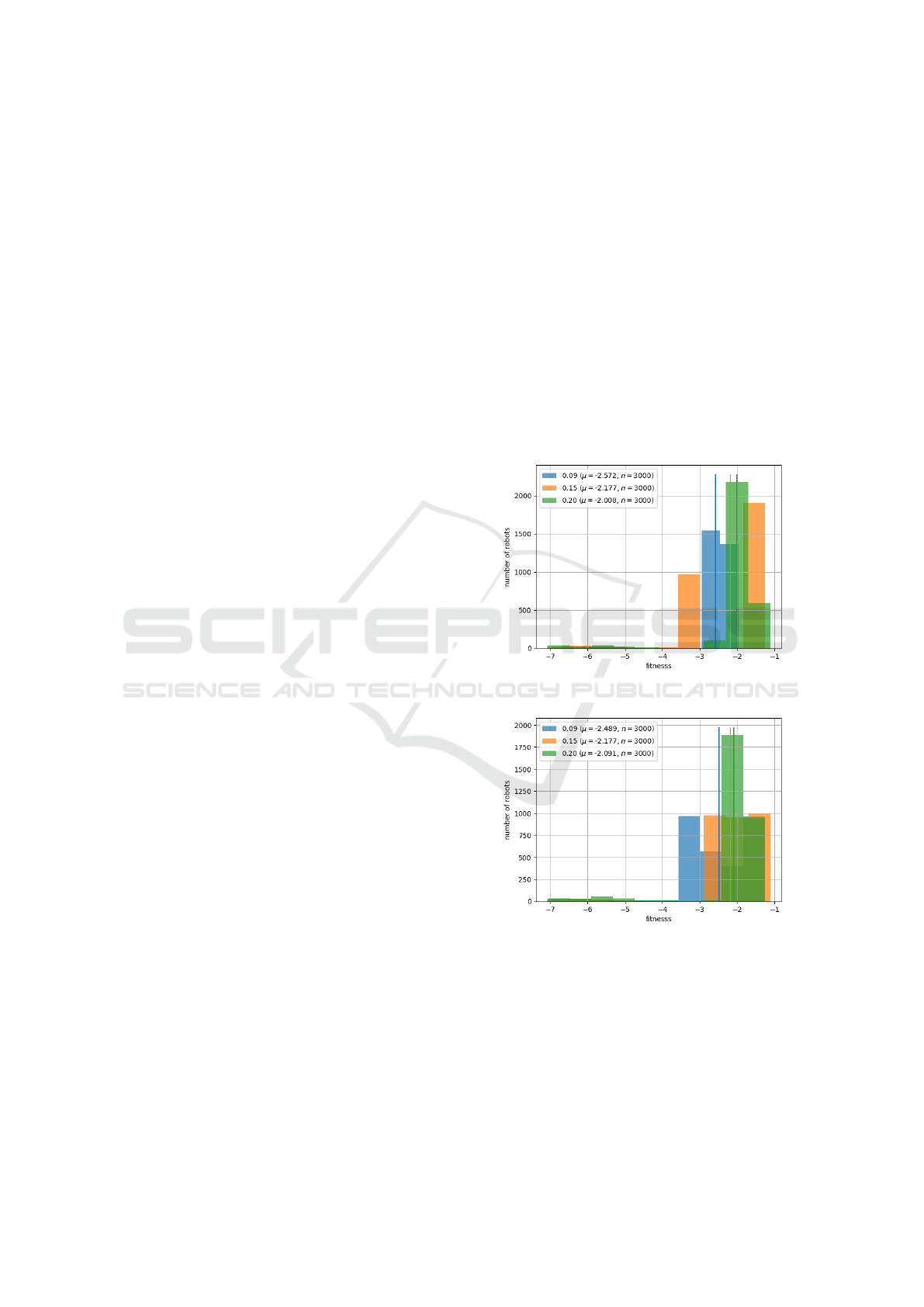
Eiben, A. E. and Smith, J. E. (2015). Introduction to evolu-
tionary computing. Springer.
Finnerty, J. R. (2005). Did internal transport, rather than
directed locomotion, favor the evolution of bilateral
symmetry in animals? BioEssays, 27(11):1174–1180.
Friedman, M. (2008). The evolutionary origin of flatfish
asymmetry. Nature, 454(7201):209–212.
Haasdijk, E., Rusu, A. A., and Eiben, A. E. (2010). Hy-
perneat for locomotion control in modular robots.
In Evolvable Systems: From Biology to Hardware:
9th International Conference, ICES 2010, York, UK,
September 6-8, 2010. Proceedings 9, pages 169–180.
Springer.
Holl
´
o, G. (2015). A new paradigm for animal symmetry.
Interface focus, 5(6):20150032.
Hupkes, E., Jelisavcic, M., and Eiben, A. E. (2018). Re-
volve: a versatile simulator for online robot evolu-
tion. In Applications of Evolutionary Computation:
21st International Conference, EvoApplications 2018,
Parma, Italy, April 4-6, 2018, Proceedings 21, pages
687–702. Springer.
Jelisavcic, M., Glette, K., Haasdijk, E., and Eiben, A.
(2019). Lamarckian evolution of simulated modular
robots. Frontiers in Robotics and AI, 6:9.
Johnston, I. G., Dingle, K., Greenbury, S. F., Camargo,
C. Q., Doye, J. P., Ahnert, S. E., and Louis, A. A.
(2022). Symmetry and simplicity spontaneously
emerge from the algorithmic nature of evolution.
Proceedings of the National Academy of Sciences,
119(11):e2113883119.
Kargar, B. H., Miras, K., and Eiben, A. (2021). The effect of
selecting for different behavioral traits on the evolved
gaits of modular robots. In ALIFE 2021: The 2021
Conference on Artificial Life. MIT Press.
Lan, G., De Carlo, M., van Diggelen, F., Tomczak, J. M.,
Roijers, D. M., and Eiben, A. E. (2021). Learning
directed locomotion in modular robots with evolvable
morphologies. Applied Soft Computing, 111:107688.
Luo, J., Stuurman, A. C., Tomczak, J. M., Ellers, J., and
Eiben, A. E. (2022). The effects of learning in mor-
phologically evolving robot systems. Frontiers in
Robotics and AI, 9:797393.
Miras, K., De Carlo, M., Akhatou, S., and Eiben,
A. (2020a). Evolving-controllers versus learning-
controllers for morphologically evolvable robots. In
Applications of Evolutionary Computation: 23rd Eu-
ropean Conference, EvoApplications 2020, Held as
Part of EvoStar 2020, Seville, Spain, April 15–17,
2020, Proceedings 23, pages 86–99. Springer.
Miras, K., Ferrante, E., and Eiben, A. E. (2020b). Envi-
ronmental influences on evolvable robots. PloS one,
15(5):e0233848.
Miras, K., Haasdijk, E., Glette, K., and Eiben, A. E. (2018).
Search space analysis of evolvable robot morpholo-
gies. In Applications of Evolutionary Computation:
21st International Conference, EvoApplications 2018,
Parma, Italy, April 4-6, 2018, Proceedings 21, pages
703–718. Springer.
Møller, A. P. and Thornhill, R. (1998). Bilateral symmetry
and sexual selection: a meta-analysis. The American
Naturalist, 151(2):174–192.
Stanley, K. O., D’Ambrosio, D. B., and Gauci, J. (2009).
A hypercube-based encoding for evolving large-scale
neural networks. Artificial life, 15(2):185–212.
Toxvaerd, S. (2021). The emergence of the bilateral symme-
try in animals: A review and a new hypothesis. Sym-
metry, 13(2):261.
Weisstein, E. W. (2003). Fermat points. https://mathworld.
wolfram.com/FermatPoints.html.
APPENDIX
A Hyperparameter Selection
Analysis
(a) Fitness distributions for different values of
body mutation rates (p
brain
).
(b) Fitness distributions for different values of
brain mutation rates (p
brain
).
Figure 7: Comparing distributions of robots fitnesses of last
generations over 30 experiments, while controlling, with
grid search, for k and vision capability, given different val-
ues of mutation rates. n = µ ∗30.
While for the tournament size parameter k we
saw some clear improvement in convergence using
a higher value of 6 instead of 3, for the overall mu-
tation rates of the “brain” and “body” genotypes we
Testing Emergent Bilateral Symmetry in Evolvable Robots with Vision
105