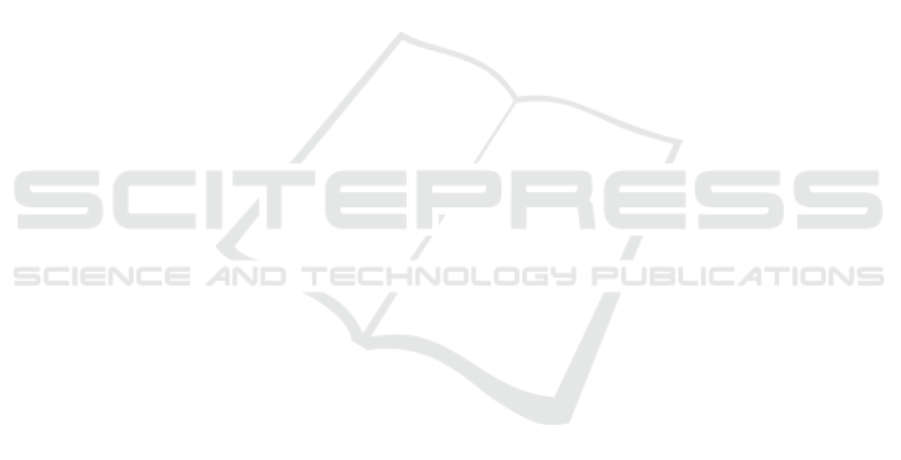
10, 2017, Revised Selected Papers 12, pages 81–95.
Springer.
De Vega, F. F., Alvarado, J., and Cortez, J. V. (2022). Opti-
cal music recognition and deep learning: An appli-
cation to 4-part harmony. In 2022 IEEE Congress
on Evolutionary Computation (CEC), pages 01–07.
IEEE.
Everingham, M. et al. (2010). The pascal visual ob-
ject classes (voc) challenge. International Journal of
Computer Vision.
Girshick, R. (2015). Fast r-cnn. In Proceedings of the IEEE
International Conference on Computer Vision.
Good, M. (2001). Musicxml: An internet-friendly format
for sheet music. In XML Conference and Expo.
Haji
ˇ
c, J. and Pecina, P. (2017). The muscima++ dataset for
handwritten optical music recognition. In 14th IAPR
ICDAR. IEEE.
He, K. et al. (2016). Deep residual learning for image recog-
nition. In Proceedings of the IEEE Conference on
Computer Vision and Pattern Recognition (CVPR).
He, K. et al. (2017). Mask r-cnn. In Proceedings of the
IEEE International Conference on Computer Vision.
Jiao, L., Zhang, F., Liu, F., Yang, S., Li, L., Feng, Z., and
Qu, R. (2019). A survey of deep learning-based object
detection. IEEE Access.
Johnson, J. M. and Khoshgoftaar, T. M. (2019). Survey on
deep learning with class imbalance. Journal of Big
Data.
Jr, J. H., Dorfer, M., Widmer, G., and Pecina, P. (2018).
Towards full-pipeline handwritten omr with musical
symbol detection by u-nets. In ISMIR.
Li, Y., Liu, H., Jin, Q., Cai, M., and Li, P. (2023). Tromr:
Transformer-based polyphonic optical music recogni-
tion. In ICASSP 2023-2023 IEEE International Con-
ference on Acoustics, Speech and Signal Processing
(ICASSP), pages 1–5. IEEE.
Lin, T.-Y. et al. (2014). Microsoft coco: Common objects in
context. In European Conference on Computer Vision.
Springer.
Long, J., Shelhamer, E., and Darrell, T. (2015). Fully con-
volutional networks for semantic segmentation. In
Proceedings of CVPR.
Pacha, A. (2019). Incremental supervised staff detection.
In Proceedings of the 2nd international workshop on
reading music systems, pages 16–20.
Pacha, A. and Calvo-Zaragoza, J. (2018). Optical music
recognition in mensural notation with region-based
convolutional neural networks. In ISMIR, pages 240–
247.
Pacha, A., Choi, K., Couasnon, B., Ricquebourg, Y.,
Zanibbi, R., and Eidenberger, H. (2018). Handwrit-
ten music object detection: Open issues and baseline
results. In 13th IAPR International Workshop on Doc-
ument Analysis Systems (DAS), pages 163–168. IEEE.
Pacha, A. and Eidenberger, H. (2017). Towards self-
learning optical music recognition. In 2017 16th IEEE
International Conference on Machine Learning and
Applications (ICMLA), pages 795–800. IEEE.
Rebelo, A., Fujinaga, I., Paszkiewicz, F., Marcal, A. R. S.,
Guedes, C., and Cardoso, J. S. (2012). Optical mu-
sic recognition: State-of-the-art and open issues. In-
ternational Journal of Music Information Retrieval
(IJMIR).
Ren, S., He, K., Girshick, R., and Sun, J. (2015). Faster
r-cnn: Towards real-time object detection with region
proposal networks. Advances in neural information
processing systems, 28.
R
´
ıos-Vila, A., Calvo-Zaragoza, J., and Paquet, T. (2024a).
Sheet music transformer: End-to-end optical music
recognition beyond monophonic transcription. arXiv
preprint arXiv:2402.07596.
R
´
ıos-Vila, A., Calvo-Zaragoza, J., Rizo, D., and Paquet, T.
(2024b). Sheet music transformer++: End-to-end full-
page optical music recognition for pianoform sheet
music. arXiv preprint arXiv:2405.12105.
Roland, P. (2002). The music encoding initiative (mei). In
Proceedings of the First International Conference on
Musical Applications Using XML.
Ronneberger, O., Fischer, P., and Brox, T. (2015). U-net:
Convolutional networks for biomedical image seg-
mentation. In MICCAI. Springer.
Shatri, E. and Fazekas, G. (2020). Optical music recogni-
tion: State of the art and major challenges. In Proceed-
ings of TENOR’20/21, Hamburg, Germany. Hamburg
University for Music and Theater.
Shatri, E. and Fazekas, G. (2021). Doremi: First glance
at a universal omr dataset. In Proceedings of the 3rd
WoRMS.
Szegedy, C. et al. (2017). Inception-v4, inception-resnet
and the impact of residual connections on learning.
In Proceedings of the AAAI Conference on Artificial
Intelligence (AAAI).
Tuggener, L., Elezi, I., Schmidhuber, J., and Stadelmann,
T. (2018). Deep watershed detector for music object
recognition. arXiv preprint arXiv:1805.10548.
Yesilkanat, A., Soullard, Y., Co
¨
uasnon, B., and Girard, N.
(2023). Full-page music symbols recognition: state-
of-the-art deep models comparison for handwritten
and printed music scores.
Zhao, Z. et al. (2019). Object detection with deep learning:
A review. IEEE Transactions on Neural Networks and
Learning Systems.
Knowledge Discovery in Optical Music Recognition: Enhancing Information Retrieval with Instance Segmentation
319