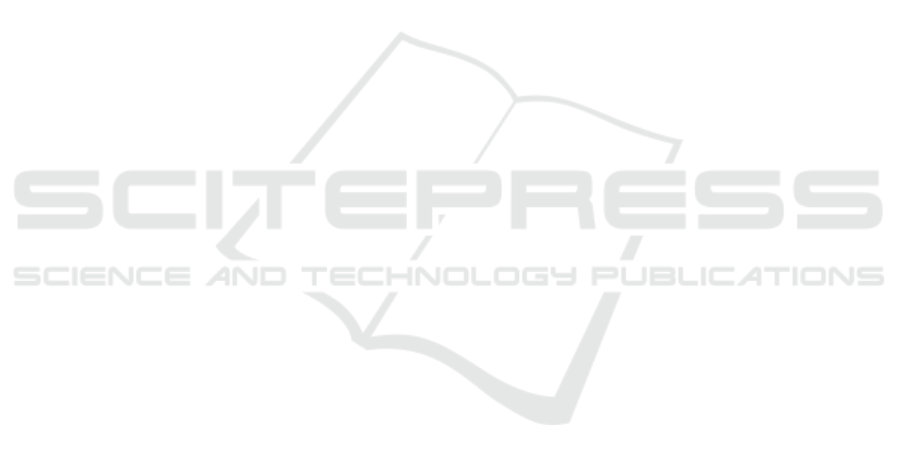
The comparison between both methods showed
a notable improvement in monitoring accuracy when
using sensors. In addition, a 10% decrease in illegal
logging was observed with the implementation of the
sensor system. This shows that we achieved greater
efficiency in detecting illegal logging. Early detection
and immediate notifications allowed for faster actions
to be taken to prevent and mitigate illegal logging, re-
sulting in an effective reduction in the number of trees
cut down.
6 CONCLUSIONS AND FUTURE
WORK
The illegal logging detection model allowed us to
identify illegal logging activities with a high accu-
racy of 90%, resulting in a 10% reduction in these
activities in real-time by detecting chainsaw sounds.
The experimental results indicated that the Convolu-
tional Neural Network (CNN) algorithm offers bet-
ter performance and higher effectiveness compared to
other methods. The research focused on identifying
chainsaw sounds during logging activities in the for-
est. The experimental validation was carried out using
a Huawei P SMART 2019 mobile device to record and
send the audios to the detection model. The model
was evaluated with different sounds at close distances
of 10 meters and far distances of 110 meters, ob-
taining positive and acceptable results, with an illegal
logging detection accuracy ranging between 80% and
90%.
As future work, it is recommended to implement
this model in other municipalities in the Amazonas
region, such as Chachapoyas, Utcubamba, and Con-
dorcanqui, to expand the use of the application and
strengthen forest protection. In addition, the integra-
tion of more sophisticated smart sensors, such as the
AR854, is suggested to cover a larger area and achieve
a more precise analysis of results. Collaboration with
local governments and environmental organizations
will be crucial for the success and sustainability of
these initiatives. In particular, efforts should be fo-
cused on critical points such as Chachapoyas where
illegal logging is most prevalent, to maximize the im-
pact of protection measures.
ACKNOWLEDGEMENTS
We would like to express our gratitude to the Re-
search Department of the Peruvian University of Ap-
plied Sciences for all the support provided throughout
the research and development process of this work.
REFERENCES
Al-Obaidi, A. P. D. A. S. M. (2017). Edu.my. Available:
http://jestec.taylors.edu.my/V12Issue2.htm.
Antonelli, A., Dhanjal-Adams, K. L., and Silvestro, D.
(2023). Integrating machine learning, remote sensing
and citizen science to create an early warning system
for biodiversity. Plants, People, Planet, 5:307–316.
Ball, J. G. C., Petrova, K., Coomes, D. A., and Flaxman, S.
(2022). Using deep convolutional neural networks to
forecast spatial patterns of amazonian deforestation.
Methods in Ecology and Evolution, 13:2622–2634.
Bogomolov, A. R. (2021). Monitoring and diagnostics
of forest condition using sound sensors and artificial
intelligence technologies. IOP Conference Series,
678(1):012005.
Casallas, A. (2022). Design of a forest fire early alert system
through a deep 3d-cnn. Available: https://www.mdpi
.com/1424-8220/22/22/8790.
D. H., . e. P. P. d. U. M. d. J. (2022). Prevenir amazon
´
ıa.
Available:https://preveniramazonia.pe/nuevo-estudio
-tala-ilegal-amazonia-peruana/ [Accessed: 12-Apr-
2024].
Doblas, J., Shimabukuro, Y., Sant’Anna, S., Carneiro, A.,
Arag
˜
ao, L., and Almeida, C. (2020). Optimizing
near real-time detection of deforestation on tropical
rainforests using sentinel-1 data. Remote Sensing,
12:3922.
Dominguez, D., Villar, L. P. D., Pantoja, O., and Gonz
´
alez,
M. (2022). Forecasting amazon rain-forest deforesta-
tion using a hybrid machine learning model. Sustain-
ability, 14(2):691.
Dong, Q., Liu, Y., and Liu, X. (2023). Drone sound de-
tection system based on feature result-level fusion us-
ing deep learning. Multimedia Tools and Applications,
82:149–171.
GEOBOSQUE (2017). P
´
erdida de bosque. Available: https:
//geobosques.minam.gob.pe/geobosque/view/acerca.
php.
Georreferenciada, R. A. D. I. S. (2018). La amazon
´
ıa saque-
ada. Available: https://mineriailegal.amazoniasocioa
mbiental.org/ [Accessed: 12-Apr-2024].
Hethcoat, M. G., Carreiras, J. M. B., Edwards, D. P., Bryant,
R. G., and Quegan, S. (2021). Detecting tropical se-
lective logging with c-band sar data may require a
time series approach. Remote Sensing of Environment,
259:112411.
Kim, J., Min, K., Jung, M., and Chi, S. (2020). Occu-
pant behavior monitoring and emergency event detec-
tion in single-person households using deep learning-
based sound recognition. Building and Environment,
181:107092.
Kitchenham, S. M. C. B. (2007). Guidelines for performing
systematic literature reviews in software engineering.
https://www.researchgate.net/publication/302924724
WEBIST 2024 - 20th International Conference on Web Information Systems and Technologies
248