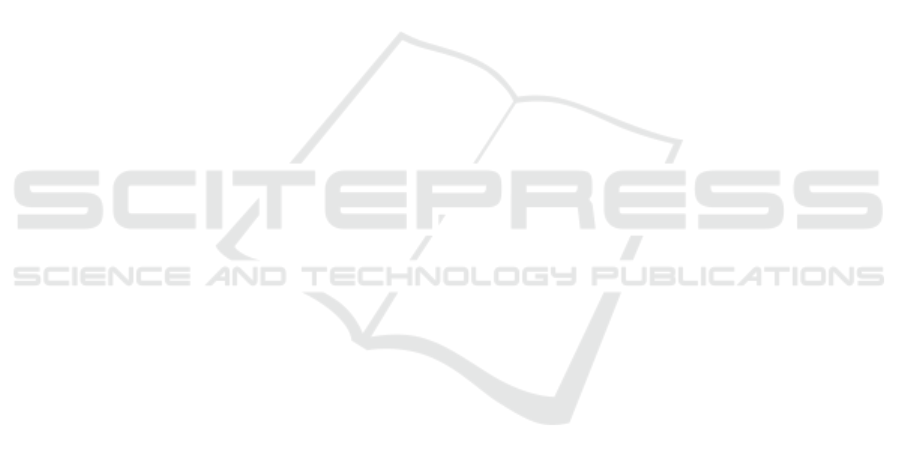
the predictive accuracy of these variables.
Experimental results revealed differences in the
performance of predictive models when comparing
georeferenced and non-georeferenced data. Particu-
larly, prediction models that use geographic features
outperformed prediction models that do not use them,
with gains ranging from 9.6% to 19.6% in the stan-
dard deviation of MAPE, from 5.5% to 10.4% in
MAE, and from 0.99% to 2.9% in RMSE. These re-
sults highlight the importance of considering the spa-
tial context when building predictive models, as incor-
porating geographic variables can lead to significant
improvements in prediction accuracy.
For future research, one intent to investigate mul-
tivariate spatial analyses and explore more sophisti-
cated spatial models to optimize employee turnover
prediction. Advanced models such as Spatial Au-
toregressive Models (SAR) and Spatial Error Mod-
els (SEM) offer more refined perspectives on spatial
interactions that may impact employee tenure. Addi-
tionally, the use of Spatial Neural Networks and Hi-
erarchical Bayesian Models can provide deeper and
more precise insights by capturing complexities in
spatial dependencies and data heterogeneity.
ACKNOWLEDGEMENTS
The authors thank the Pontif
´
ıcia Universidade
Cat
´
olica de Minas Gerais – PUC-Minas and
Coordenac¸
˜
ao de Aperfeic¸oamento de Pessoal de
N
´
ıvel Superior — CAPES (CAPES – Grant PROAP
88887.842889/2023-00 – PUC/MG, Grant PDPG
88887.708960/2022-00 – PUC/MG - Inform
´
atica,
and Finance Code 001).
REFERENCES
Ajjali, W. (2023). Coordinate systems and projections.
In ArcGIS Pro e ArcGIS Online, Springer Text-
books in Earth Sciences, Geography and Environ-
ment. Springer, Cham.
Anselin, L. (2021). Spatial models in econometric research.
In Oxford Research Encyclopedia of Economics and
Finance.
Anselin, L. and Bera, A. (1998). Spatial dependence in lin-
ear regression models with an introduction to spatial
econometrics. In Ullah, A. and Giles, D. E., editors,
Handbook of Applied Economic Statistics, pages 237–
289. Marcel Dekker.
Chen, Y. (2013). New approaches for calculating
moran’s index of spatial autocorrelation. PloS one,
8(7):e68336.
Chen, Y. (2023). Spatial autocorrelation equation based on
moran’s index. Scientific Reports, 13(1):19296.
Dramsch, J. S. (2020). 70 years of machine learning in geo-
science in review. Advances in Geophysics, 61:1–55.
Feitosa, F., Barros, J., Marques, E., and Giannotti, M.
(2021). Measuring changes in residential segregation
in s
˜
ao paulo in the 2000s. In Urban Socio-Economic
Segregation and Income Inequality, pages 507–523.
Springer International Publishing.
Griffith, D. A. (2000). A linear regression solution to the
spatial autocorrelation problem. Journal of Geograph-
ical Systems, 2:141–156.
Lazzari, M., Alvarez, J. M., and Ruggieri, S. (2022). Pre-
dicting and explaining employee turnover intention.
International Journal of Data Science and Analytics,
14(3):279–292.
Luo, Y. and Tang, Z. (2024). The impact of open gov-
ernment data on the digital economy: Evidence from
china. SSRN.
Nikiforova, A. and McBride, K. (2021). Open government
data portal usability: A user-centred usability analysis
of 41 open government data portals. Telematics and
Informatics, 58:101539.
Oshan, T., Wolf, L., Sachdeva, M., et al. (2022). A scoping
review on the multiplicity of scale in spatial analysis.
Journal of Geographical Systems, 24:293–324.
Pebesma, E. and Bivand, R. (2023). Spatial data science:
With applications in R. Chapman and Hall/CRC.
Rey, S. J., Anselin, L., and Li, W. (2021). Handbook of
Spatial Analysis in the Social Sciences. Edward Elgar
Publishing.
Son, W., Hwang, S. W., and Ahn, H. K. (2014). Mssq:
Manhattan spatial skyline queries. Information Sys-
tems, 40:67–83.
Taleb, N. N. (2010). The Black Swan: The Impact of the
Highly Improbable. Random House, 2nd edition.
Thibault Landry, A., Schweyer, A., and Whillans, A.
(2017). Winning the war for talent: Modern motiva-
tional methods for attracting and retaining employees.
Compensation & Benefits Review, 49(4):230–246.
Wang, J.-F., Zhang, T.-L., and Fu, B.-J. (2016). A measure
of spatial stratified heterogeneity. Ecological Indica-
tors, 67:250–256.
Wirtz, B. W., Weyerer, J. C., Becker, M., and M
¨
uller, W. M.
(2022). Open government data: A systematic litera-
ture review of empirical research. Electronic Markets,
32(4):2381–2404.
Zhao, Y., Hryniewicki, M. K., Cheng, F., Fu, B., and Zhu,
X. (2019). Employee turnover prediction with ma-
chine learning: A reliable approach. In Sani, A.
M. M., Shaout, K., and Abbass, H. A., editors, Intel-
ligent Systems and Applications: Proceedings of the
2018 Intelligent Systems Conference (IntelliSys) Vol-
ume 2, pages 737–758. Springer International Publish-
ing.
Exploiting Data Spatial Dependencies for Employee Turnover Prediction
257