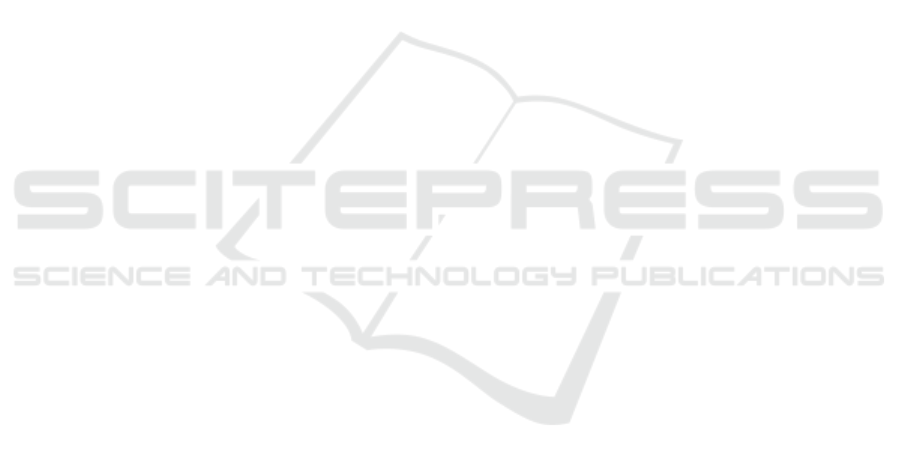
graph and text. Proceedings of the AAAI Conference
on Artificial Intelligence, 32(1).
Han, Z. and Wang, J. (2024). Knowledge enhanced graph
inference network based entity-relation extraction and
knowledge graph construction for industrial domain.
Frontiers of Engineering Management, 11(1):143–
158.
Hao, S., Tan, B., Tang, K., Ni, B., Shao, X., Zhang, H.,
Xing, E. P., and Hu, Z. (2023). Bertnet: Harvesting
knowledge graphs with arbitrary relations from pre-
trained language models. In Rogers, A., Boyd-Graber,
J. L., and Okazaki, N., editors, Findings of the As-
sociation for Computational Linguistics: ACL 2023,
Toronto, Canada, July 9-14, 2023, pages 5000–5015.
Association for Computational Linguistics.
Jiang, J., Zhou, K., Zhao, W. X., Li, Y., and Wen, J.-R.
(2023). Reasoninglm: Enabling structural subgraph
reasoning in pre-trained language models for ques-
tion answering over knowledge graph. arXiv preprint
arXiv:2401.00158.
Kommineni, V. K., K
¨
onig-Ries, B., and Samuel, S. (2024).
From human experts to machines: An llm supported
approach to ontology and knowledge graph construc-
tion. arXiv preprint arXiv:2403.08345.
Lin, D. (2024). Revolutionizing retrieval-augmented gener-
ation with enhanced pdf structure recognition. arXiv
preprint arXiv:2401.12599.
Lison, P., Barnes, J., and Hubin, A. (2021). skweak: Weak
supervision made easy for NLP. In Ji, H., Park, J. C.,
and Xia, R., editors, Proceedings of the 59th Annual
Meeting of the Association for Computational Lin-
guistics and the 11th International Joint Conference
on Natural Language Processing: System Demonstra-
tions, pages 337–346, Online. Association for Com-
putational Linguistics.
Liu, J. and Duan, L. (2021). A survey on knowledge graph-
based recommender systems. In 2021 IEEE 5th Ad-
vanced Information Technology, Electronic and Au-
tomation Control Conference (IAEAC), pages 2450–
2453.
Luo, L., Li, Y.-F., Haffari, G., and Pan, S. (2023).
Reasoning on graphs: Faithful and interpretable
large language model reasoning. arXiv preprint
arXiv:2310.01061.
Markowitz, E., Balasubramanian, K., Mirtaheri, M., An-
navaram, M., Galstyan, A., and Ver Steeg, G. (2022).
StATIK: Structure and text for inductive knowledge
graph completion. In Carpuat, M., de Marneffe, M.-
C., and Meza Ruiz, I. V., editors, Findings of the Asso-
ciation for Computational Linguistics: NAACL 2022,
pages 604–615, Seattle, United States. Association for
Computational Linguistics.
Melnyk, I., Dognin, P., and Das, P. (2022). Knowledge
graph generation from text. In Findings of the Asso-
ciation for Computational Linguistics: EMNLP 2022,
pages 1610–1622, Abu Dhabi, United Arab Emirates.
Association for Computational Linguistics.
Nguyen, H. L., Vu, D. T., and Jung, J. J. (2020). Knowledge
graph fusion for smart systems: A survey. Information
Fusion, 61:56–70.
Noy, N., Gao, Y., Jain, A., Narayanan, A., Patterson, A., and
Taylor, J. (2019). Industry-scale knowledge graphs:
lessons and challenges. Commun. ACM, 62(8):36–43.
OpenAI (2024). Hello gpt-4o. Online:
https://openai.com/index/hello-gpt-4o (accessed
2024-06-24).
Pan, S., Luo, L., Wang, Y., Chen, C., Wang, J., and Wu, X.
(2024). Unifying large language models and knowl-
edge graphs: A roadmap. IEEE Transactions on
Knowledge and Data Engineering.
Purohit, H., Shalin, V. L., and Sheth, A. P. (2020). Knowl-
edge graphs to empower humanity-inspired ai sys-
tems. IEEE Internet Computing, 24(4):48–54.
Schramm, S., Wehner, C., and Schmid, U. (2023). Compre-
hensible artificial intelligence on knowledge graphs:
A survey. Journal of Web Semantics, 79:100806.
Shen, T., Zhang, F., and Cheng, J. (2022). A compre-
hensive overview of knowledge graph completion.
Knowledge-Based Systems, 255:109597.
Sun, Q., Luo, Y., Zhang, W., Li, S., Li, J., Niu, K., Kong,
X., and Liu, W. (2024). Docs2kg: Unified knowledge
graph construction from heterogeneous documents as-
sisted by large language models. arXiv preprint
arXiv:2406.02962.
Tama
ˇ
sauskaite, G. and Groth, P. (2023). Defining a knowl-
edge graph development process through a systematic
review. ACM Trans. Softw. Eng. Methodol., 32(1).
Toro, S., Anagnostopoulos, A. V., Bello, S., Blumberg,
K., Cameron, R., Carmody, L., Diehl, A. D., Doo-
ley, D., Duncan, W., Fey, P., et al. (2023). Dy-
namic retrieval augmented generation of ontologies
using artificial intelligence (dragon-ai). arXiv preprint
arXiv:2312.10904.
Veseli, B., Singhania, S., Razniewski, S., and Weikum, G.
(2023). Evaluating language models for knowledge
base completion. In European Semantic Web Confer-
ence, pages 227–243. Springer.
Wang, Y., Wan, Y., Bai, L., Cui, L., Xu, Z., Li, M., Yu, P. S.,
and Hancock, E. R. (2024). Collaborative knowledge
graph fusion by exploiting the open corpus. IEEE
Transactions on Knowledge and Data Engineering,
36(2):475–489.
Zhang, X., Bosselut, A., Yasunaga, M., Ren, H., Liang, P.,
Manning, C. D., and Leskovec, J. (2022). Greaselm:
Graph reasoning enhanced language models for ques-
tion answering. arXiv preprint arXiv:2201.08860.
Zhang, Y., Chen, Z., Zhang, W., and Chen, H.
(2023). Making large language models perform bet-
ter in knowledge graph completion. arXiv preprint
arXiv:2310.06671.
Zhou, Z.-W., Ting, Y.-H., Jong, W.-R., Chen, S.-C., and
Chiu, M.-C. (2023). Development and application of
knowledge graphs for the injection molding process.
Machines, 11(2):271.
Zhu, Y., Wang, X., Chen, J., Qiao, S., Ou, Y., Yao, Y.,
Deng, S., Chen, H., and Zhang, N. (2023). Llms for
knowledge graph construction and reasoning: Recent
capabilities and future opportunities. arXiv preprint
arXiv:2305.13168.
ICINCO 2024 - 21st International Conference on Informatics in Control, Automation and Robotics
376