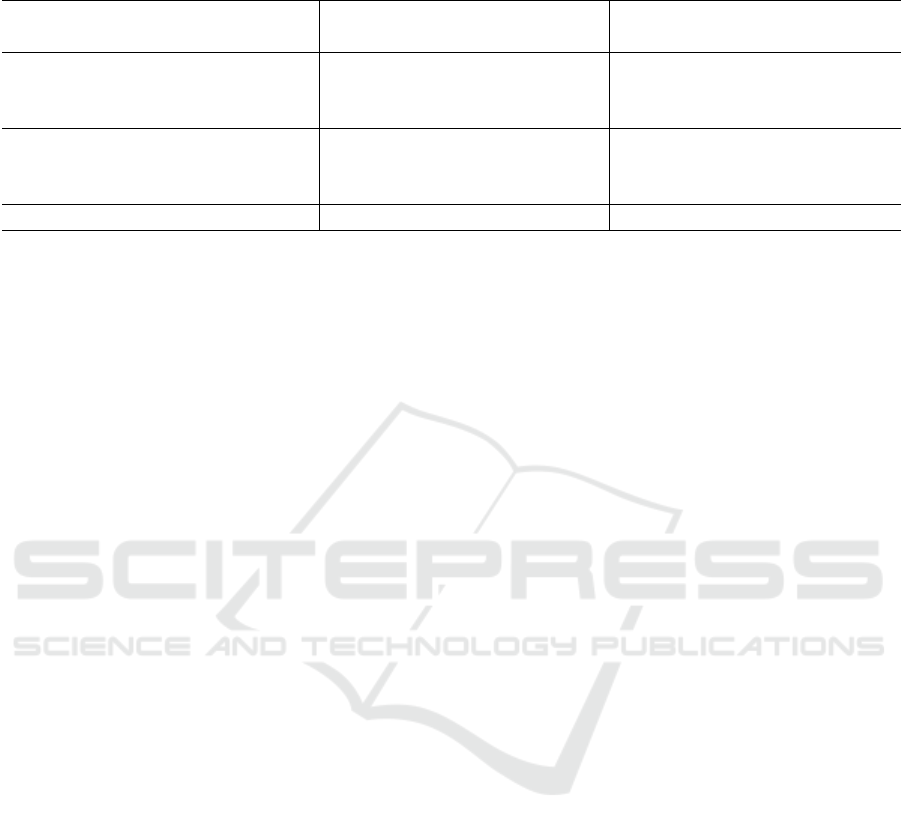
Table 3: Comparison of baselines with experimental settings. Our proposed prompt-based knowledge distillation models
outperform the baseline models.
Type Model
WASSA-21 RWW
Precision Recall F1 Precision Recall F1
Baseline
BERT 68.52 68.67 67.70 18.81 19.33 18.80
RoBERTa 72.39 73.84 71.74 23.20 21.97 20.61
XLNet 60.74 63.18 60.92 20.40 20.26 18.52
Experiment
BERT+PKD 69.02 71.15 68.58 23.52 23.32 21.17
RoBERTa+PKD 73.85 75.16 73.92 24.63 22.69 22.47
XLNet+PKD 62.55 64.29 61.75 22.52 21.41 19.28
∆ Change +1.46 +1.32 +2.18 +1.43 +0.72 +1.86
tions. In 2012 IEEE/WIC/ACM International Confer-
ences on Web Intelligence and Intelligent Agent Tech-
nology, volume 1, pages 346–353.
Alhuzali, H. and Ananiadou, S. (2021). SpanEmo: Casting
multi-label emotion classification as span-prediction.
In Merlo, P., Tiedemann, J., and Tsarfaty, R., edi-
tors, Proceedings of the 16th Conference of the Eu-
ropean Chapter of the Association for Computational
Linguistics: Main Volume, pages 1573–1584, Online.
Association for Computational Linguistics.
Baccianella, S., Esuli, A., and Sebastiani, F. (2010). Senti-
WordNet 3.0: An enhanced lexical resource for senti-
ment analysis and opinion mining. In Calzolari, N.,
Choukri, K., Maegaard, B., Mariani, J., Odijk, J.,
Piperidis, S., Rosner, M., and Tapias, D., editors, Pro-
ceedings of the Seventh International Conference on
Language Resources and Evaluation (LREC’10), Val-
letta, Malta. European Language Resources Associa-
tion (ELRA).
Brown, T. B., Mann, B., Ryder, N., Subbiah, M., Kaplan, J.,
Dhariwal, P., Neelakantan, A., Shyam, P., Sastry, G.,
Askell, A., Agarwal, S., Herbert-Voss, A., Krueger,
G., Henighan, T., Child, R., Ramesh, A., Ziegler,
D. M., Wu, J., Winter, C., Hesse, C., Chen, M., Sigler,
E., Litwin, M., Gray, S., Chess, B., Clark, J., Berner,
C., McCandlish, S., Radford, A., Sutskever, I., and
Amodei, D. (2020). Language models are few-shot
learners.
Buechel, S., Buffone, A., Slaff, B., Ungar, L., and Sedoc, J.
(2018). Modeling empathy and distress in reaction to
news stories. In Proceedings of the 2018 Conference
on Empirical Methods in Natural Language Process-
ing. Association for Computational Linguistics.
Buechel, S., Modersohn, L., and Hahn, U. (2020). Towards
label-agnostic emotion embeddings.
Calefato, F., Lanubile, F., and Novielli, N. (2018). Emotxt:
A toolkit for emotion recognition from text.
Demszky, D., Movshovitz-Attias, D., Ko, J., Cowen, A.,
Nemade, G., and Ravi, S. (2020). Goemotions: A
dataset of fine-grained emotions. In Proceedings of
the 58th Annual Meeting of the Association for Com-
putational Linguistics. Association for Computational
Linguistics.
Devlin, J., Chang, M.-W., Lee, K., and Toutanova, K.
(2019). Bert: Pre-training of deep bidirectional trans-
formers for language understanding. In Proceedings
of the 2019 Conference of the North. Association for
Computational Linguistics.
Ekman, P. and Friesen, W. V. (1971). Constants across cul-
tures in the face and emotion. Journal of personality
and social psychology, 17(2):124.
Farquhar, S., Kossen, J., Kuhn, L., and Gal, Y. (2024). De-
tecting hallucinations in large language models using
semantic entropy. Nature, 630(8017):625–630.
Halder, K., Akbik, A., Krapac, J., and Vollgraf, R. (2020).
Task-aware representation of sentences for generic
text classification. In Scott, D., Bel, N., and Zong, C.,
editors, Proceedings of the 28th International Confer-
ence on Computational Linguistics, pages 3202–3213,
Barcelona, Spain (Online). International Committee
on Computational Linguistics.
Hasan, M., Rundensteiner, E., and Agu, E. (2019). Auto-
matic emotion detection in text streams by analyzing
twitter data. International Journal of Data Science
and Analytics, 7.
Hinton, G., Vinyals, O., and Dean, J. (2015). Distilling
the knowledge in a neural network. In NIPS Deep
Learning and Representation Learning Workshop.
Jiang, Y., Chan, C., Chen, M., and Wang, W. (2023). Lion:
Adversarial distillation of proprietary large language
models. In The 2023 Conference on Empirical Meth-
ods in Natural Language Processing.
Kleinberg, B., van der Vegt, I., and Mozes, M. (2020).
Measuring emotions in the covid-19 real world worry
dataset.
Liu, Y., Ott, M., Goyal, N., Du, J., Joshi, M., Chen, D.,
Levy, O., Lewis, M., Zettlemoyer, L., and Stoyanov,
V. (2019). Roberta: A robustly optimized bert pre-
training approach.
Loshchilov, I. and Hutter, F. (2017). Decoupled weight
decay regularization. In International Conference on
Learning Representations.
Lukasik, M., Bhojanapalli, S., Menon, A. K., and Kumar, S.
(2022). Teacher’s pet: understanding and mitigating
biases in distillation. Transactions on Machine Learn-
ing Research.
Prompt Distillation for Emotion Analysis
333