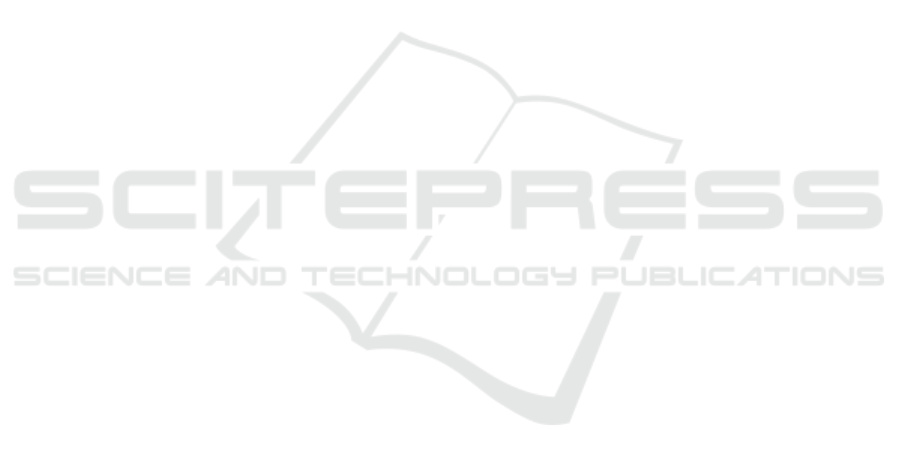
EASA (2021b). Concepts of Design Assurance for Neural
Networks (CoDANN) II. EASA and Daedalean.
EASA (2023a). Artificial Intelligence Roadmap 2.0 –
Human-centric approach to AI in aviation. European
Aviation Safety Agency (EASA), Cologne.
EASA (2023b). Concept Paper: First Usable Guidance for
Level 1&2 Machine Learning Applications, n.2. Eu-
ropean Aviation Safety Agency (EASA), Cologne.
EUROCAE (2005). DO-254 / ED-80 Design Assurance
Guidance for Airborne Electronic Hardware. RTCA,
Inc / EUROCAE.
EUROCAE (2011). DO-178 / ED-12C – Software Consid-
erations in Airborne Systems and Equipment Certifi-
cation. RTCA, Inc / EUROCAE.
Gabreau, C., Gauffriau, A., Grancey, F. D., Ginestet, J.-
B., and Pagetti, C. (2022). Toward the certification
of safety-related systems using ML techniques: the
ACAS-Xu experience. In 11th European Congress on
Embedded Real Time Software and Systems, ERTS.
Gabreau, C., Pesquet-Popescu, B., Kaakai, F., and Lefevre,
B. (2021). AI for Future Skies: On-going standardis-
ation activities to build the next certification/approval
framework for airborne and ground aeronautical prod-
ucts. In proceedings of the Workshop on Artificial In-
telligence Safety (AISafety).
Gauffriau, A. and Pagetti, C. (2023). Formal description of
ml models for unambiguous implementation.
Grancey, F. D., Ducoffe, M., Gabreau, C., Gauffriau, A.,
Ginestet, J.-B., Hervieu, A., Huraux, T., Pagetti, C.,
Clavi
`
ere, A., and Damour, M. (2022). Optimizing the
design of a safe ML-based system - the ACAS Xu ex-
perience. In 11th European Congress on Embedded
Real Time Software and Systems, ERTS.
Hawkins, R., Habli, I., Kelly, T., and McDermid, J. (2013).
Assurance cases and prescriptive software safety cer-
tification: a comparative study. Safety Science, 59:55–
71.
Hawkins, R. and Kelly, T. (2009). A systematic approach
for developing software safety arguments. In Proceed-
ings of the 27th International Systems Safety Confer-
ence.
Hawkins, R. and Kelly, T. (2010). A structured approach to
selecting and justifying software safety evidence. In
Proceedings of the 5th IET System Safety Conference.
Hawkins, R., Paterson, C., Picardi, C., Jia, Y., Calinescu,
R., and Habli, I. (2021). Guidance on the Assurance
of Machine Learning in Autonomous Systems (AM-
LAS).
He, X., Zhao, K., and Chu, X. (2021). Automl: A sur-
vey of the state-of-the-art. Knowledge-Based Systems,
212:106622.
Idmessaoud, Y., Farges, J.-L., Jenn, E., Mussot, V., Fernan-
des Pires, A., Chenevier, F., and Conejo Laguna, R.
(2024). Uncertainty in Assurance Case Template for
Machine Learning. In Embedded Real Time Systems
(ERTS), Toulouse, France.
Kaakai, F., Adibhatla, S. S., Pai, G., and Escorihuela, E.
(2023). Data-centric operational design domain char-
acterization for machine learning-based aeronautical
products. In Guiochet, J., Tonetta, S., and Bitsch,
F., editors, Computer Safety, Reliability, and Security,
pages 227–242, Cham. Springer Nature Switzerland.
Kaakai, F., Dmitriev, K., Adibhatla, S., Baskaya, E.,
Bezzecchi, E., Bharadwaj, R., Brown, B., Gentile,
G., Gingins, C., Grihon, S., and Travers, C. (2022).
Toward a machine learning development lifecycle for
product certification and approval in aviation. SAE In-
ternational Journal of Aerospace, 15(2):127–143.
Kelly, T. and Weaver, R. (2004). The goal structuring nota-
tion – a safety argument notation. In Proceedings of
the dependable systems and networks 2004 workshop
on assurance cases, volume 6. Citeseer Princeton, NJ.
LNE (2021). Certification Standard of Processes for AI.
Technical report, Laboratoire National de m
´
etrologie
et d’essais (LNE).
MLEAP (2024). EASA Research -– Machine Learning Ap-
plication Approval (MLEAP) – final report. European
Union Aviation Safety Agency (EASA).
Polacsek, T., Sharma, S., Cuiller, C., and Tuloup, V. (2018).
The need of diagrams based on toulmin schema appli-
cation: an aeronautical case study. EURO Journal on
Decision Processes, 6(3):257–282.
SAE (2011). ARP4754A / ED-79A – Guidelines for de-
velopment of civil aircraft and systems-enhancements,
novelties and key topics. SAE / EUROCAE.
SAE (2021). EUROCAE WG114 / SAE G34 Artificial In-
telligence in Aviation – AIR6988 / ER-022 – Artifi-
cial Intelligence in Aeronautical Systems: Statement
of Concerns. SAE / EUROCAE.
SAE (2025). ARP6983 / ED-324 – Process Standard for
Development and Certification/Approval of Aeronau-
tical Safety-Related Products Implementing AI (to ap-
pear). SAE / EUROCAE.
Thinking the Certification Process of Embedded ML-Based Aeronautical Components Using AIDGE, a French Open and Sovereign AI
Platform
71