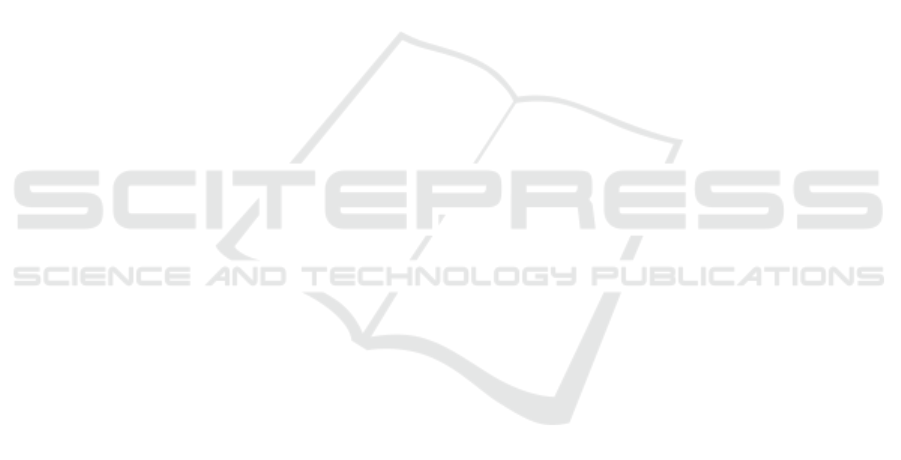
strengthen the proposed clustering approach.
Future work will focus on evaluating the approach
on larger datasets while removing the assumption of
equal probability-distributions among clients.
ACKNOWLEDGEMENTS
This paper was developed within the project funded
by Next Generation EU - “Age-It - Ageing well in
an ageing society” project (PE0000015), National Re-
covery and Resilience Plan (NRRP) - PE8 - Mission
4, C2, Intervention 1.3. The views and opinions ex-
pressed are only of the authors and do not necessarily
reflect those of the European Union or the European
Commission.
REFERENCES
Abid Ali Awan (2021). Heart Disease patients -
Targeting treatment for heart disease patients.
URL: https://www.kaggle.com/datasets/kingabzpro/
heart-disease-patients.
Act, A. (1996). Health insurance portability and account-
ability act of 1996. Public law, 104:191.
Battineni, G., Chintalapudi, N., and Amenta, F. (2019).
Data for: Machine learning in medicine: performance
calculation of dementia prediction by support vector
machines (SVM). Mendeley Data.
Bharati, S., Mondal, M. R. H., Podder, P., and Prasath, V. S.
(2022). Federated learning: Applications, challenges
and future directions.
Bonawitz, K., Kairouz, P., McMahan, B., and Ramage,
D. R. (2021). Federated learning and privacy. ACM
Queue, 19.
Brand
˜
ao, A., Mendes, R., and Vilela, J. P. (2021). Ef-
ficient Privacy Preserving Distributed K-Means for
Non-IID Data. In Abreu, P. E., Pereira Rodrigues, P.,
Fern
´
andez, A., and Gama, J., editors, Advances in In-
telligent Data Analysis XIX, 19th International Sym-
posium on Intelligent Data Analysis, IDA 2021, vol-
ume 12695, pages 439–451. Springer.
Dhade, P. and Shirke, P. (2024). Federated learning for
healthcare: A comprehensive review. Engineering
Proceedings, 59(1):230.
Garst, S. and Reinders, M. (2024). Federated K-Means
Clustering. ArXiV preprint, arXiv:2310.01195v2.
Liu, Y., Ma, Z., Yan, Z., Wang, Z., Liu, X., and a, J. (2020).
Privacy-preserving federated k-means for proactive
caching in next generation cellular networks. Infor-
mation Sciences, 521:14–31.
Marulli, F., Balzanella, A., Campanile, L., Iacono, M., and
Mastroianni, M. (2021a). Exploring a federated learn-
ing approach to enhance authorship attribution of mis-
leading information from heterogeneous sources. In
2021 International Joint Conference on Neural Net-
works (IJCNN), pages 1–8.
Marulli, F., Verde, L., Marrone, S., Barone, R., and De Bi-
ase, M. S. (2021b). Evaluating efficiency and effec-
tiveness of federated learning approaches in knowl-
edge extraction tasks. In 2021 International Joint
Conference on Neural Networks (IJCNN), pages 1–6.
Palechor, F. M. and De la Hoz Manotas, A. (2019). Dataset
for estimation of obesity levels based on eating habits
and physical condition in individuals from colombia,
peru and mexico. Data in brief, 25:104344.
Pedrycz, W. (2022). Federated FCM: Clustering Under Pri-
vacy Requirements. IEEE Transactions on Fuzzy Sys-
tems, 30(8):3384–3388.
Sheller, M. J., Edwards, B., Reina, G. A., Martin, J., Pati,
S., Kotrotsou, A., Milchenko, M., Xu, W., Marcus,
D., Colen, R. R., et al. (2020). Federated learning
in medicine: facilitating multi-institutional collabora-
tions without sharing patient data. Scientific reports,
10(1):12598.
Stallmann, M. and Wilbik, A. (2022). On a Framework
for Federated Cluster Analysis. Applied Sciences,
12(10455).
Voigt, P. and Von dem Bussche, A. (2017). The eu gen-
eral data protection regulation (gdpr). A Practical
Guide, 1st Ed., Cham: Springer International Pub-
lishing, 10(3152676):10–5555.
W. H. Wolberg, W. N. S. and Mangasarian, O. L.
(2017). Breast cancer Wisconsin (diagnos-
tic) data set. URL: https://www.kaggle.com/
datasets/nancyalaswad90/breast-cancer-dataset.
Wahab, O. A., Mourad, A., Otrok, H., and Taleb, T. (2021).
Federated machine learning: Survey, multi-level clas-
sification, desirable criteria and future directions in
communication and networking systems. IEEE Com-
munications Surveys Tutorials, 23(2):1342–1397.
Yang, K., Mohammadi Amiri, M., and Kulkarni, S. R.
(2024). Greedy centroid initialization for federated K
-means. Knowledge and Information Systems.
Zhu, S., Xu, Q., Zeng, J., Wang, S., Sun, Y., Yang, Z., and
Oeng, Z. (2023). F
3
KM: Federated, Fair, and Fast k-
means. Proc. ACM Manag. Data, 1(4):241.
A Federated K-Means-Based Approach in eHealth Domains with Heterogeneous Data Distributions
559