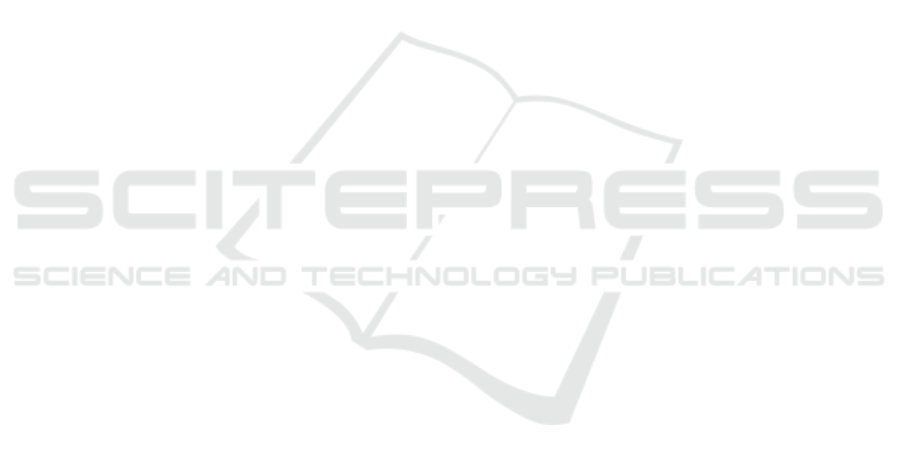
4.2 Conflicts of Interest
The authors declare that they have no known com-
peting financial interests or personal relationships that
could have appeared to influence the work reported in
this paper. Experiment participants signed an autho-
rization form prior to the study to authorize the usage
of the captured physiological data as well as perfor-
mance from their Riot Gamertag and remained anony-
mous according to the Spanish national law LOPD
(Ley Org
´
anica de Protecci
´
on de Datos de Car
´
acter
Personal).
REFERENCES
Ahsan, M. R., Ibrahimy, M. I., and Khalifa, O. O. (2009).
Emg signal classification for human computer interac-
tion: a review. volume 33, pages 480–501.
Alhargan, A., Cooke, N., and Binjammaz, T. (2017). Multi-
modal affect recognition in an interactive gaming en-
vironment using eye tracking and speech signals. In
Proceedings of the 19th ACM International Confer-
ence on Multimodal Interaction. ACM.
Argasi
´
nski, J. K. and Grabska-Gradzi
´
nska, I. (2017). Pat-
terns in serious game design and evaluation applica-
tion of eye-tracker and biosensors. In Artificial Intel-
ligence and Soft Computing, pages 367–377. Springer
International Publishing.
Benedek, M. and Kaernbach, C. (2010). A continuous mea-
sure of phasic electrodermal activity. Journal of neu-
roscience methods, 190(1):80–91.
Bradley, M. M. and Lang, P. J. (1994). Measuring emotion:
The self-assessment manikin and the semantic differ-
ential. Journal of Behavior Therapy and Experimental
Psychiatry, 25(1):49–59.
Bradley, M. M. and Lang, P. J. (2000). Measuring emo-
tion: Behavior, feeling, and physiology. In Lane, R.
D. R., Nadel, L., Ahern, G. L., Allen, J., and Kasz-
niak, A. W., editors, Cognitive Neuroscience of Emo-
tion, pages 25–49. Oxford University Press.
Cacioppo, J. T., Chen, H. Y., and Cacioppo, S. (2017).
Reciprocal influences between loneliness and self-
centeredness: A cross-lagged panel analysis in a
population-based sample of african american, his-
panic, and caucasian adults. Personality and Social
Psychology Bulletin, 43(8):1125–1135.
Ekman, P. (2005). Basic emotions. In Handbook of Cogni-
tion and Emotion, pages 45–60. John Wiley & Sons,
Ltd.
Hafeez, T., Saeed, S. M. U., Arsalan, A., Anwar, S. M.,
Ashraf, M. U., and Alsubhi, K. (2021). EEG in game
user analysis: A framework for expertise classification
during gameplay. PLOS ONE, 16(6):e0246913.
Jones, B. J., Tan, T., and Bloom, S. R. (2012). Minire-
view: Glucagon in stress and energy homeostasis. En-
docrinology, 153(3):1049–1054.
Kivikangas, J. M., Chanel, G., Cowley, B., Ekman, I.,
Salminen, M., J
¨
arvel
¨
a, S., and Ravaja, N. (2011). A
review of the use of psychophysiological methods in
game research. Journal of Gaming & Virtual Worlds,
3(3):181–199.
Leis, O. and Lautenbach, F. (2020). Psychological and
physiological stress in non-competitive and competi-
tive esports settings: A systematic review. Psychology
of Sport and Exercise, 51:101738.
Mauss, I. B. and Robinson, M. D. (2009). Measures of emo-
tion: A review. Cognition & Emotion, 23(2):209–237.
Nisbett, R. E. and Wilson, T. D. (1977). The halo effect: Ev-
idence for unconscious alteration of judgments. Jour-
nal of Personality and Social Psychology, 35(4):250–
256.
Pedraza-Ramirez, I., Musculus, L., Raab, M., and Laborde,
S. (2020). Setting the scientific stage for esports psy-
chology: a systematic review. International Review of
Sport and Exercise Psychology, 13(1).
Posada-Quintero, H. F., Florian, J. P., Orjuela-Ca
˜
n
´
on, A. D.,
and Chon, K. H. (2016). Highly sensitive index of
sympathetic activity based on time-frequency spectral
analysis of electrodermal activity. American Journal
of Physiology-Regulatory, Integrative and Compara-
tive Physiology, 311(3):R582–R591.
Russell, J. A. (1980). A circumplex model of affect. Journal
of Personality and Social Psychology, 39(6):1161–
1178.
Samara, A., Galway, L., Bond, R., and Wang, H. (2017).
Affective state detection via facial expression analysis
within a human computer interaction context. Journal
of Ambient Intelligence and Humanized Computing,
10(6):2175–2184.
Shaffer, F. and Ginsberg, J. P. (2017). An overview of heart
rate variability metrics and norms. Frontiers in Public
Health, 5.
Valenza, G., Citi, L., Saul, J. P., and Barbieri, R. (2018).
Measures of sympathetic and parasympathetic auto-
nomic outflow from heartbeat dynamics. Journal of
Applied Physiology, 125(1):19–39.
Van Gent, P., Farah, H., Van Nes, N., and Van Arem, B.
(2019). Heartpy: A novel heart rate algorithm for
the analysis of noisy signals. Transportation research
part F: traffic psychology and behaviour, 66:368–378.
Yaribeygi, H., Panahi, Y., Sahraei, H., Johnston, T. P., and
Sahebkar, A. (2017). The impact of stress on body
function: a review. EXCLI Journal; 16:Doc1057;
ISSN 1611-2156.
SUPPLEMENTARY MATERIAL
See Tables 5 and 6 in https://drive.google.com/file/d/
1zByOo59gS2x0cGhZLhY5akaVFhTGlAQi/view?u
sp=sharing.
The Stress Is Real: Physiological Measurement of League of Legends Players Experience During a Live Esports Event
205