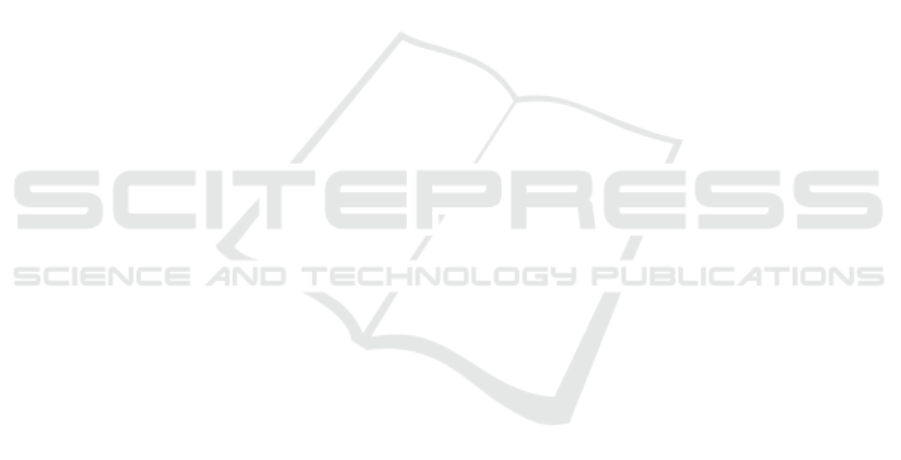
5.3 Summary of Findings for Each
Question
1. Regarding the first question, the results indicate
that integrating an AI system proves beneficial in
reducing cognitive overload.
2. Concerning the second question, our analysis re-
veals that a quadratic model with positive coeffi-
cients is most appropriate for our case.
3. The results from the third question indicate that
integrating AI into the workflow does not change
the quantity of tasks assigned to trainees. This
demonstrates that the inclusion of AI has no ad-
verse effects on the volume of operational training
tasks.
5.4 Discussion
Our experimental findings affirm the critical role
of AI integration in mitigating cognitive overload.
Nevertheless, addressing overload requires a multi-
dimensional approach that extends beyond AI facil-
itation to include flexibility, learning capabilities, and
unforeseen event management. Crucially, maintain-
ing comprehensive training for human pilots empha-
sizes the imperative of human oversight.
We have identified a quadratic model with posi-
tive coefficients as optimal for our experimental con-
text. Yet, real-world applications necessitate consid-
eration of environmental impacts, feasibility, and ben-
efits associated with downtime activities. Our algo-
rithm not only supports AI assistance but also strate-
gically manages the workload on trainees—essential
in training-centric scenarios. To bolster security and
maintain operational readiness, an AI-first strategy
is recommended, ensuring human operators are pre-
pared to intervene when AI limitations surface.
6 CONCLUSION
This paper studied the tasks scheduling of a Human-
AI collaborative drone-piloting mission. A novel task
scheduling algorithm integrating micro-breaks during
the mission was proposed to schedule necessary re-
covery time for human workers during their working
hours to deal with the human mental workload over-
load while while fulfilling the designated mission.
Experiments show that our method allows to integrate
AI in order to reduce the risk of overload while pre-
serving the training of human pilots. Regarding the
recovery model, results require to be tested with ob-
servation from real-life situations.
REFERENCES
Albulescu, P., Macsinga, I., Rusu, A., Sulea, C., Bodnaru,
A., and Tulbure, B. T. (2022). ”Give me a break!”
A systematic review and meta-analysis on the efficacy
of micro-breaks for increasing well-being and perfor-
mance. PLOS ONE, 17(8):e0272460.
Asadayoobi, N., Taghipour, S., and Jaber, M. (2023). Opti-
mising stochastic task allocation and scheduling plans
for mission workers subject to learning-forgetting,
fatigue-recovery, and stress-recovery effects. Expert
Systems with Applications, 229:120524.
Brazaitis, M. and Satas, A. (2023). Regular short-duration
breaks do not prevent mental fatigue and decline in
cognitive efficiency in healthy young men during an
office-like simulated mental working day: An EEG
study. International Journal of Psychophysiology,
188:33–46.
Caldwell, J. A., Mallis, M. M., Caldwell, J. L., Paul, M. A.,
Miller, J. C., and Neri, D. F. (2009). Fatigue Counter-
measures in Aviation. Aviation, Space, and Environ-
mental Medicine, 80(1):29–59.
Drenth, P. J. D., editor (1998). Organizational psychology.
Number 4 in Handbook of work and organizational
psychology / ed. by Pieter J. D. Drenth. Psychology
Press, Hove.
Hancock, G., Longo, L., Young, M., and Hancock, P.
(2021). MENTAL WORKLOAD. In Salvendy, G. and
Karwowski, W., editors, HANDBOOK OF HUMAN
FACTORS AND ERGONOMICS, pages 203–226. Wi-
ley, 1 edition.
Hoover, C. S., Ragsdale, J. M., and Ayres, T. B. (2022).
An experimental test of resource recovery from phys-
ical and relaxation work break activities. Stress and
Health, 38(3):477–489.
Jaber, M. Y. and Neumann, W. P. (2010). Modelling worker
fatigue and recovery in dual-resource constrained sys-
tems. Computers & Industrial Engineering, 59(1):75–
84.
Li, K., Xu, S., and Fu, H. (2020). Work-break schedul-
ing with real-time fatigue effect and recovery. Inter-
national Journal of Production Research, 58(3):689–
702.
Tucker, P. (2003). The impact of rest breaks upon acci-
dent risk, fatigue and performance: A review. Work &
Stress, 17(2):123–137.
Xie, B. and Salvendy, G. (2000). Prediction of Men-
tal Workload in Single and Multiple Tasks Envi-
ronments. International Journal of Cognitive Er-
gonomics, 4(3):213–242.
Zhang, M., Li, C., Shang, Y., Huang, H., Zhu, W., and Liu,
Y. (2022). A task scheduling model integrating micro-
breaks for optimisation of job-cycle time in human-
robot collaborative assembly cells. International Jour-
nal of Production Research, 60(15):4766–4777.
ICCAS 2024 - International Conference on Cognitive Aircraft Systems
102