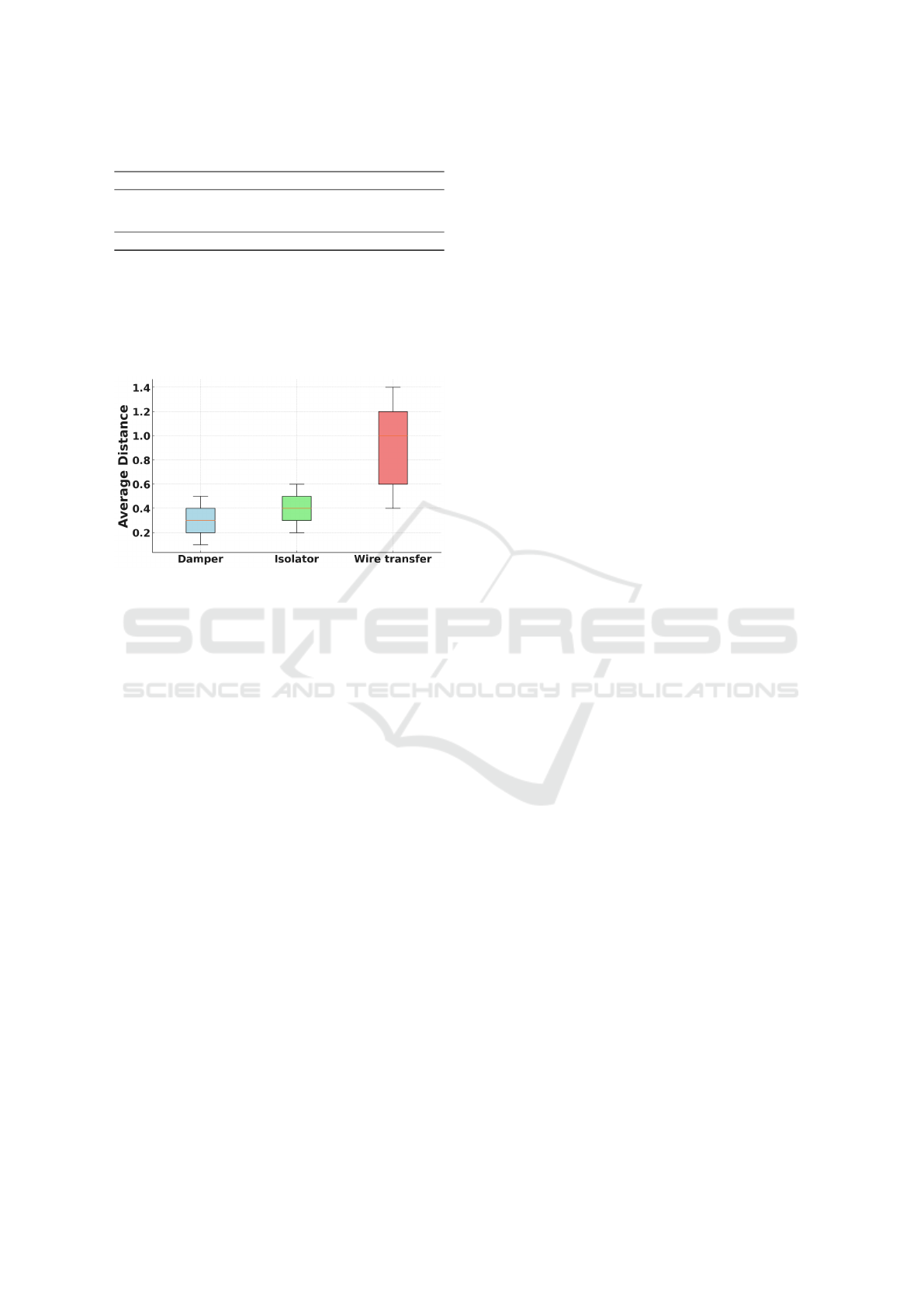
Table 7: Overall evaluation.
Recognitions Efficiency Mean A.distance Deviance
Damper 46 92.00% 0.44 0.07
Isolator 48 96.00% 0.61 0.14
Wire marker 50 100.00% 1.41 0.34
Mean 48 96.00% 0.82 0.18
The experimental analysis was summarized and
graphically presented using boxplots. These graphs
provide a visual summary that can help identify the
central tendency, variability, and symmetry of the
data, along with potential outliers, as shown in Figure
12.
Figure 12: Boxplot of average distances.
5 CONCLUSIONS
This paper presents a novel approach for detecting
and classifying elements along power lines using a
LiDAR sensor. In contrast to traditional methods that
process entire 3D point clouds, this method focuses
on analyzing a single two-dimensional (2D) slice of
an object, significantly reducing the data volume and
increasing the computational efficiency.
Object classification was achieved by calculating
the absolute differences between consecutive values
within a 2D slice of the LiDAR point cloud.
These differences were aggregated to create a
unique signature for each object, allowing effective
categorization. The results indicated that the kNN
classification system can introduce the capability of
power-line object recognition to a LaRa autonomous
inspection robot equipped with a LiDAR sensor,
achieving accurate identification of different classes
of objects.
ACKNOWLEDGEMENTS
The project is supported by the National Council for
Scientific and Technological Development (CNPq)
(process CNPq 407984/2022-4); the Fund for
Scientific and Technological Development (FNDCT);
the Ministry of Science, Technology and Innovations
(MCTI) of Brazil; the Araucaria Foundation; and the
General Superintendence of Science, Technology and
Higher Education (SETI).
REFERENCES
Alhassan, A. B., Zhang, X., Shen, H., and Xu, H.
(2020). Power transmission line inspection robots:
A review, trends and challenges for future research.
International Journal of Electrical Power & Energy
Systems, 118:105862.
Chen, C., Jin, A., Yang, B., Ma, R., Sun, S., Wang,
Z., Zong, Z., and Zhang, F. (2022). Dcpld-net:
A diffusion coupled convolution neural network for
real-time power transmission lines detection from
uav-borne lidar data. International Journal of Applied
Earth Observation and Geoinformation, 112:102960.
Chen, M., Tian, Y., Xing, S., Li, Z., Li, E., Liang, Z., and
Guo, R. (2021). Environment perception technologies
for power transmission line inspection robots. Journal
of Sensors, 2021(1):5559231.
Dem
ˇ
sar, J., Curk, T., Erjavec, A., Gorup,
ˇ
C., Ho
ˇ
cevar, T.,
Milutinovi
ˇ
c, M., Mo
ˇ
zina, M., Polajnar, M., Toplak,
M., Stari
ˇ
c, A., et al. (2013). Orange: data mining
toolbox in python. the Journal of machine Learning
research, 14(1):2349–2353.
Dem
ˇ
sar, J. and Zupan, B. (2012). Orange: Data mining
fruitful and fun. Inf. Dru
ˇ
zba IS, 6:1–486.
Jenssen, R., Roverso, D., et al. (2018). Automatic
autonomous vision-based power line inspection: A
review of current status and the potential role of deep
learning. International Journal of Electrical Power &
Energy Systems, 99:107–120.
Korki, M., Shankar, N. D., Shah, R. N., Waseem, S. M.,
and Hodges, S. (2019). Automatic fault detection
of power lines using unmanned aerial vehicle (uav).
In 2019 1st International Conference on Unmanned
Vehicle Systems-Oman (UVS), pages 1–6. IEEE.
Paneque, J., Valseca, V., Mart
´
ınez-de Dios, J., and Ollero,
A. (2022). Autonomous reactive lidar-based mapping
for powerline inspection. In 2022 International
Conference on Unmanned Aircraft Systems (ICUAS),
pages 962–971. IEEE.
Qin, X., Wu, G., Lei, J., Fan, F., Ye, X., and Mei, Q.
(2018). A novel method of autonomous inspection for
transmission line based on cable inspection robot lidar
data. Sensors, 18(2):596.
Rohmer, E., Singh, S. P., and Freese, M. (2013). V-rep:
A versatile and scalable robot simulation framework.
In 2013 IEEE/RSJ international conference on
intelligent robots and systems, pages 1321–1326.
IEEE.
Yang, L., Fan, J., Liu, Y., Li, E., Peng, J., and
Liang, Z. (2020). A review on state-of-the-art
power line inspection techniques. IEEE
LiDAR-Based Object Recognition for Robotic Inspection of Power Lines
203